Nitrogen+Syngas 365 May-Jun 2020
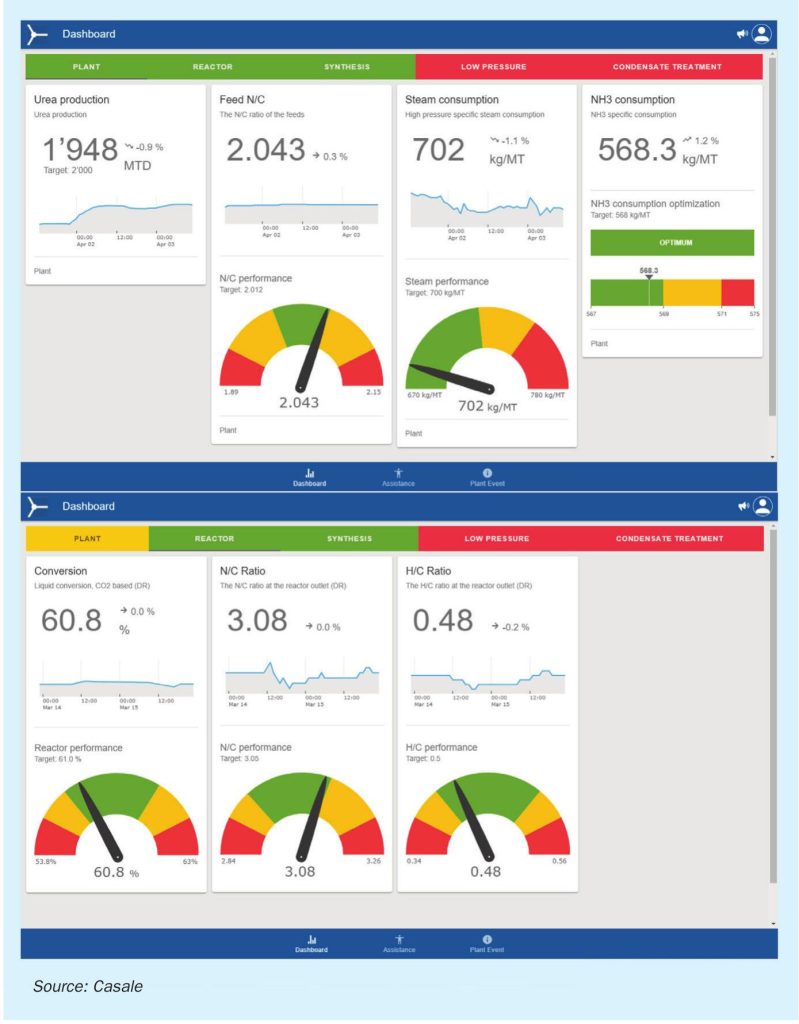
31 May 2020
The growing importance of technical services
TECHNICAL SERVICES AND DIGITALISATION SOLUTIONS
The growing importance of technical services
As existing facilities grow older, service requirements increase. Equipment needs to be low maintenance and back in operation quickly after service and repair. Technology licensors are expanding their technical services with new digital tools, using digitalised expertise with real time insights and data driven analytics to boost chemical production and ensure that equipment operates reliably and efficiently, while maintaining product quality.
CASALE
Casale’s digitalised expertise at customer service
The operation of chemical plants is a highly complex task due to the intricate interaction between chemicals, reactions, flow dynamics, etc. and from the need to manipulate a large set of variables to reach production targets, while maintaining optimum efficiency. Plant performance and its associated operating costs are impacted by both external and internal factors, for example, changes in the composition of the raw materials, weather conditions, as well as the experience and expertise of the operators. Plant performance is measured in terms of key performance indicators, including the overall production, specific energy consumption, plant stability, emissions, etc.
The close relationship between process operation and mechanical reliability adds to the complex operation of chemical plants. Often, plants are highly stressed by the operating conditions, which are kept close to their limits to maintain profits. This is particularly true for old plants whose mechanical reliability and related performance must be carefully monitored. Therefore, it is of utmost importance to develop maintenance programs aimed at improving reliability. The operational data becomes a fundamental part of a more extensive analysis that not only supports the diagnostics of failures but also helps to understand the root causes during mechanical inspections.
Chemical plants generate a huge amount of data on a daily basis. The data is mostly used for DCS visualisation and archival purposes, and for helping field operations and control. Unfortunately, due to the lack of resources and time, prominent trends and data correlations are seldom analysed thoroughly, leading to suboptimal operation. Furthermore, in critical scenarios, expert advice is normally needed, if not mandatory.
To help plant operations, support maintenance and improve mechanical reliability, Casale has created a portfolio of digital products that takes full advantage of the existing plant data infrastructure:
- Casale Remote Engineering Service (CARES);
- Casale Operator Training System (OTS);
- Casale Model Predictive Control (MPC);
- Casale smart instrumentation.
Casale Remote Engineering Service (CARES)
CARES is a remote engineering service which provides assistance to plant operators and managers, supporting the achievement of their daily operational targets, improving process reliability and supporting mechanical inspections.
At the core of remote engineering services, Casale uses an automatic data reconciliation algorithm to convert measured data from the plant into physically-consistent values that respect global and local mass and energy conservation around units of operation, sections of the plant or the whole plant. An important feature to note is that the data reconciliation tool uses the same Casale proprietary models and thermodynamic packages regularly used for designing the plants: a critical feature that ensures the highest possible fidelity of the service.
The data reconciliation is much more than a process simulation, it is a tailor-made process model built up to reveal the real time performance of the plant, including full information of the critical process streams, which cannot be directly measured in the field, e.g. the full composition at the reactor outlet. Thus, calculated key performance indicators (KPIs) based on reconciled data offer great value to clients, providing an effective way to identify critical aspects of the plant operation and their temporal behaviour.
Data reconciliation also allows the identification of faulty readings and anomalies in the field instrumentation used to guide the process operation. Thanks to the power of the data reconciliation, abnormal deviations among reconciled quantities and field measurements are promptly detected and thus used to alert critical conditions into the plant. Essentially the identification of erratic readings of the instrumentation may suggest the underperformance of a plant section or the drift of parameters towards critical operating conditions and constraints.
For instance, in the case of urea plants, CARES provides value not only through the KPIs of the critical HP synthesis equipment, but also alerts the operator of critical conditions which can impair the reliability and equipment life. The example of the HP stripper is typical: specific KPIs monitor the equipment performance such as the NH3 stripping efficiency providing guidance for process optimisation, while at the same time the stripper temperatures are kept under strict surveillance to alert the attainment of critical thresholds for corrosion or anomalies in the reading of the temperature transmitter which may represents a risk for equipment integrity.
Moreover, the operating conditions of such critical equipment, such as the temperature and inlet/oulet composition of the process solution, provide valuable information used by CARES to complement mechanical inspections and the diagnosis of findings by supporting pro-active maintenance programs.
How does CARES help clients?
Using a combination of advanced analytics and expert advice provided by the customer care team, plant behaviour is analysed, focusing on the aspects that are most relevant to the client:
- Boost plant productivity: CARES provides continuous support to utilise the plant capabilities at its best and to achieve maximum production.
- Improve plant efficiency: CARES provides guidance for reducing emissions, energy consumption and to achieve plant stability.
- Troubleshooting: CARES offers support in identifying the root cause of plant upsets and unexpected shutdowns, providing solutions to improve reliability and increase the on-stream factor.
- Improve understanding and confidence in plant operation: Take the right action at the right moment. Feel confident and conscious of the plant behaviour. This is not an easy task. CARES provides valuable advice which allows improved knowledge and confidence of the plant operation and production process.
- Identify plant limitations: Identify equipment bottlenecks and operating procedures that are hindering plant performance.
- Supporting pro-active maintenance and inspection programs.
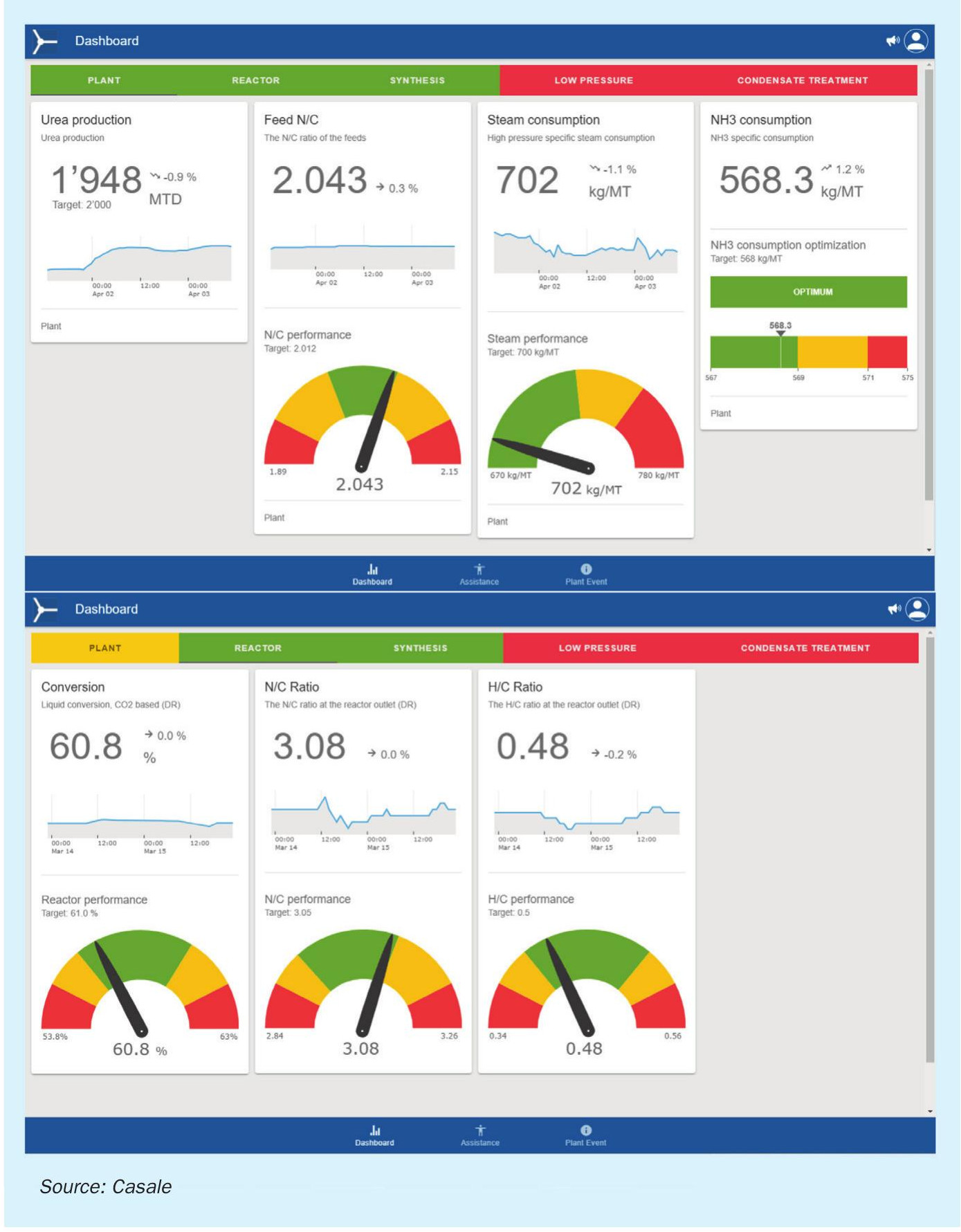
Expert advice is communicated to clients via a web dashboard available anywhere, anytime via a secure web page. The aim of the web dashboard is to provide a clear view of the plant and equipment performance using simple visual indicators (widgets) for the key performance indicators. The dashboard is not limited to displaying current values of the indicators but can also be used for trending and performance analysis: the user can inspect the timeline of the operation by selecting any date in the main interface window.
