Nitrogen+Syngas 370 Mar-Apr 2021
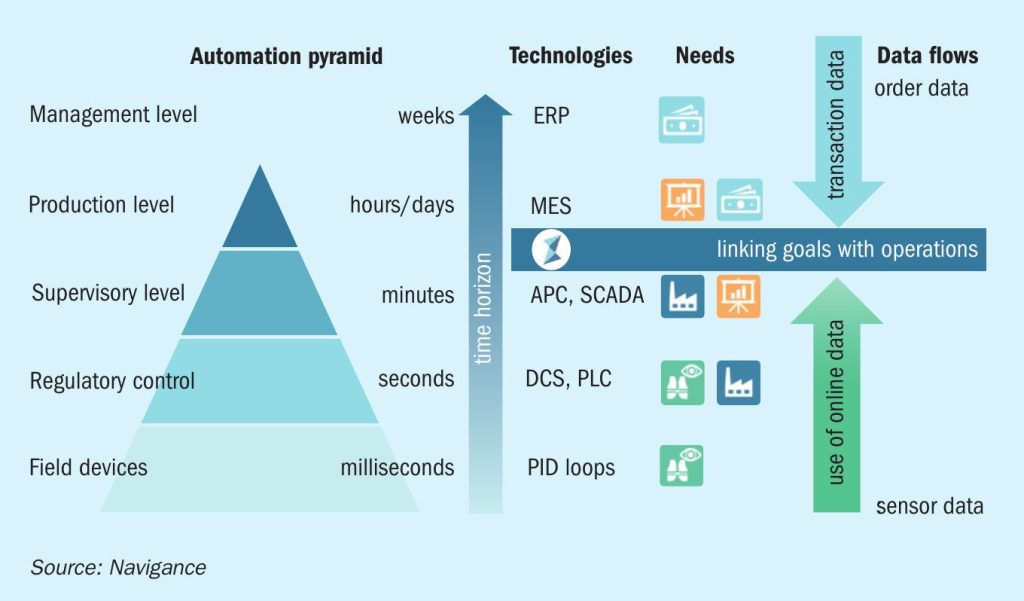
31 March 2021
Opportunities for data-driven analytics
As industry trends add pressure to optimise processes, new digital technologies for roundthe-clock, data-driven decision-making can help plants boost production efficiency without time-consuming manual analysis or large-scale investment. Here, Dr S. Werner of Navigance explores their potential and shares the difference they are already making.
When Navigance conducted a recent study1 of over 40 experts working in various chemical manufacturing roles, what they revealed about the key priorities in the sector and the role digital technologies can play in addressing them was fairly unanimous.
Most saw the potential in digitalising aspects of their operations and making better use of the data being collected across it. Many recognised that artificial intelligence and other advanced analytics technologies could help to unlock operational efficiencies by enabling more data-driven decision-making.
However, the extent to which they felt ready to take the steps needed to achieve that themselves varied greatly. This depended largely on the ‘demographics’ of their company: size, level of digital maturity, and how effectively they were dealing with the priorities most said came ahead of efficiency.
Most crucial was the need to ensure safety and minimise time lost to accidents. Next was keeping plant availability high, preventing downtime, production losses and their potentially high costs. Only once safety and availability were being managed at consistently high levels would plants turn their focus to increasing the efficiency of their processes.
Increasingly, though, manufacturers may not have the comfort of waiting “until the time is right” to optimise their processes. Trends gathering pace in the sector for some time look set to make optimisation a necessity rather than a nice-to-have.
For one thing, the effects of economic downturn in recent years mean the ability to do more with less – from people to energy to raw materials – has never been more pressing. The Covid-19 pandemic has exacerbated this trend, with over 32,000 production jobs lost in the sector in the 12 months from September 2019 alone2 .
Chemical producers are also struggling to replace lost experience as an ageing workforce retires, taking with them skills and a problem-solving intuition that are hard to replicate quickly enough in new recruits.
