Nitrogen+Syngas 385 Sept-Oct 2023
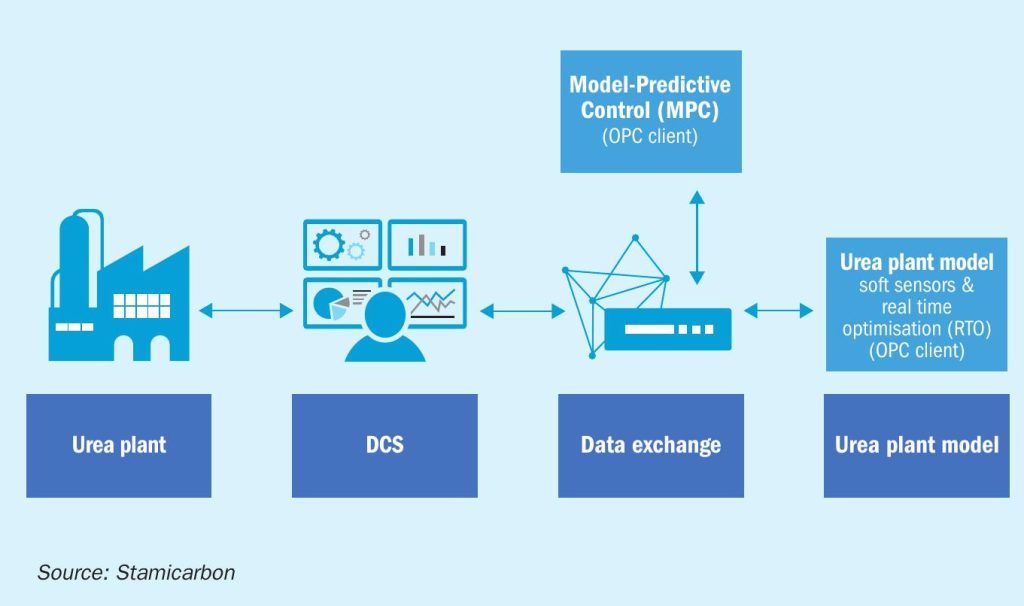
30 September 2023
Digital tools reshaping urea plant operations
DIGITALISATION
Digital tools reshaping urea plant operations
With the growing global demand for food and the rise in ecological challenges, there’s a pressing need for a more sustainable and environmentally-friendly approach to fertilizer production. Achieving a sustainable increase in plant load and operational margins through improved operations is a demanding task. Luc Dieltjens and Ali El Sibai of Stamicarbon discuss how a plant can effectively address these challenges with digital tools to optimise the process.
Model-based process optimiser: A powerful digital tool
The model-based process optimiser is undoubtedly one of the digital tools that can substantially enhance plant operations and consequently refine the overall performance of chemical processes. By relying on a robust model and by utilising an efficient solver, the optimiser computes the optimal operating conditions with respect to a defined objective function (e.g., maximising productivity, minimising energy consumption) and under the existing process constraints/disturbances. Clearly, this demands, as a first step, a comprehensive assessment of the plant’s condition, process control, and dynamics.
Stamicarbon, nitrogen technology licensor of MAIRE Group, combined these “standard” aspects of a process optimiser with its own process control solutions. The result is a unique process optimiser, Stami Digital Process Optimizer, that acts like an autopilot for the urea plant and can therefore consistently achieve an efficiency boost ranging from 2–5%, contingent on local conditions.
Stami Digital Process Optimizer: The principle
All urea stripping plants are characterised by a high degree of interaction among the process variables. Any change at the back end will affect the synthesis and vice versa. These are typical control characteristics for plants incorporating multiple recycles. The dehydration of ammonium carbamate to urea and water is slow, necessitating large retention times in the reactor, typically around one hour. This reactor hold-up is referred to as ‘pure dead time’ in the process. Dead time is the property of a physical system whereby the response to an applied disturbance is delayed in its effect.