The dashboard service is completely customised according to customer needs. It is provided with a chat interface to maintain a direct link with the Casale customer care team and is complemented by periodic conference calls and written reports. The customer care team is also ready to support clients when problems occur. It is like having a Casale expert on site every day. Fig. 1 shows screenshots (of a section) of the CARES dashboard and related KPIs.
Benefits
Clients that use CARES have benefitted from the following: l Direct operational advice and suggestions from the customer care team based on the behaviour of KPIs. Casale team experts comprise a broad spectrum of competences ranging from process to mechanical and instrumentation engineering. l Critical insights of asset performance, leveraging Casale proprietary models that enable equipment parameters to be calculated that cannot be directly measured (e.g. catalyst health, catalyst activity, conversion efficiency, heat exchanger performance). l Analysis of a full set of reconciled values for the different sections of the plant. Information that can be easily used to tune or replace sensors that consistently exhibit large discrepancies between measured and reconciled values.
- Visualisation of performance and alert indicators of single units and plant sections by means of custom-made dashboards. Information that could be used by plant operators to reduce downtime and optimise equipment maintenance.
- Detailed reports summarising all the above and highlighting the limits of existing equipment.
How does CARES work?
Casale CARES can be visualised as a virtual cycle, where raw plant data is processed in the Casale data centre, where it is cleaned, corrected, structured and augmented to become a real source of information that can be used to improve plant operation. As shown in Fig. 2, Casale CARES is possible due to the interaction of different modules, including secure data transfer, analytics, monitoring, and reporting.
Secure data transfer
The first and essential step is to establish an automatic and secure plant data collection mechanism that transfers data from the client historian to the Casale data centre. Casale acknowledges that plant data is extremely precious, hence the established connection always satisfies the clients’ security requirements. Casale often uses OSIsoft® PI cloud connect technology (see Fig. 3) to achieve secure data transfer and minimum infrastructure adjustments. This technology is based on a software-as-a-service platform that allows sharing data between the plant historian and Casale’s data centre.
CARES analytics
The CARES analytics module prepares data received from plant data. It detects and removes outliers and sets the variance of all measured quantities. Once the data is prepared, data reconciliation can take place of the streams and units of operation present in the section or plant being studied and with the determination of KPIs of critical and proprietary units. Fig. 4 shows an example of the reconciled values obtained for the synthesis loop of an ammonia plant. Lastly, plant behaviour is evaluated and a root-cause analysis of underperforming assets is carried out using machine learning techniques. All analytics can be triggered in batch or continuous mode.
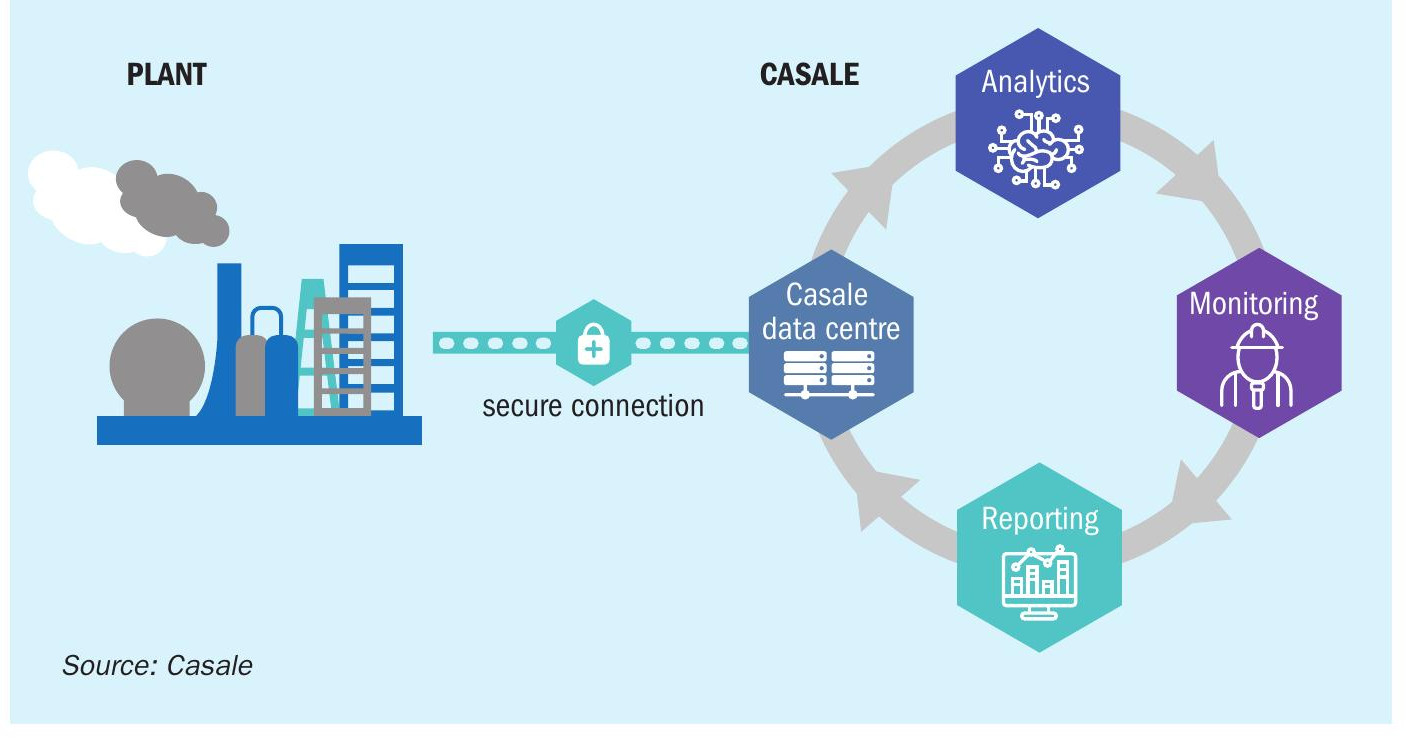
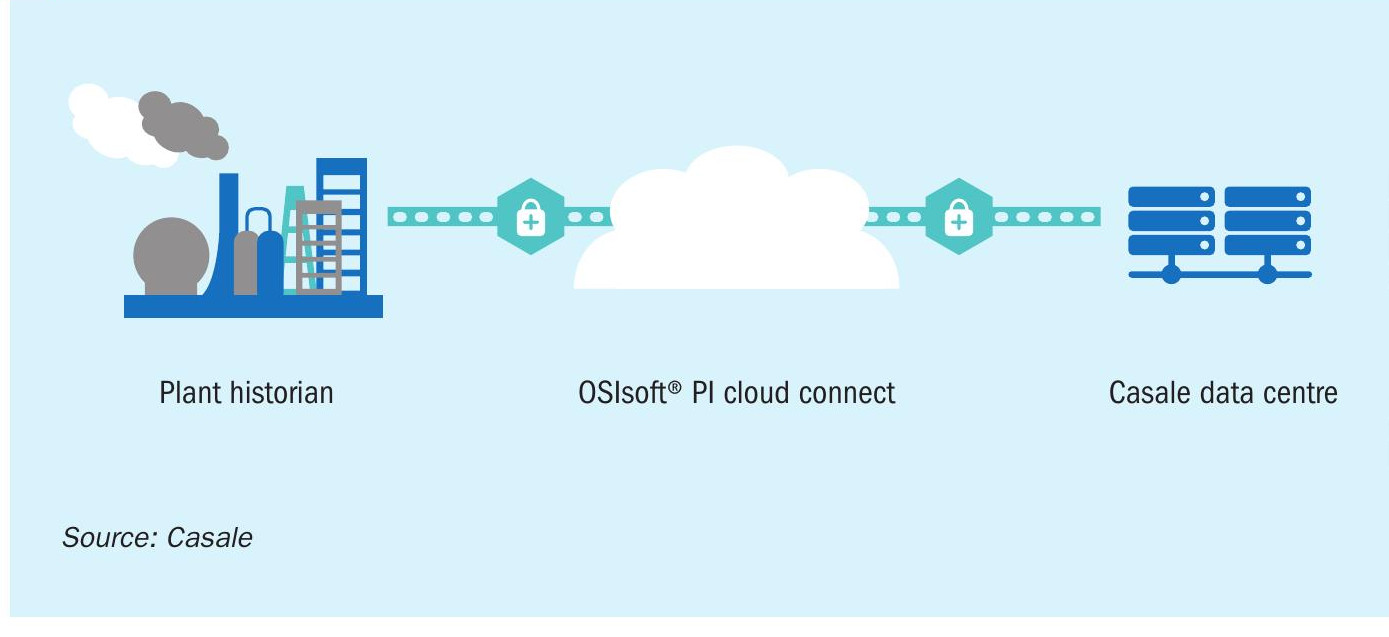
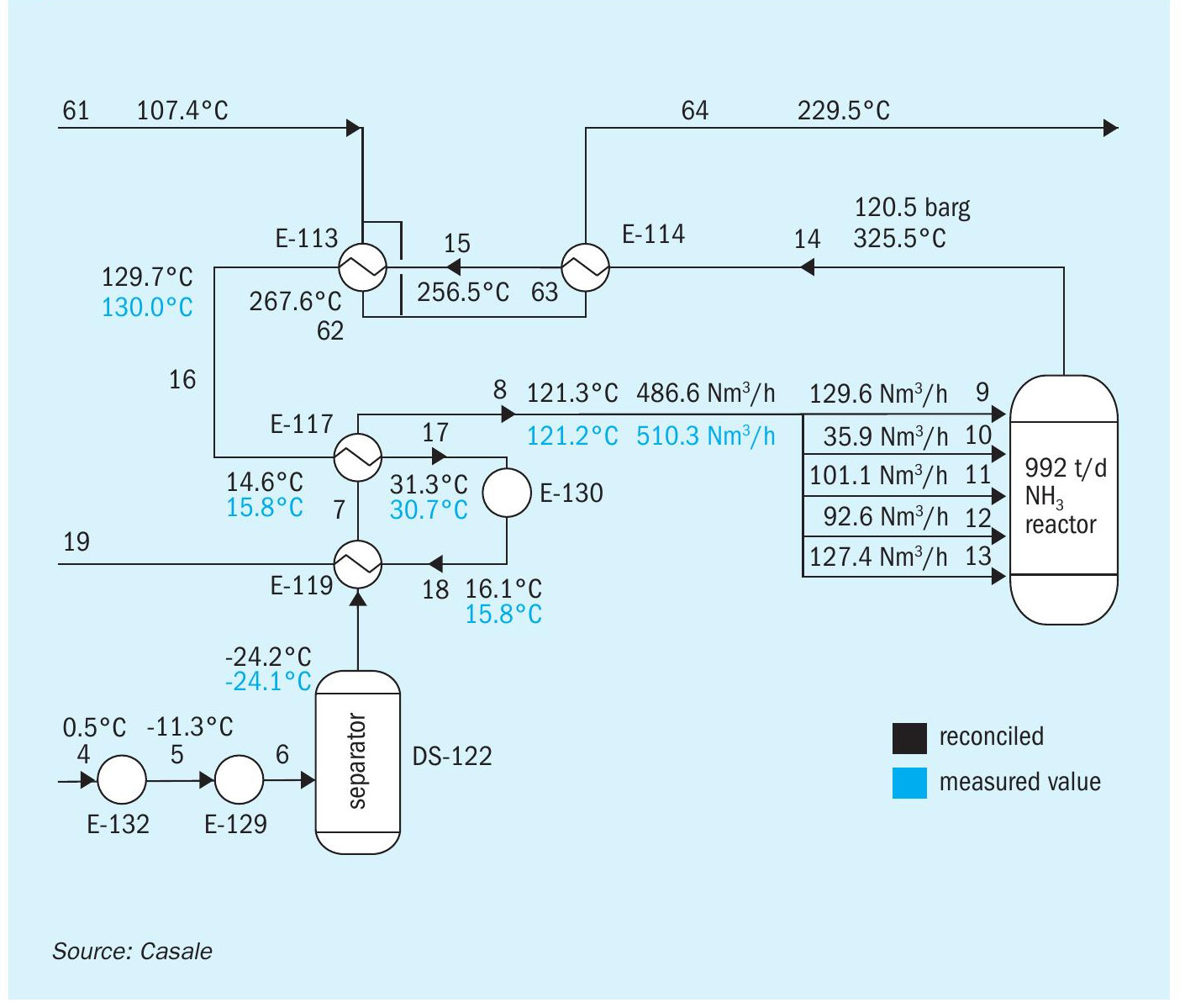
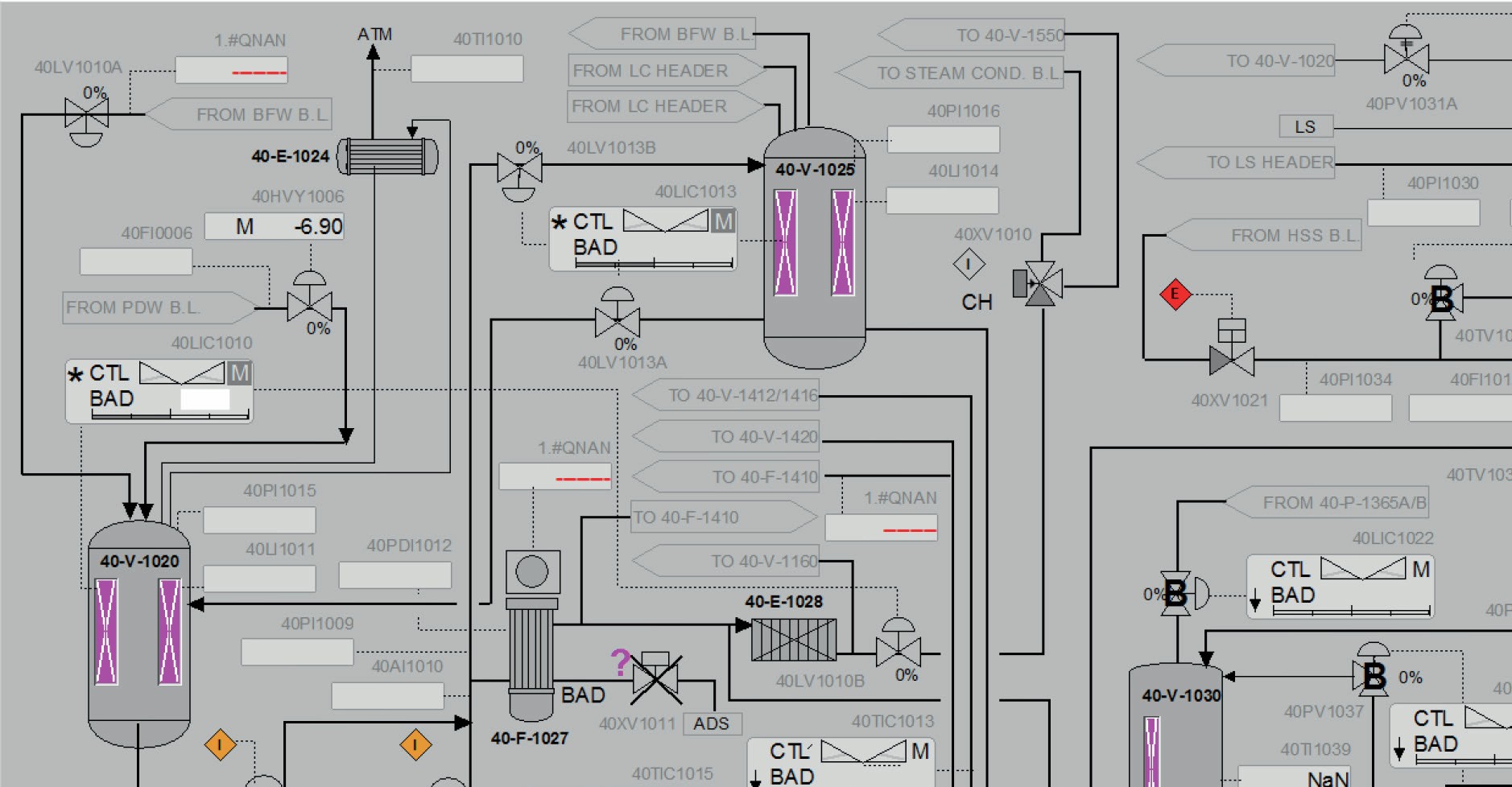
CARES monitoring
CARES is more than remote monitoring software, it is a true remote engineering service. Behind the scenes, a dedicated team of engineers will follow plant and asset performance. This is possible thanks to a specialised technical staff, that not only includes process engineers, who are a very important part of the structure, but also other subject experts dedicated to solving problems faced by clients, including instrumentation, machinery, reliability, and maintenance.
CARES reporting for valuable feedback
Providing effective feedback to the plant operational team is essential for a remote engineering service. CARES offers two types of reporting: a web dashboard, providing quick and effective plant performance visualisation, and periodic reports, presenting a detailed analysis of the performance of the assets and sections being studied during the last period. The reports also highlight identified states or bottlenecks that prevent optimal operation and stability of different sections of the plant.
Casale operator training system (OTS)