What’s more, the coming years will see a keener focus on the sustainability of chemical processes. Rather than simply offsetting the impact of their operations, chemical manufacturers must radically rethink their ways of working and make much more efficient use of resources and materials.
The data dilemma
For those prepared to act now, digital technologies, big data and the expertise to harness them effectively can help to overcome these challenges and overtake those who continue to wrestle with them.
However, just 4% of people Bain & Company surveyed3 from over 400 companies with $1 billion-plus turnovers said they had the resources needed to draw useful insights from and act on their data. Indeed, Navigance’s own survey found the greatest barrier to digital adoption was plants’ ability to spare time or people for complex manual data analysis or to implement technologies that would take that burden off their shoulders.
The good news is that it is possible to use plants’ data to optimise both availability and process efficiency, without tying up in-house resources, big investments or even long periods before seeing the benefits. It can be done in a way that improves both the sustainability and profitability of operations while reducing production costs. And it builds on technologies and methodologies that are already in place.
The opportunity in chemicals
The chemical sector is in a good position to reap the benefits of data-driven decision-making.
Use of control systems has been widespread since the 1960s, and a typical plant now has thousands of sensors collecting huge volumes of process and performance data. This data is used to help control the plant, generate reporting and may also be stored long term to support troubleshooting and analytics.
Much of this work is done retrospectively and at irregular intervals, though, rather than routinely and in real time, when it can make the greatest potential difference. Also, significant amounts of that work are done in manual workflows, which take time and resources and are prone to errors. It also depends on plants being able to spare personnel for extended periods to conduct the necessary analysis. A challenge at the best of times, but even more so given current economic conditions.
Decisions based on this data, though grounded in many years of industry experience, are usually made using intuition and a ‘gut feeling’ more than an irrefutable reading of the facts. A skill hard to teach to a new generation who will carry the baton forward.
In addition, the sheer volume of data being analysed manually makes it highly likely that important patterns and potential will be missed; they simply can’t all be spotted by human eyes. And if the data used is only ever historical, plant teams will always be on the back foot.
The opportunity digitalisation presents is to integrate continuous process data into operational decision-making. Moving from retrospective analysis and troubleshooting to a comprehensive and near real-time view of how the operation is running to inform confident, effective action.
Doing so can help to close the gap between commercial and operational goals.
The automation pyramid in Fig. 1 shows layers of decision-making from transactional data-based ERP system orders down to the stabilisation of individual measurements in PID loops. Each layer addresses needs, such as safety and availability in the bottom up to margin and efficiency above.
Navigance helps operational teams deliver on their commercial goals by providing expertise that is hard to acquire in house, and an approach that builds on and surpasses established methods of understanding and improving how plants work.
The challenges of modelling
Models are a vital tool for the chemical sector and engineering sciences in general. In essence, they are mathematical representations of the relationships between variables in (real) systems. In the chemical industry or continuous manufacturing in general, they can describe and help operators understand the behaviour of a plant or process.
Models based on first principles have been used for decades to help design new plants or revamp, debottleneck, and optimise operations in existing ones. But modelling real-world scenarios in today’s highly complex operations can be very challenging.
First principle models are based on established science and often explicit relationships in a particular unit operation in a chemical process. They rely heavily on recognised correlations and a fundamental understanding of underlying ‘ab initio’ physiochemical phenomena such as kinetics or chemical equilibria or pure substances. However, on closer inspection that understanding is not really complete at all.
In many cases, a flow-sheet model combining first principles with correlations such as heat transfer, porosity and vapour pressure can be constructed to describe the full extent of the plant. Even getting vapour-liquid equilibria (VLE) data for a non-ideal multi-component mixture can be limited by available correlations or validity ranges. Understanding the pressure drop of irregular catalyst particles in a packed bed relies on estimation rather than on exact calculation. It can take many years of work, lots of resources and substantial investment to address these points.