The Stami Digital Process Optimizer combines the advantages of a multivariable, model-predictive control (MPC) system with the non-linear optimisation capability of a urea process modelling tool. It uses a model of the urea process to predict the behaviour of the plant several hours into the future. Given the safety and operating limitations, as well as the quality specifications of the process, the system calculates the optimal adjustments to be made. It then implements these adjustments by sending minor changes to the setpoints of key process variables in the distributed control system (DCS). This ensures urea producers benefit from enhanced operational reliability, guaranteeing continuous and consistently optimal operation.
The basic principle of an MPC system is to utilise existing knowledge of the process transfer characteristics. By understanding the relations between manipulated variables, measured disturbances, and controlled variables, the process can be continuously driven to the desired operating conditions. Using data-driven dynamic models derived from step-testing, the MPC controller stabilises the process operation and pushes it as close as possible to process limits, such as synthesis pressure.
Stami Digital Process Optimizer builds upon the process monitor that calculates key performance indicators (KPIs) such as plant load, ammonia emissions, energy consumption, and soft sensor key variables. These soft-sensor key variables provide additional process information which is not measured or cannot be measured, such as stripper tube load, stripper efficiency, and ammonia emissions. These soft sensor key variables have been included in the MPC design as control objectives.
The process optimiser directly anticipates the effects that disturbances have on the process outputs that are controlled as soon as these disturbances are observed. It then steers the process to new operating conditions. Conversely, a traditional feedback control system like a PID controller first needs to observe the effects of disturbances at the controlled process outputs before it can start counteracting these disturbances.
The architecture of the process optimiser system is shown in Fig. 1. Without this system, corrective actions are left to the discretion of the operators. The process optimiser automates operator handling and drives the urea plant to an optimised operating point by integrating real-time optimisation (RTO) and MPC.
The plant model calculates not only the KPIs and soft sensor key variables but also the real-time optimiser values. Optimal conditions for the process at a steady state are determined by the RTO, while the trajectory to be followed, as predicted using a linear dynamic model obtained through a plant step test, is managed by the MPC. Given the typical complexity (high degree of interactions and recycles) of a urea plant, the MPC is an invaluable tool to achieve the optimal, stable operating point efficiently.
Once the desired stability is reached, the MPC will be used to maximise the CO2 flow without breaching any operating constraints, ensuring the highest possible production capacity at all times.
Customer experiences
A process optimiser project has been executed on a urea plant with Stamicarbon’s LAUNCH MELT™ pool reactor design, which currently operates at a capacity of 1,400 t/d. The customer sought opportunities to enhance the urea plant’s operation without significant equipment modifications. The process optimiser was chosen as the optimisation tool. This urea plant is intricately integrated with two melamine plants and an ammonium nitrate plant, adding layers of complexity and challenges to the project. The project provided numerous insights, lessons, and best practices beneficial to all involved.
Current operation is not always optimal
The first lesson learned was that the accuracy of a plant model depends on how well it’s constructed. Given the urea plant’s expansive operating window, the model had to be robustly programmed to encompass all operating scenarios, using historical data as a basis. At certain junctures, the model’s predictions and trends diverged from the customer’s established experiences and practices. This led to in-depth discussions between the process engineers of the customer and Stamicarbon. Through these deliberations, it became evident that the urea plant wasn’t being operated optimally. The insights from these discussions enabled the customer to enhance urea production.
Implementation can take time
The implementation phase of this project took longer than expected due to several reasons. Firstly, differing opinions among the process engineers regarding optimal operation took time to reconcile. Secondly, some critical instruments weren’t functioning correctly, which impacted the implementation of the control strategy in the MPC controller. Lastly, for the step tests to be carried out, the plant needed to operate at nominal conditions. Coordinating the right resources, ensuring their availability, and aligning with the necessary plant conditions proved to be more complex than anticipated.