Training of operators is an essential requirement to improve plant performance, reliability, and safety. As a first step, operators learn how to operate the plant under normal conditions following design guidelines and field experience. However, to be prepared for unexpected or uncommon plant conditions, such as start-up, shutdown, incidents, safety procedures, etc. operators need special training to understand the behaviour of the plant under these conditions, as well as the associated risks. An OTS can help operators to quickly gain operational expertise on how their plant behaves under normal and special conditions, thereby improving the confidence and knowledge of operational teams. Casale’s operator training system offers realistic high-fidelity plant simulations that cover many different scenarios and operating conditions. Moreover, the OTS allows operators to start learning how their plant works, even before the plant is running, improving the quality of operations from day one.
Existing commercial OTS providers offer excellent graphical user interfaces and dynamic simulation capabilities that perfectly mimic the operating experience of the control room. However, due to the inherent complexity of certain chemical technologies and operating conditions, standard thermodynamic packages and kinetic models used in these products, are not enough to accurately capture the physics of the associated chemical processes. To overcome this limitation, Casale can partner with any OTS provider to embed its know-how and proprietary models into the OTS, drastically increasing the accuracy of the response of the training simulator. As a result, clients receive high-fidelity plant models tested and validated by Casale. For example, Casale and Honeywell have been working in partnership to deliver an OTS for the ammonia, urea and melamine plants for the Metafrax complex in Russia (see Fig. 5).
Benefits include:
- reduced operational risks, downtime, failures, etc.;
- the best high-fidelity OTS can be obtained thanks to proprietary models to simulate the plants;
- operators can be trained even before start-up.
Casale model predictive control (MPC)
Plants are often operated in the “comfort zone” meaning that the key operating parameters controlling the performance are voluntarily kept below their optimum. This is mainly because even the most experienced operator is not able to adjust the parameters rapidly enough to respond to plant fluctuations or external changes and to avoid hitting physical constraints.
A model predictive control (MPC) system is an automation system that complements the DCS to overcome these problems by constantly tuning the set points of the different control loops to stabilise the operation and push the plant to the optimum. As shown in Fig. 6, once stabilisation is achieved, it is then possible, using the MPC, to safely increase performance and to achieve higher production, or to reduce energy consumption. Depending on the client’s needs an MPC system can be implemented on the whole plant or on a section of it.
Casale has partnered with the most important providers of MPC software to offer a dedicated MPC product for all Casale technologies. The product has been developed by adding Casale process expertise to the most evolved industrial MPC software, such as Honeywell, Yokogawa, Rockwell Automation, etc. and have been successfully applied to several chemical industrial sectors.
How does Casale’s MPC solution help clients?
Most available MPC systems are a simple automation system, which applies purely mathematical algorithms to optimise selected plant parameters, named controlled variables (CV). These are considered the key players in the achievement of the optimum performance of the entire plant, thus they are strictly “controlled” and maintained at the optimum. This is possible due to continuous and smooth actions on manipulated variables (MV), which change the set point of controllers to correct the observed plant drift produced by external disturbances.
While this approach may seem sufficient, in practice it can only be applied to correct small plant perturbations for simple linear systems. Complex plants, such as those found in the fertilizer industry, can only be properly stabilised and optimised using custom thermodynamic and kinetic process models. This is because only through reliable models is it possible to identify and target the actual optimum of the critical controlled variables, which is not a steady figure (see Fig. 6), but dynamically changes depending on the real time capabilities of the whole plant. By using best-in-class MPC software, which incorporates Casale proprietary models, Casale’s MPC product achieves even greater MPC performance. The optimum process performance is achieved utilising efficient and robust process models, which constantly monitor the operation of the critical sections of the plant.
For its urea technology, Casale has made great efforts to develop MPC software for process optimisation, which is now available in the market. Among different functionalities, the software allows the efficiency of the urea synthesis to be maximised by dynamically targeting the optimum N/C, H/C and operating pressure in the urea reactor. This is possible thanks to the power of the physical process model which supports the MPC operation by suggesting the optimum value of the critical controlled variables depending on the real plant capabilities and constraints.