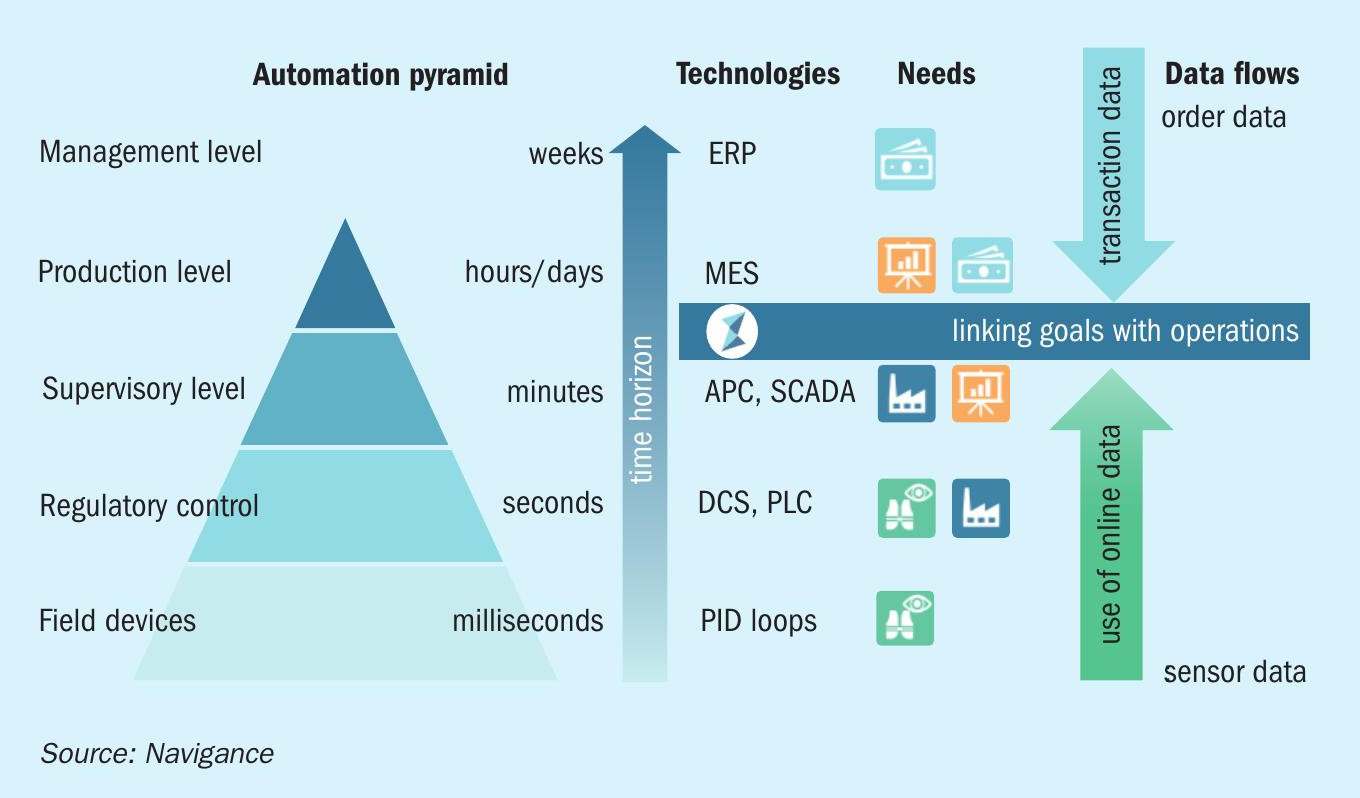
In addition, observed ‘real world’ information is usually needed to “fill in the blanks” where parameters are unknown or missing. But getting it becomes increasingly difficult as the complexity and number of parameters involved increases.
In an attempt to describe the phenomena seen in real-world systems sufficiently, it is easy to end up with thousands of model equations and partial differential equations that cannot be solved analytically.