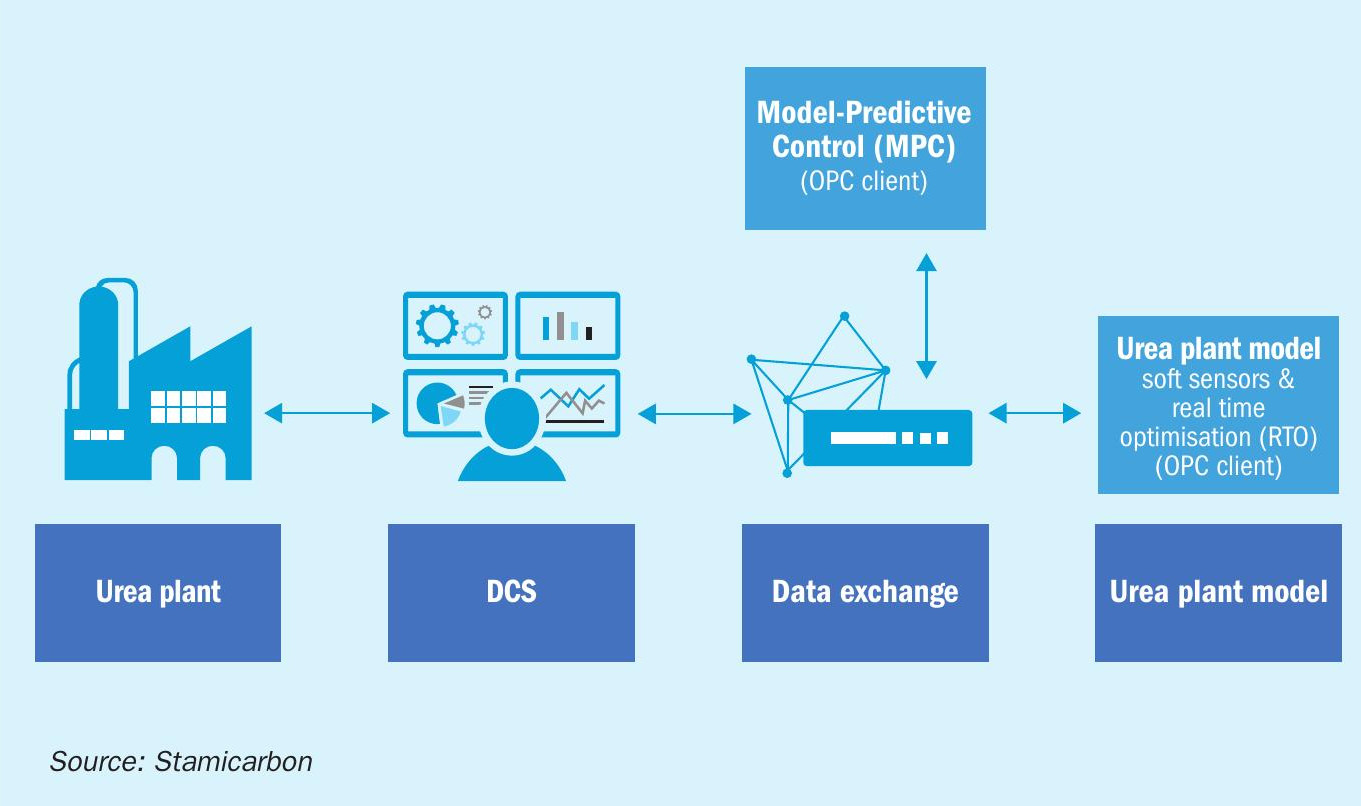
Results
The Stami Digital Process Optimizer calculates the ideal N/C ratio in the pool reactor, which the MPC then uses for anticipation. Because of an improved yield in the reactor, the overall production capacity rises. Additionally, there are gains from reducing variations in the N/C ratio when the optimiser is active (see Fig. 2). The stripper tube load, continuously calculated by the plant model, has the lowest specific energy consumption at its maximum. This tube load is set as a constraint in the MPC, meaning it shouldn’t exceed a certain limit. The MPC directs it towards this maximum.
An optimisation goal might be to maximise load or, considering feedstock constraints, to minimise energy use or some other objective function. Typical benefits of the process optimiser include a 1-5% rise in production, a 1-3% drop in specific energy consumption, an increased on-stream factor, and reduced manual oversight due to fewer interventions by operators. For the afore-mentioned project, the Stami Digital Process Optimizer achieved a 4% production boost and a notable reduction in energy costs. The results from deploying the Stami Digital Process Optimizer surpassed expectations in terms of both plant performance and knowledge acquisition.