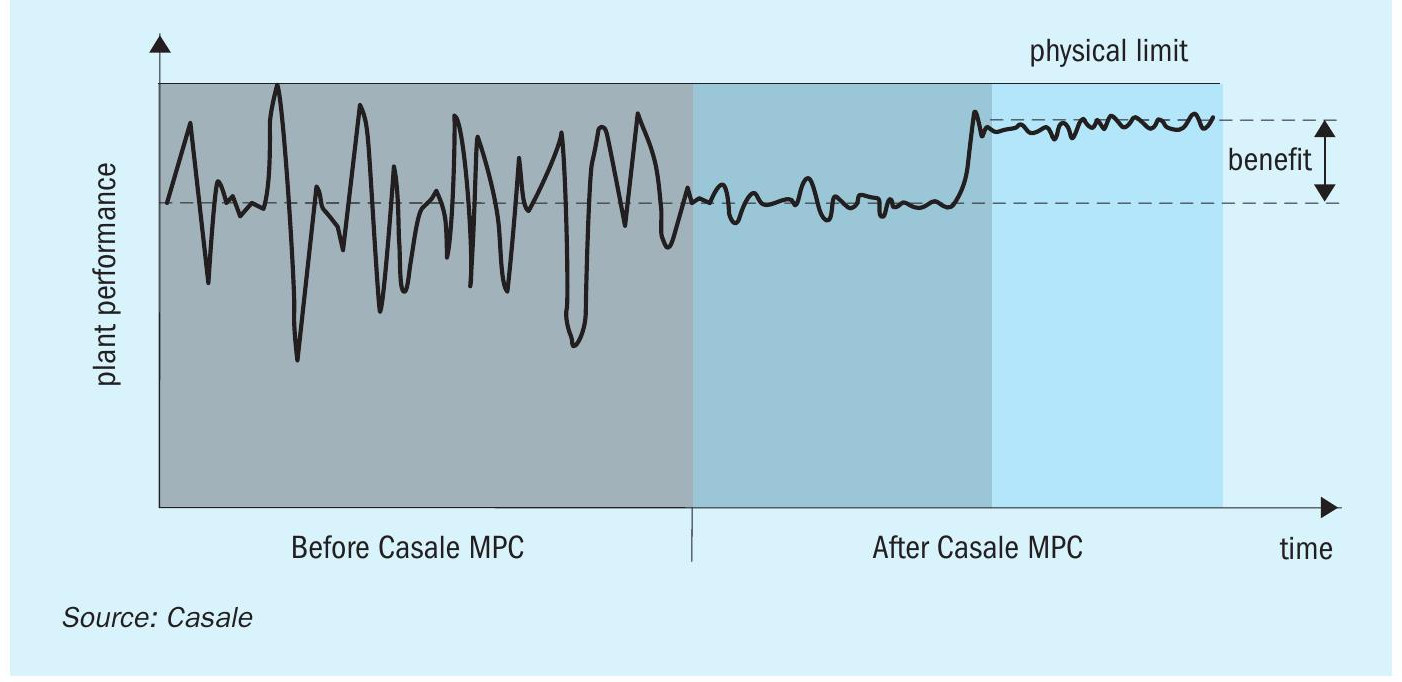
What is the optimum set of conditions for the urea reactor? Qualitatively one may know that by increasing the N/C ratio and reducing the H/C ratio the reactor performance is improved. However, optimisation of the process is not an easy task. For instance, increasing the ammonia may lead to a pressure increase in the HP synthesis, a decrease of HP stripper and HP scrubber efficiency, and to the increase of pressure in the downstream urea recovery stage, and a partial loss of vacuum in the evaporation unit. Ammonia that cannot be handled by the plant will make the process unstable, leading to an increase in the emissions and raw material consumption. Lastly, the excess of ammonia contributes to an increase in utilities consumption for recovering and recycling the extra ammonia.
The above considerations highlight that the optimum N/C is not a steady figure for a given process technology but may change plant by plant and for a given plant may depend on several other conditions. For example, the operating procedure of the process may suggest operating the reactor at N/C 3.1 but this should only be used as a guideline. The optimum figure will depend on plant, the actual condition of the equipment, the environmental conditions, etc. Only advanced MPC software that embeds the process knowledge can target such an optimum N/C ratio at all times and depending on the real capabilities of the plant and related constraints.
Similarly, the optimisation of the water loops in the entire process is complex, since they strongly affect the value of the H/C ratio in the urea reactor.
It is well known that water is needed to control emissions, pressure, and the composition of recycled solutions. Water is provided to avoid crystallisation and keep the process stable, but any excess impairs the synthesis efficiency. Water is efficiently controlled by the Casale MPC acting on critical parameters in the process condensate treatment, urea recovery and recycling sections. The MPC constantly tunes the plant downstream to stabilise the composition of the recycle streams, targeting minimum water content and fulfilling constraints e.g. for crystallisation, pressure control and emissions.
The Casale MPC drives the plant to achieve the highest N/C and minimum H/C in the reactor to maintain:
- plant stability;
- synthesis pressure;
- HP stripper and HP scrubber efficiency (CO2 stripping plants);
- NH3 venting in continuous and discontinuous streams;
- crystallisation of the recycle streams;
- stability and performance of vacuum evaporation section (vacuum pressure);
- product quality.
Casale smart instrumentation
All of the innovative digital products mentioned above work better when complemented by a robust instrumentation system. Casale offers a range of reliable tools and novel instruments that can support daily plant operations (Raman urea analyser, tunable diode laser, refractive index meter, HP urea service guided wave radar level, etc.).
Casale has developed several hardware solutions providing value-added quantities that cannot be directly or reliably measured via standard instrumentation.
For instance, Casale has developed innovative solutions for level, pressure, and composition, which are especially targeted at urea synthesis, monitoring the performance of the urea reactor and other critical HP synthesis units such as the HP stripper and the HP reactor.
Customers can now benefit from the newly-developed guided wave radar level transmitter and the improved HP diaphragm seals pressure transmitter for the urea synthesis. It is very important to ensure reliability in the level and pressure control in order to stabilise the plant.
The composition of any HP process stream is even more important and can be monitored in real time by Casale’s Raman urea process analyser.
Tunable diode laser (TDL) techniques are used for monitoring emissions in process vents.
The availability of urea grade materials and tailor-made engineering for the urea process allows further techniques to be implemented, such as the on-line measurement of the refractive index in the recycle streams of the process to support the control of recycling water.
These are just some examples of smart instruments available today to support process operation. Casale offers tailor-made solution for any kind of need the process may have.
Raman urea process analyser
The Casale Raman urea process analyser, a new powerful tool for on-line process analysis and control of urea plants, is a striking example of a smart instrument that can provide added value to digital services. Based on Raman spectroscopy, the composition of a process stream is determined by analysing a light spectrum created from a laser light source injected into the process stream via a special probe (see Fig. 7) The spectrum represents a fingerprint of the different chemical components present in the stream.
The Raman urea process analyser accurately measures the full composition of any high-pressure stream of the urea process in terms of NH3 %, CO2 %, urea% and H2 O% and provides KPIs of the urea synthesis, such as the N/C and H/C molar ratios, conversion and stripping efficiency. In this way, the analyser supports the everyday operation providing alerts for deviations of the most important parameters of the urea synthesis which can then be promptly corrected by the plant operators.
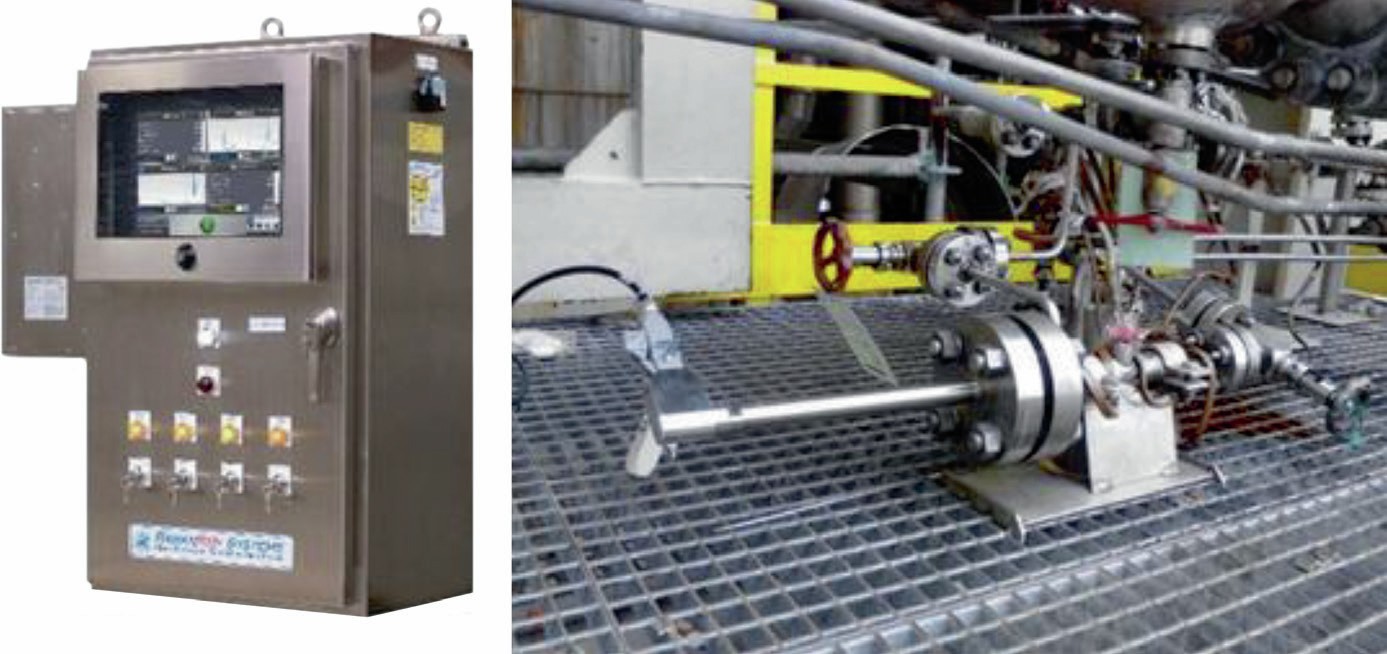
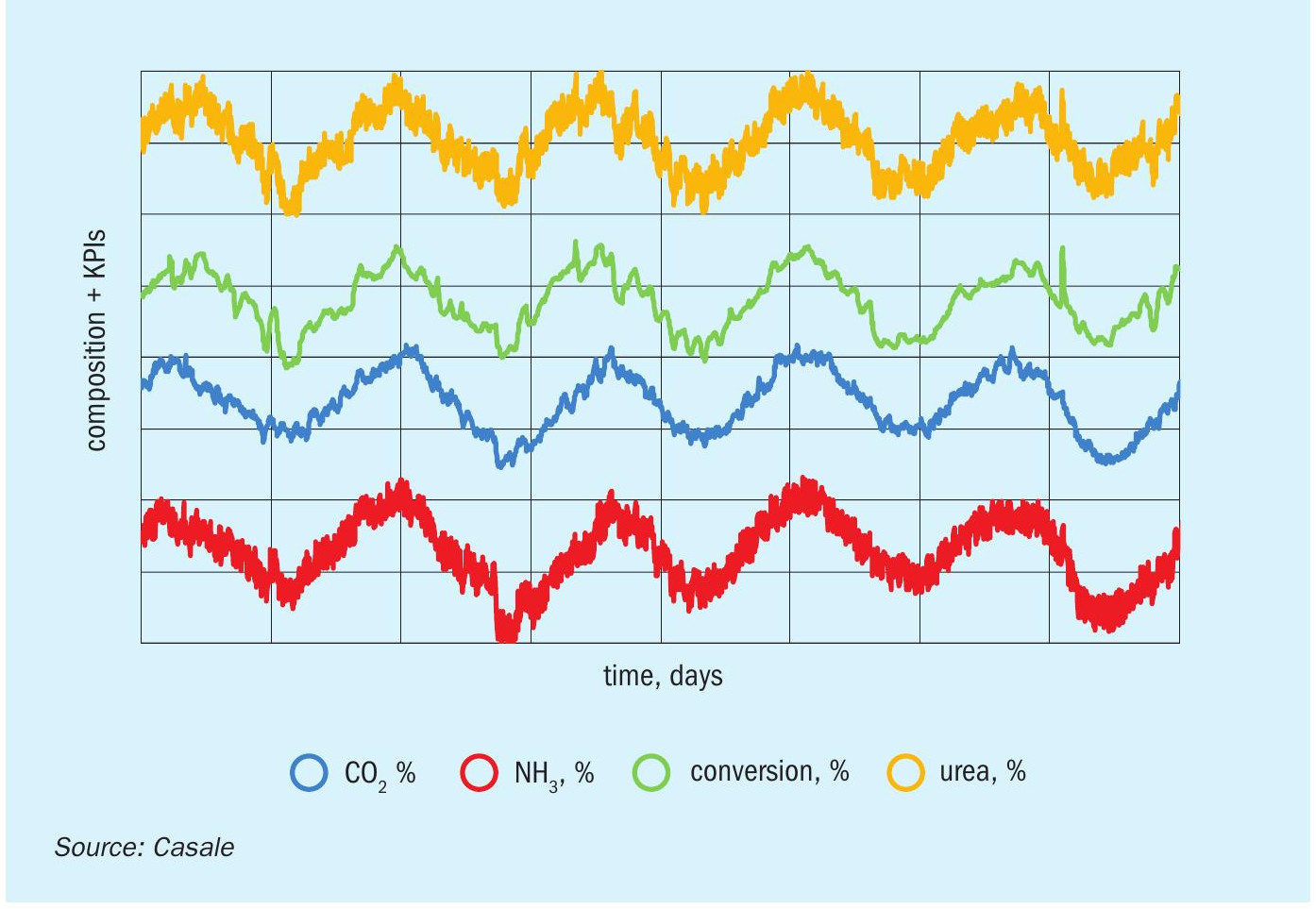
The Raman urea process analyser overcomes the limitations of traditional instrumentation for on-line analysis of the urea process, e.g. N/C monitoring systems, in which the N/C ratio is inferred from density measurements at the exit of the urea reactor. The analyser also automatically samples different locations of the urea synthesis section, i.e. reactor, stripper, condenser, scrubber, downstream lines, etc. resulting in savings due to the elimination of manual sampling. Other important benefits are the reduction of safety concerns related to sampling operations (in particular, due to the volatility of the involved components), and improvement in the quality of the results.