This is when “fiddle factors” come into play; widely established parameters used to fit model outputs to the observed data. This includes “activity factors” to correct for deviations in catalytic activity, or “shape factors” to account for inaccuracies in the pressure drop.
These factors compensate for unknowns or uncertainties in the models but can result in physically unrealistic situations in parts of the simulation and leave significant inaccuracies in the final result.
Describing a plant to its full extent can mean stacking many different first principle based models, and all their potential inaccuracies, on top of each other. Bridging this gap between model and the day-to-day reality in plants, takes expert care and a fresh approach that builds on and enhances what has gone before.
Data-driven hybrid models
Hybrid models can help overcome these gaps by harnessing the large data volumes already available from process control systems and data historians in place across the industry.
A hybrid approach provides flexibility to not only present a real-world view of plants but also adapt to changing conditions within them. It can also avoid the trap of “spurious correlations,” which are often the result of a purely statistical approach and bring little value in optimisation4 .
The benefits of using data-driven neural networks and hybrid models to dynamically model chemical systems have been recognised for some time – described in academic contexts as much as 20 years ago5 . Applying the theory in practice, however, involves obstacles it takes expert care to overcome.
As we have heard, the data required usually exists, but it needs preparing and processing in order to be useful. This can prove both time consuming and expensive if done manually. Data scientists spend up to 80% of their time preparing data for analysis, with the vast majority of that spent cleaning and organising the data6 . Fortunately, the days of needing to do so are fading.
Progress in making access to data easier, combined with the decreased cost of computation and data storage, and the rapid advances seen in machine learning technology, now presents the opportunity to create data-driven models that build on automatic and continuous flows of high-quality, cleaned data for advanced and automatic analysis.
The Navigance approach
Over recent years, Navigance has developed and demonstrated the successful use of next-generation, data-driven hybrid process models that adapt easily to both a plant’s historic and real-time data, generating reliable, prescriptive advice teams can use to continuously optimise their operations.
These models are still based on established first principle techniques, physicochemical relationships and engineering principles. The difference is they are augmented with neural networks adapted to each plant’s specific setup and circumstances, and machine learning algorithms that provide advanced and almost instantaneous analysis of the data.
In contrast to rigid first principle models, Navigance hybrid models learn intuitively and respond quickly to changing conditions and factors, from varying load scenarios to deactivating catalysts. And they work regardless of the plant’s technologies and setup.
In the example shown in Fig. 2, Navigance models were tailored to suit a plant’s specific process based on an initial data set as it introduced a new catalyst. It shows the quick adaptation of a machine learning model to a new situation in a plant. After a catalyst change the models give high-quality predictions quickly after the initial, unsteady start-up phase.
Real benefits, rapid results
With Navigance base models ready to adapt to each plant’s particular setup and circumstances, they can be deployed quickly, and realise process optimisation potential faster.
Navigance works with producers to implement a detailed optimisation strategy tailored to their most pressing operational goals and any constraints and limitations. This includes identifying appropriate control variables with which to steer their operations and increase process efficiency.