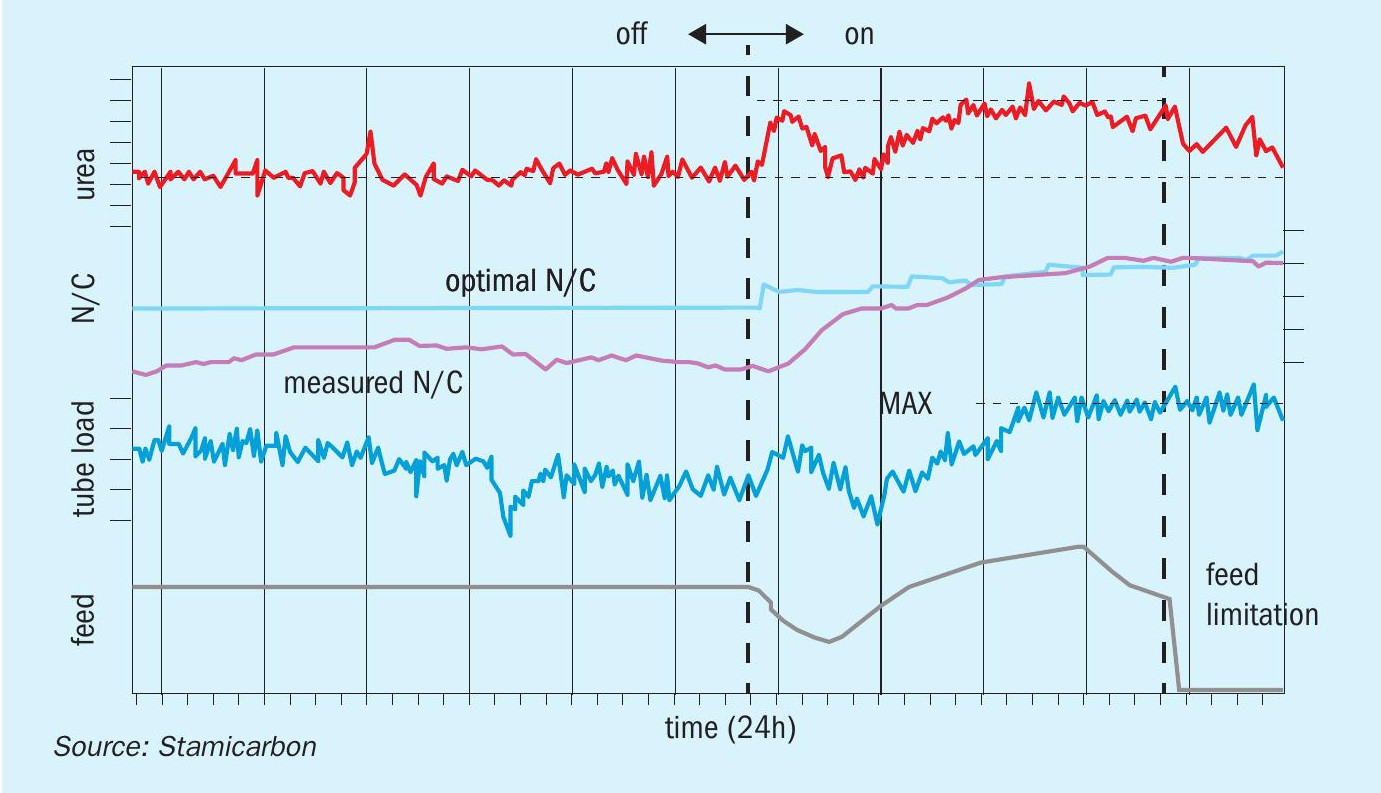
The process model becomes especially powerful for plant optimisation when paired with an MPC. Implementing the Stami Digital Process Optimizer requires no hardware modifications, nor does it necessitate plant shutdowns – it’s solely a software extension. To ensure data security Stamicarbon has been certified in the information security standard ISO 27001.