More importantly, the analyser provides real-time data. Lab analyses are normally p erformed according to standard procedures and require some hours to complete. In contrast, the Raman urea process analyser delivers real-time measurements of the composition of synthesis streams and related KPIs, capturing in this way the dynamics of the urea synthesis section. Fig. 8 shows the variation of the composition of a urea stream and related reactor conversion during several days of production.
The main benefits of the analyser translate into a reduction in the steam demand of the HP stripper by up to 5%, reduced emissions by up to 5 kg/tonne of ammonia and up to 3% increase in plant production.
SAIPEM
Saipem’s digital transformation
Over its history of more than 60 years, Saipem has always been at the forefront of technology, bringing innovative solutions to the industry and executing the most challenging projects. A roadmap for digitalisation is currently ongoing to embrace all aspects of Saipem’s activities. When this roadmap meets the expertise of Saipem’s proprietary Snamprogetti™ Urea Technology, new services can be offered to customers.
The main advantage that digital tools can provide is the management of huge data in a boosted way. Modern plants are equipped with a huge amount of analogue/ digital signal which are useful for plant control and safeguard; however, every plant engineer has struggled to find in this jungle of data the key parameters that can solve an operational problem or explain why a plant trip has occurred. Today, machine learning techniques, enabled by IoT platform, can be applied in order to analyse huge quantities of data and find correlations and sensitive parameters. These data can then be used to optimise the behaviour of the plant, identify root causes and prevent failures.
Snamprogetti™ Urea Technology is well known for its flexibility and ease of operation, thanks to the ammonia stripping process and the use of the MP decomposition section. This flexibility can be further enhanced when combined with optimisation of operating parameters. In the process of optimisation, the licensor can offer extensive knowledge of the correlations existing among the different sections of the plant (thus avoiding the optimisation of one parameter from jeopardising the performance of other equipment), robust simulation capabilities and the expertise accrued in the design of more than 200 plants over the world, including more than 60 revamped units.
A good example of the combination of licensor know-how with state-of-the-art digital technologies is the so-called “digital twin”, a perfect copy of the actual operating plant. The digital twin is based on plant process simulation and it is fed with live plant data; it can be designed in the engineering phase, connected and tested during plant commissioning, thus ensuring smooth hand-over to the production team, and kept continuously updated. Once online, the licensor can independently monitor the plant remotely, suggesting adjustments to the process parameters and evaluating possible optimisations. The remote monitoring enhances the collaboration and the decision making process between the licensor and owner, which is vital to maintain the optimum performance of the plant throughout its lifetime. It also fosters communication as it avoids the exchange of huge amounts of data and overcomes logistic constraints such as different time-zones.
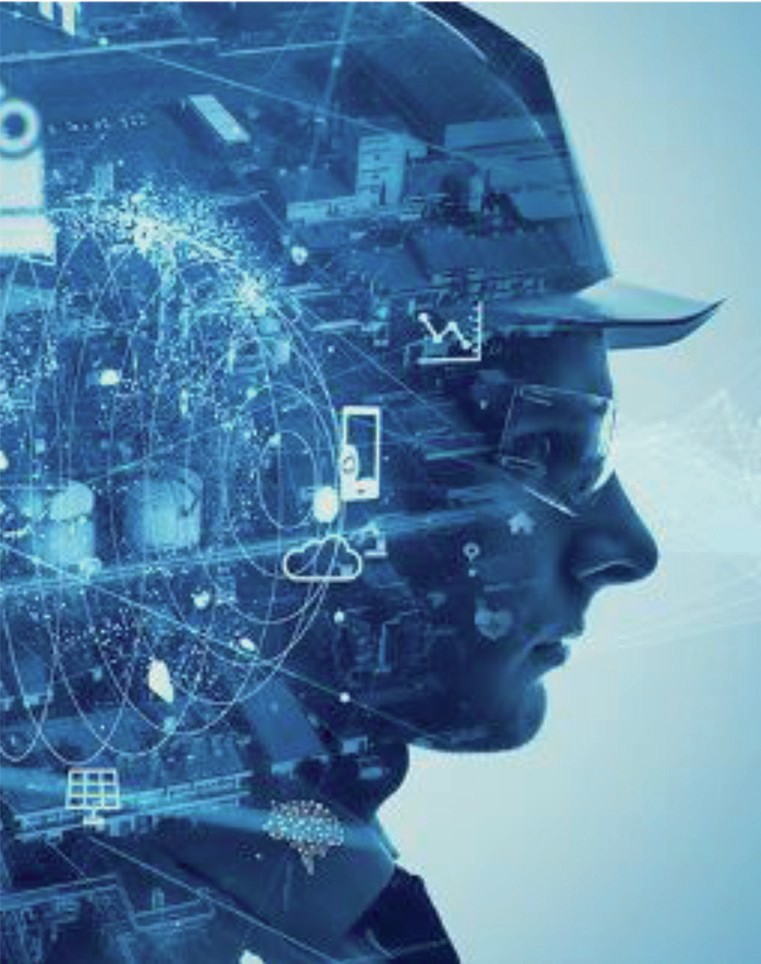
Big data analysis is also helpful in the unlikely event of a plant or equipment failure. In this context, it is highly useful to exploit techniques such as data science visualisation tools and neural network prediction capabilities, which will analyse the data much faster than a human, reducing engineering man-hours by up to 80%. This allows process engineers to better use their valuable time to promptly recognise the root cause and identify potential preventive measures or design changes to avoid reoccurrence of the event. When involved in the root cause analysis, the licensor can offer independent judgement to detect potential deviation from design conditions and compare the behaviour of the plant/equipment with other units installed elsewhere to reduce the potential causes.
In addition, neural networks can be trained to predict with high accuracy the performance of equipment, reproducing also the physics characteristic time of the phenomena. This process, called predictive analysis, is able to anticipate the occurrence of a future event (such as an item malfunction causing plant shutdown) or an abnormal operating condition, such as high pressure or temperature, matching past trends with current plant data.
Another field of application made possible thanks to the digital revolution is the so-called additive manufacturing, which consists of the 3D printing of objects with a layer-bylayer material deposition starting from metal powders. Even though this is not applicable to all materials used in the fertiliser industry, it may be very useful for specific, non-pressure equipment or part thereof which can be engineered to the specific needs of each plant without being constrained by the requirements of standard manufacturing (which for example limit the possibilities in terms of geometry and shape). Saipem has recently developed a few cases which perfectly fit within Snamprogetti™ Urea Technology, for example the re-design of the high-pressure carbamate mixer.
Nowadays the austenitic stainless steels used in the high-pressure parts of Snamprogetti™ Urea technology offer good corrosion resistance if sufficiently passivated. By using an additive manufacturing technique, the mixer has now been redesigned using a more corrosion resistant alloy (CRA) starting from powder raw material.
The mixer was redesigned to be printed with a suitable alloy through 3D printing technology based on selective laser melting (SLM), that can accomplish geometry optimisation with the absence of welding, allowing weight saving as well as improving corrosion and erosion resistance.
Different samples were printed using the selected alloy under laboratory trials in order to verify the behaviour in terms of physical corrosion and mechanical properties. In case of satisfactory test results for the material tested with this additive manufacturing technique, the mixer prototype will be delivered for the final field testing.
In future, the application of additive manufacturing can guarantee a continuous improvement of Snamprogetti™ Urea technology to reduce plant downtime, costly maintenance and customer’s spare parts.
STAMICARBON
Boost plant performance with real-time insights
The use of first principles models to support process operations have been practiced in the chemical and petrochemical industry for over 40 years, but, their use in urea plants has been limited. Urea plant models, however, can encapsulate a large amount of process knowledge and companies have started to realise the significant value using these models in offline and online model-based applications. Such applications include real-time optimisation, predictive control, data reconciliation, virtual (soft) sensors, process performance monitoring and total plant monitoring systems, to name a few.
Stamicarbon, the innovation and licensing company of Maire Tecnimont Group, is committed to a sustainable fertilizer industry, by investing amongst others in innovative digital solutions.