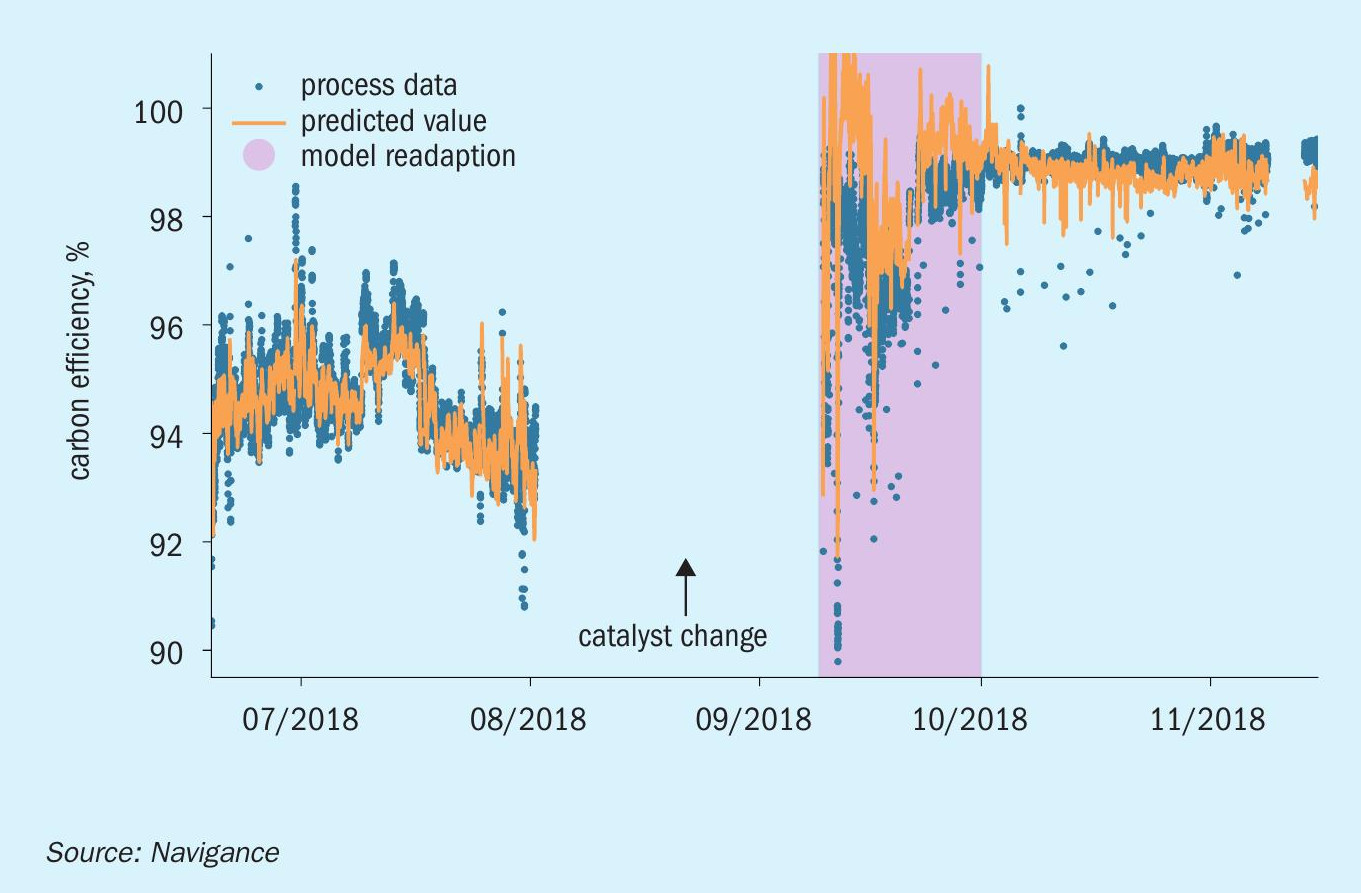
In the usage phase, a continuous stream of plant data is uploaded securely to the Navigance cloud. There it is automatically cleaned and prepared to remove all outliers, noise and discrepancies using adaptive filters, pattern-matching heuristics and other additional classification techniques.
This cleaned data provides the foundation for automatic forecasting and real-time, prescriptive recommendations based on a complete and current view of the most pertinent information. Plant personnel can access this advice in an online dashboard and act on it with confidence to refine process control variables, hit their optimisation goals and critical KPIs, and so deliver on the plant’s commercial objectives.
In this way, producers can take data-driven decision-making to new levels. Moving away from hours spent analysing and cleansing ‘descriptive statistics’ and beyond predictive forecasting to a future of prescriptive advice for round-the-clock optimisation (Fig. 3).
It’s a future that producers of chemicals other than methanol are already seeing rapid benefits from today, too.
In formaldehyde production, for example, where methanol equates to over 90% of total production costs, the amount of product it yields is a vital KPI to measure and optimise.
Within just three months of introducing the Navigance Optimization Engine at one of its plants, one large formaldehyde producer with multiple units found the models and recommendations helped increase yield by 0.8 mol-% above the long-term average typically seen.
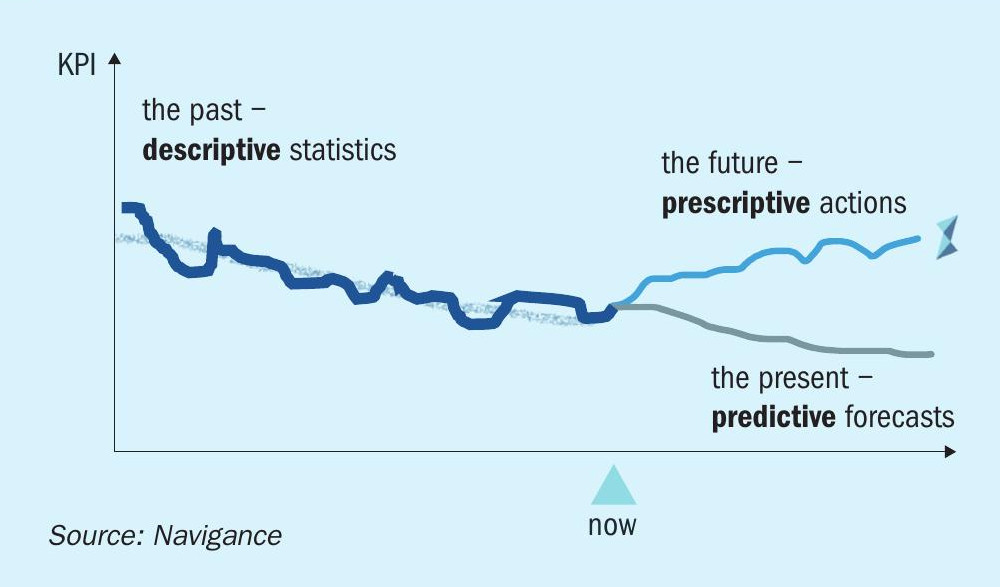
Assessing optimisation potential
A common question producers ask when deciding if and when to introduce data-driven optimisation is: “how much benefit can we expect in our plant?” One method Navigance applies to assess a plant’s optimisation potential is the Best Demonstrated Practice (BDP) approach7 .
The underlying idea is that plants do not always run at their optimal level, so exhibit efficiency fluctuations in the trends of their major KPIs. In one example from a methanol plant, the KPI in question – the yield – can be seen fluctuating over time (Fig. 4).
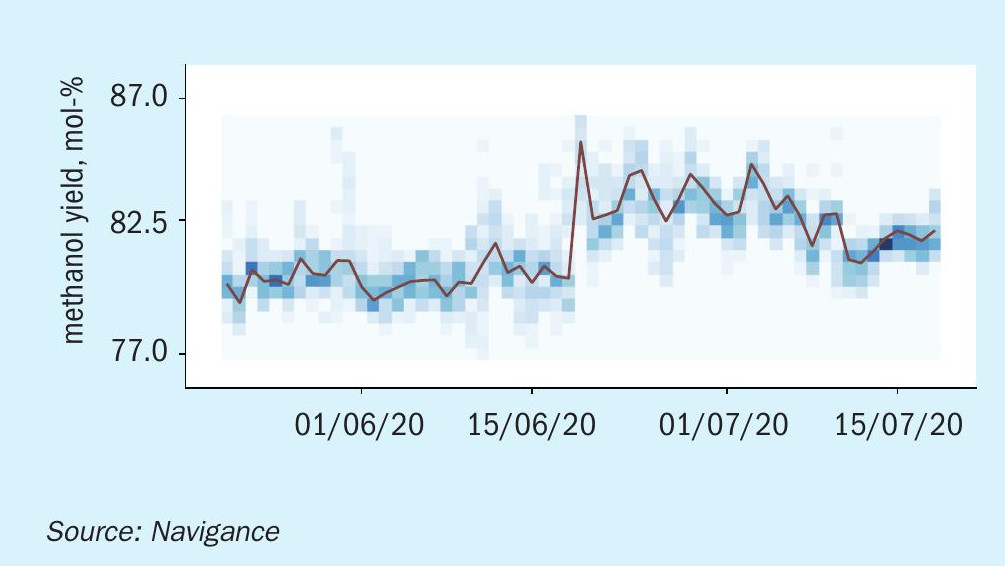
By exploring how well the plant has proven to perform in a given week, then looking at hourly performance, it can be assumed that continuous optimisation and fine tuning of the process in a given week can lift the worst-performing tercile up to the average performance demonstrated in the same week. In the same example (Fig. 5), the weekly average optimisation potential ranged from a 0.2% to a 0.4% increase in yield.