The Stami Digital Process Monitor is one of the products that forms part of Stamicarbon’s suite of digital products. By using a urea plant model as the engine of the Stami Digital Process Monitor, this monitor enables plant operators to optimise their plant operations, leading to an increase of the plant capacity, while reducing the plant energy consumption and plant emissions.
The Stami Digital Process Monitor feeds real-time data of a urea plant to a rigorous plant model which calculates key performance indicators (KPIs), such as plant load, energy consumption and emissions, as well as a variety of soft sensor key variables, such as equipment efficiency, equipment load and reactor load and shows them on a dashboard (Fig. 1).
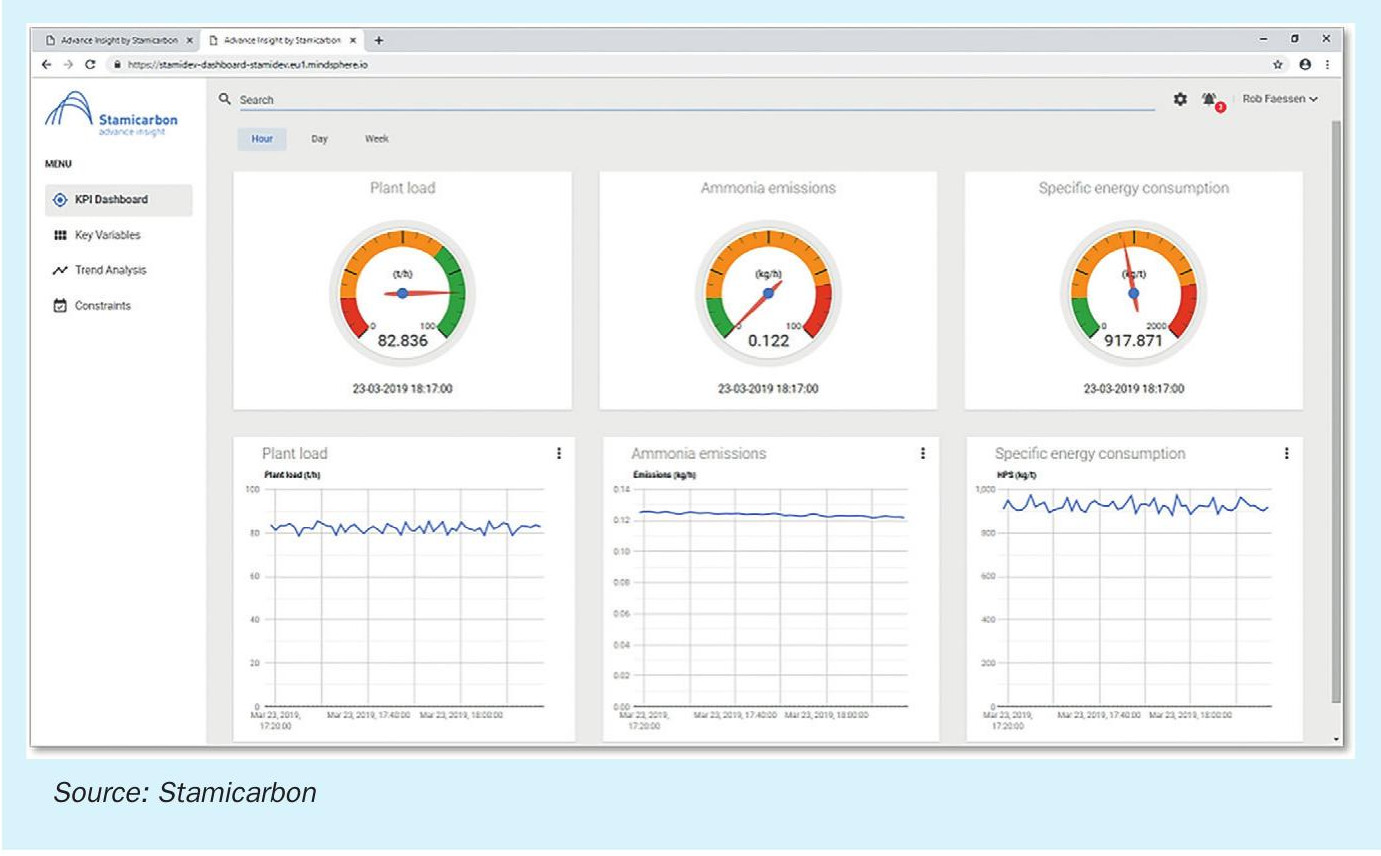
As illustrated in Fig. 2, the real-time plant data is unidirectional communicated to the process monitor engine, which runs in a secured Cloud environment. The plant data is processed by the plant model, which calculates the KPI and soft sensor key variables and are made available to the plant operators and all other stakeholders.
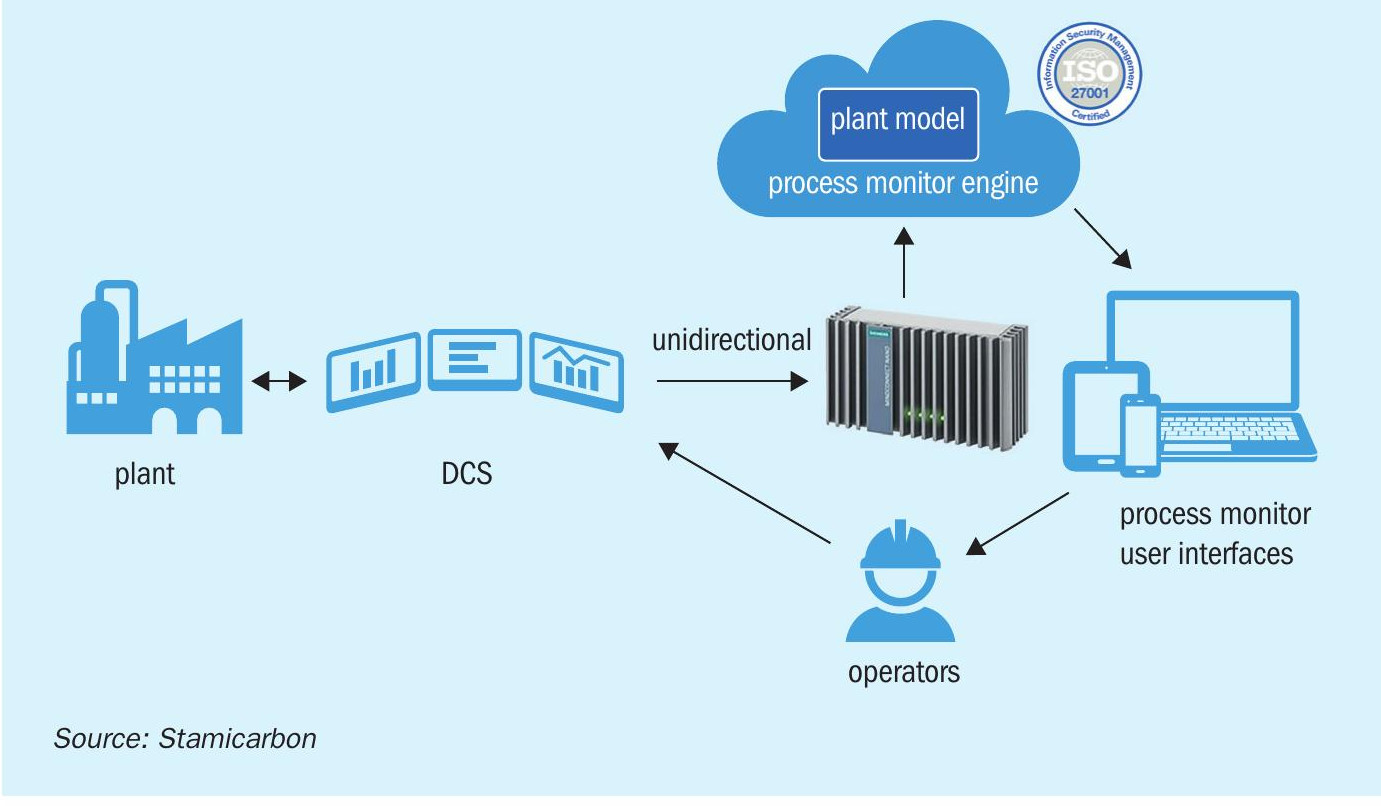
An overview of plant modelling
Depending on the level of a-priori knowledge, three different classes of models can be developed:
- knowledge driven models;
- data driven models;
- gray box models.
In contrast, data driven models, also called black box models, are proposed for situations in which physical understanding of the urea process is absent or not relevant (these models are based on experimental data which are fitted to typically simple equations).
In between the two extremes, there are many possible combinations of knowledge driven and data driven models. The prior knowledge offered by the simplified first principles analysis forms the core of a so-called gray box model, while data driven methods can compensate for fractions that cannot be modelled easily in terms of phenomenological models.
The Stamicarbon process model is purely knowledge driven, with superior prediction quality. The mathematical model developed includes mass and heat transfer equations, reaction kinetics, vapour-liquid equilibria, hydrodynamic aspects and covers the entire plant.
In total, the plant model consists of more than 5,000 linear and non-linear equations. An in-house developed equation-oriented flow sheeting program is used to ensure fast solutions of the large and complex problem.
Difficulties in urea plant modelling
Despite the simple chemistry in urea plants, the underlying physics of mixtures containing urea and carbamate is complex. First, the feedstock to the synthesis is in supercritical state. Above the critical points, distinct liquid and gas phases do not exist, which gives serious deviations in liquid/vapour-descriptions. Second, the presence of an azeotropic ratio for mixtures containing urea/carbamate/water creates solutions showing large negative deviations from Raoult’s law and form a maximum boiling azeotrope at a specific composition. Inflection points caused by strong non-linearity are often the source of numerical instability, as the solver algorithm cannot distinguish from which side to climb the hill. For online application, the model must meet a set of performance requirements with respect to speed, robustness and flexibility; already a challenge by itself!
Plant model steps
The plant model of the Stami Digital Process Monitor is produced in two distinct steps: a consulting phase and a data reconciliation phase.
ADVANCE™ CONSULT is an offline model based service, offered by Stamicarbon. It starts off by conducting interviews with plant experts and operators as they play a key role in fully exploiting the wealth of historical data. The experiences and expertise of those involved in day-to-day operation provide valuable insight into relevant process changes and performance of measuring devices, etc.
With a validated plant model and historical plant data, an in-depth analysis can be made from the plant operation.
The report will typically indicate:
- evaluation of plant sensors;
- plant constraints and proposed solutions;
- achieved operation relative to key variables;
- low frequency oscillations and disturbance rejection (e.g. day and night rhythm);
- recommendations for improvements.
Data reconciliation
To ensure that the model represents the process accurately, historical DCS data are collected from the operation and are subsequently used to validate the model. The measured data typically contain random errors, and less frequently, may also contain systematic errors.
The role of the data reconciliation is to properly identify and correct deviations, and therefore provide reliable information for updating the parameters of the model, or to conclude on systematic sensor errors.
For process control engineers, offline validation can be viewed as a feedback process, aiming to bring the model predictions on target to the process measurements, similarly to the way feedback is used in a control application to bring measured values to requested target values or within ranges.
A validation example is illustrated in Fig. 3.
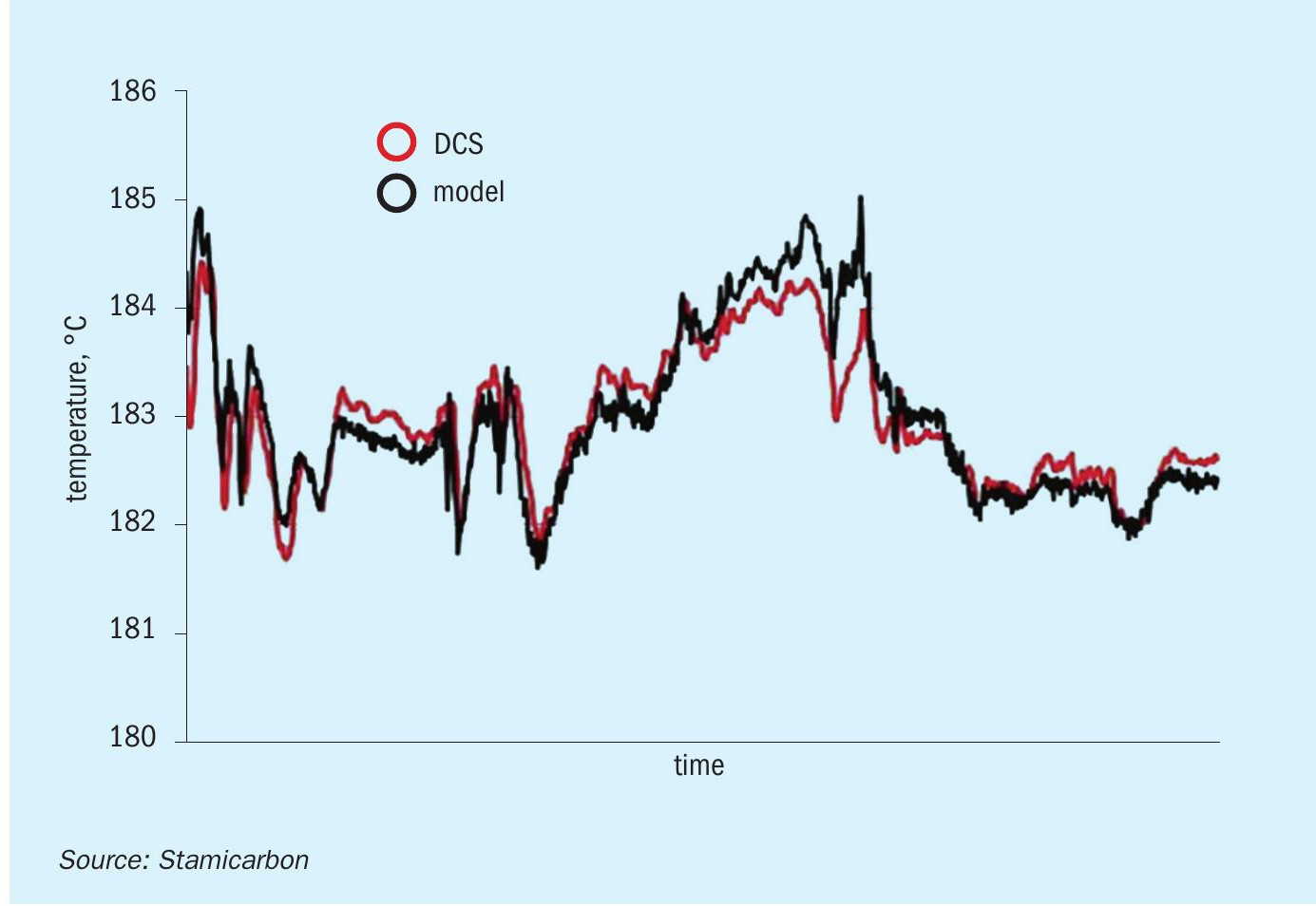
The urea reactor outlet temperature is typically a critical variable in urea synthesis. To some extent, it represents urea conversion in the reactor, assuming other interacting variables being constant. The plot in Fig. 3 shows the model predicted temperature, compared with the DCS reading over a period of two weeks. Accuracy is the level of agreement between the predicted and DCS values, while reliability is the degree to which the prediction errors vary.
The accuracy of identification and reliability of validation procedures is sensitive to the size of the corresponding datasets. The required size of the historical data is typically one to two months; hourly averages will do for analysis.
Once the advanced consult and validation phases have been completed, the Stami Digital Process Monitor provides real-time KPI and soft sensor key variables information, real-time insights, that will help to maximise efficiency and increase production.
Soft sensor key variables
Key variables (KVs) characterise the technical and economic performance of the urea process. Once KVs are fixed, the operating points are fixed. KVs are normally determined by offline sample analysis in laboratory. One of the drivers of process modelling was to give operators and process engineers a reliable and accurate estimation of key variables. This would allow them to arrive at the optimum operational point and assist them in trouble shooting activities. For example, the urea yield in a reactor is fully characterised by its key variables N/C, H/C, retention time, and system pressure. Only N/C can be measured in real time, whereas H/C and system pressure are determined by offline sample analysis in laboratory. Calculation of retention time would require a total mass balance calculation over the reactor.
The Stami Digital Process Monitor produces these real-time soft sensor key variables (see Fig. 4), enabling advanced process monitoring and optimised control of urea plants.
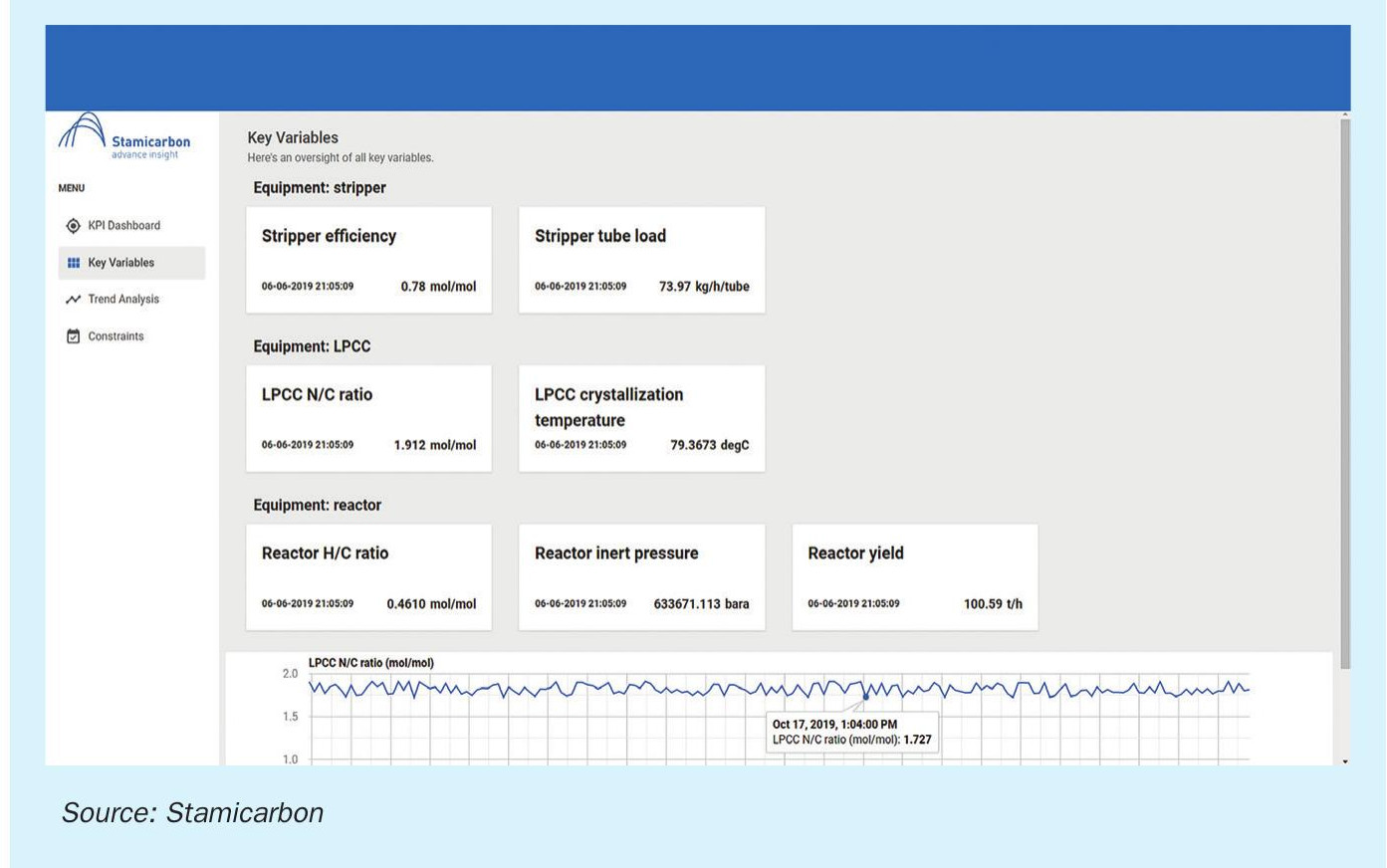
Troubleshooting
The Stami Digital Process Monitor also provides means for troubleshooting of the plant operation by comparing the plant DCS values with the values calculated by the plant model, as per the example shown in Fig. 5.
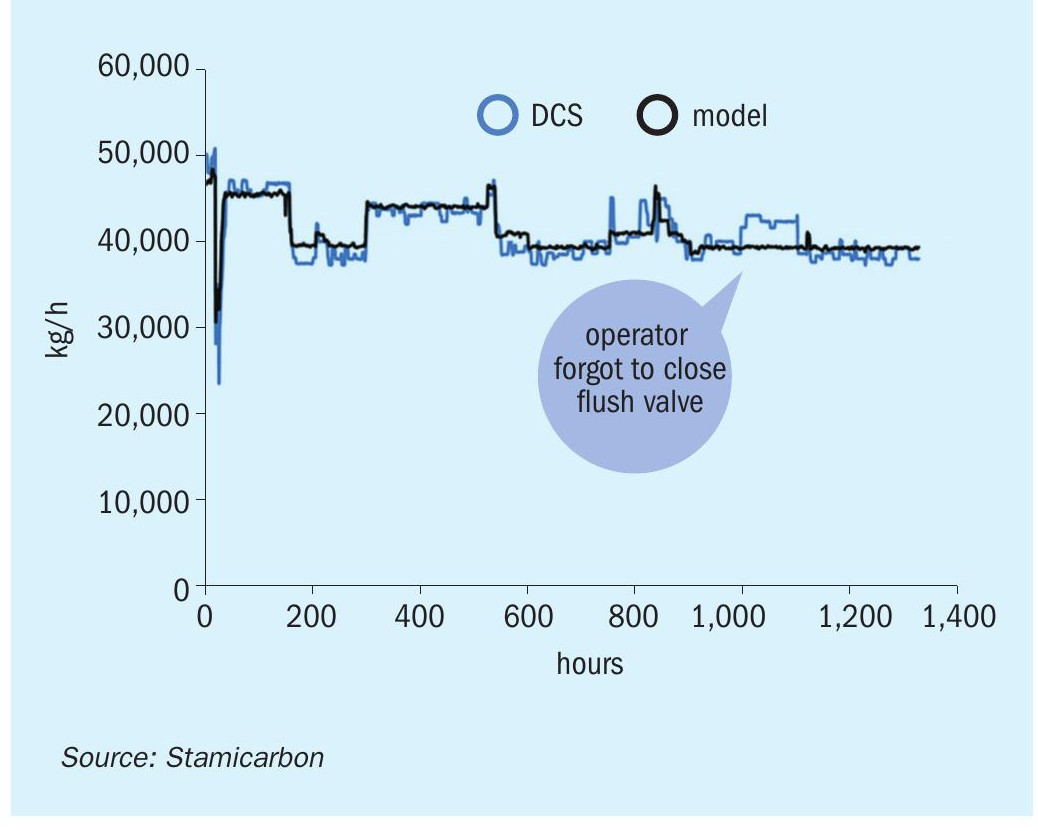
In the trend graph the DCS data is shown in blue colour and the values calculated by the model are shown in black colour. It was found that a flush valve had been unintentionally left open, which explained the fairly large difference shown in the highlighted area. The Stami Digital Process Monitor will immediately show such a difference, which will trigger the plant operator for corrective actions and prevent unnecessary loss of production.