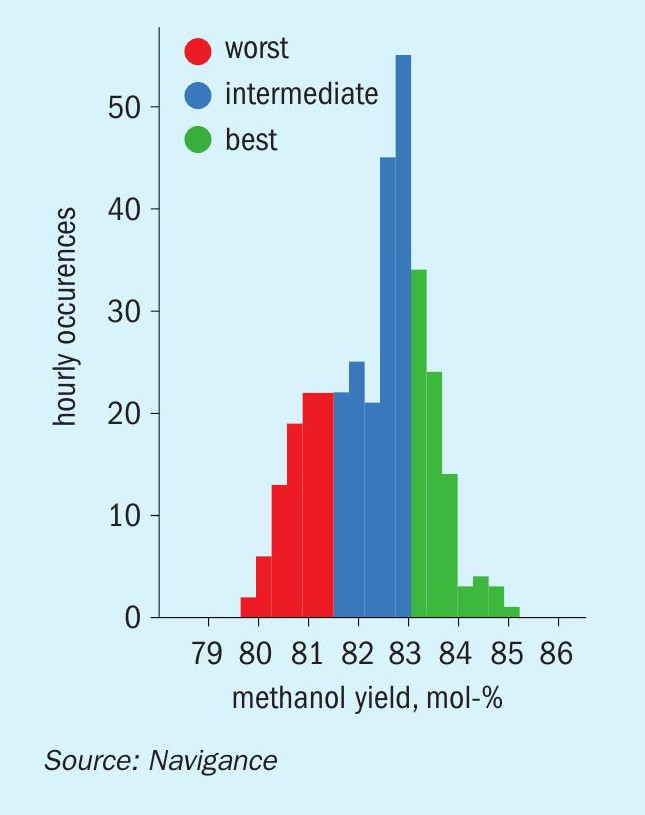
This is only one aspect that is explored. Further optimisation potential can be found in cross correlations between variables and KPIs. Assessments of high-resolution plant data commonly reveal optimisation potential of 1-3% for a methanol or ammonia plant.
Plant monitoring: an additional benefit
Navigance hybrid models offer producers other optimisation potential too. A by-product of the data cleaning and preparation process is the ability to detect anomalies, unusual behaviour and potential issues early. This forms the basis of a solution called the Navigance Plant Monitor, which combines 24/7 monitoring of the plant with predictive alerting.
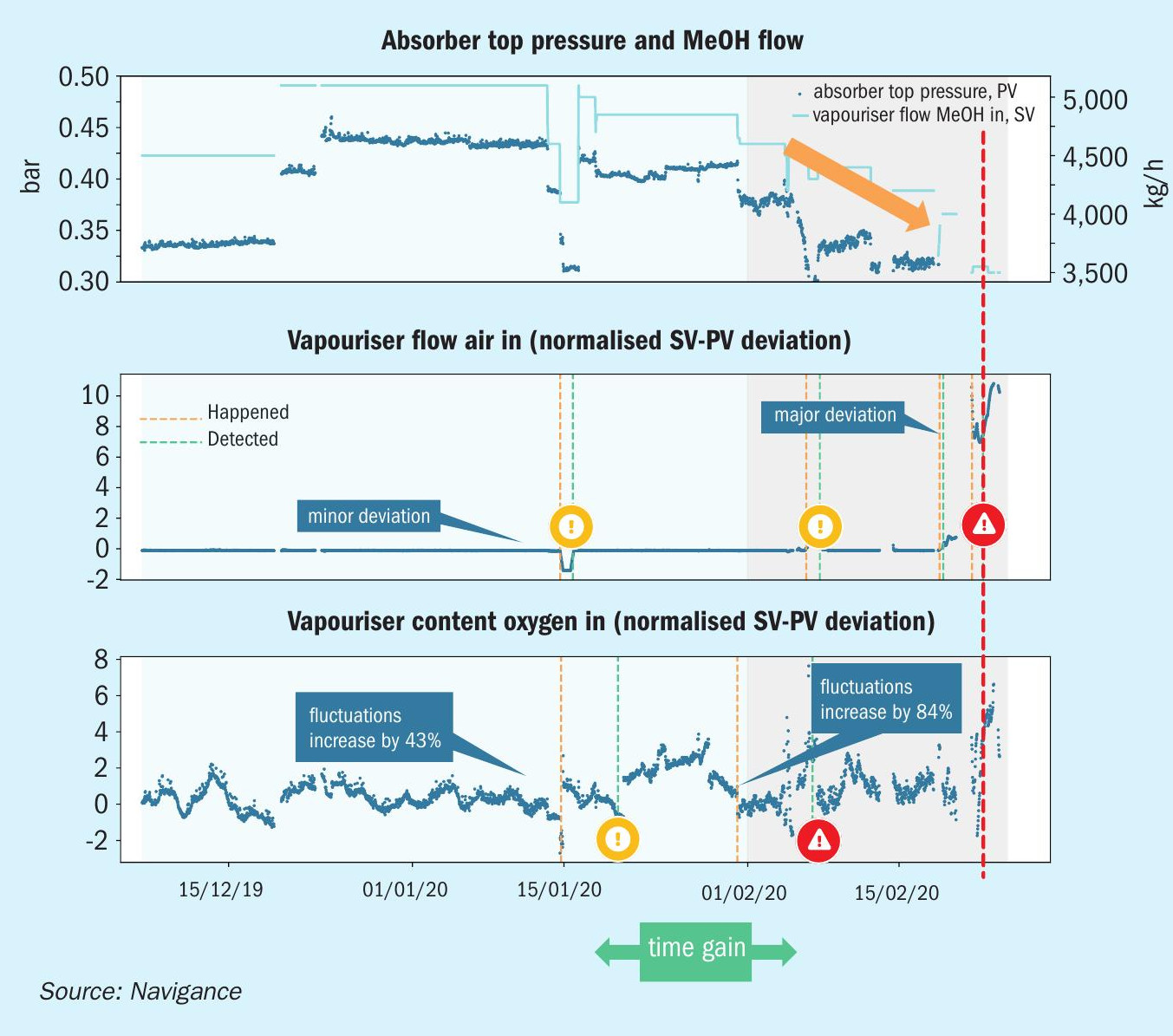
In the example shown here (Fig. 6), unusual behaviour detected by multiple separate algorithms highlighted the critical failure of a valve more than two weeks before it happened. This allowed maintenance teams to take proactive and focused action.
Conclusion
For chemical producers yet to take substantial steps into a digitalised future, or those eager to accelerate the process but struggling to spare the time needed to do so, these technologies offer a real opportunity.
Navigance offers solutions to help address both the industry’s most critical priorities and the accelerating trends that look set to shape how it operates in the years to come.
Ready to tailor to each plant’s specific setup or existing technology mix, and to implement and start using quickly without huge expense, these solutions are backed by the expertise in both chemical processes and data science needed to deploy them for maximum effectiveness.
References and notes