Another troubleshooting example is shown in Fig. 6. In this case the trend shows a constant offset between the compressor load as per the DCS value shown in red colour and the value calculated by the process model. The root cause of this particular offset was found in a wrongly configured density compensation of the orifice. As a result, the compressor can be pushed to a higher capacity which leads to a higher plant load.
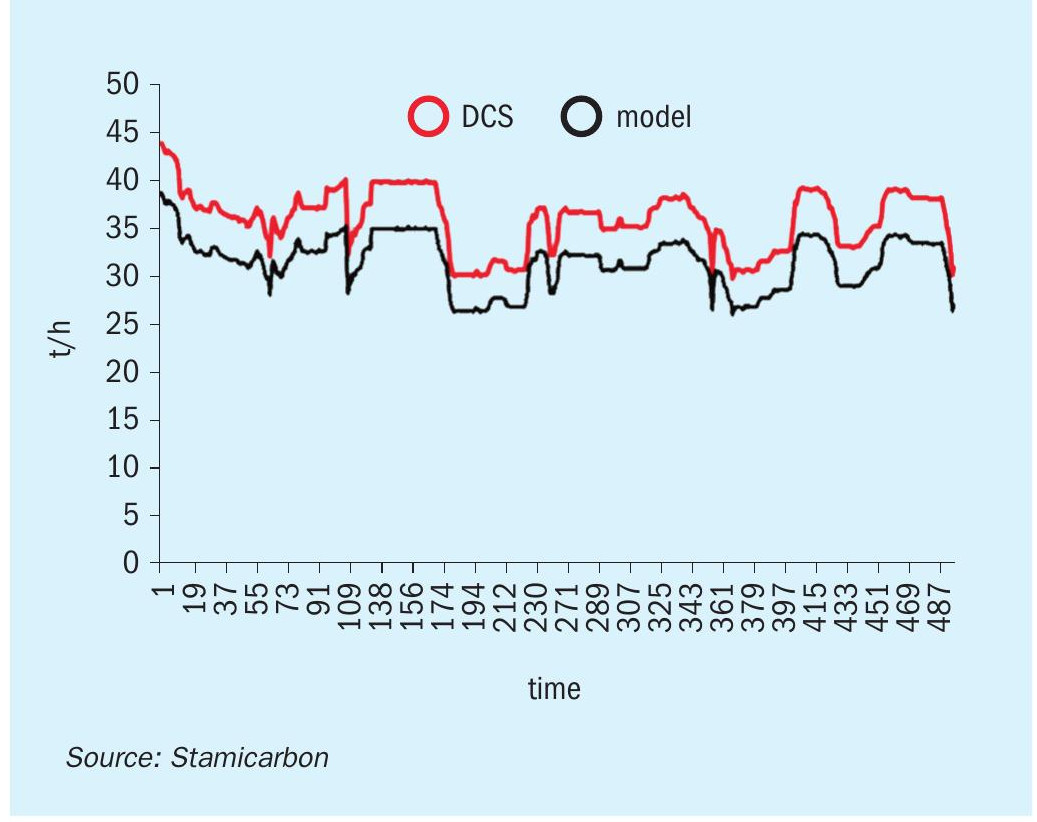
Features
The Stami Digital Process Monitor supports the following features:
- Key variables: All plant key variables can be monitored on a real time basis.
- Laboratory: The number of offline analysis in the laboratory can be significantly reduced, as the Process Monitor can calculated all these analysis as well.
- Emission monitoring: The ever increasing stringent regulations on ammonia emissions require reliable real-time monitoring systems. Ammonia emissions from absorbers can continuously be measured with high accuracy.
- Sensor duplication: Every plant sensor, which is not a model input, will be duplicated by the model. Having such a soft equivalent is a powerful tool to identify sensor and process deviations.
- Soft N/C meter addition: For Stamicarbon plants with a high-pressure falling film condenser (HPCC), conventional plants and thermal stripping plants, the model can be configured with an accurate soft N/C-meter.
- Flammability limits: In the absence of a hydrogen converter, the purge gas from the synthesis might be within flammable limits. The flammability limits can be predicted, provided that the impurities in the feedstock are measured. This can be further supported with a graphical dynamic interface showing the operating point relative to the flammable area.
CLARIANT
ReforSafE™ for improved safety and performance in syngas operations
In today’s cost competitive environment, syngas plants must perform at their full potential to survive and succeed. Cutting-edge thermal imaging services and catalyst solutions can help ensure ideal steam reformer conditions for optimum efficiency.
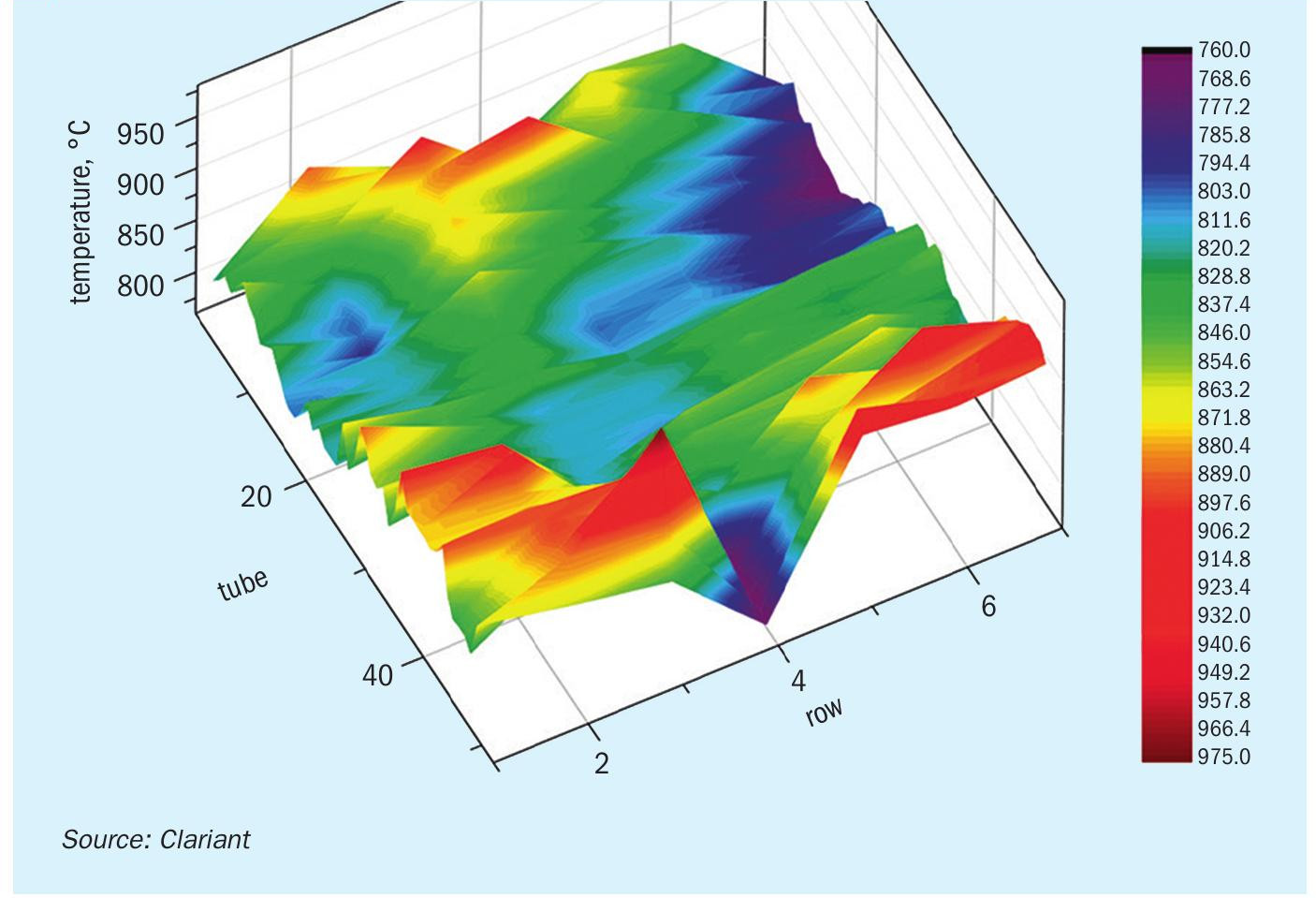
Importance of reformer tube temperature
The steam reformer is the heart of any syngas plant and the unit that is the most costly and energy intensive. It is a complex unit that operates very close to metallurgical limits. Its improper operation can result in considerable hardware costs, production loss, and plant inefficiency. Therefore, optimising its performance is key to improving overall plant profitability, and even small improvements can amount to significant financial benefits from energy savings or production increase.
Ensuring well balanced and reliable operation of reformer tubes is essential for improving the overall efficiency of the plant. For maximum efficiency, a high performance catalyst should be used to boost the chemical reaction in the tubes, and the internal heat distribution amongst the tubes must be regularly examined, evaluated and optimised.
To evaluate and optimise reformer performance, the most valuable measurement required is the tube wall temperature (TWT). This should be uniform, and 20°C below the design temperature of the tubes to obtain maximum production while still operating at a safe condition within the integrity operating window of the tubes.
The thickness of the tubes is designed for a lifetime of 100,000 hours of operation at design conditions. Overly cautious firing may lead to lower production and/or higher energy consumption. Several syngas producers are reporting a 2% decrease in production when operating at low TWTs. Conversely, operating at higher TWTs can cut the lifetime of the tubes significantly. It should be mentioned that various factors, such as catalyst deactivation, incorrect burner settings, or uneven firing, can raise the TWTs above design limits, causing overheating of the tubes. Unless mitigated, the mechanical stress on the hot tubes will increase exponentially, causing a drastic decrease in tube lifetime. In the worst case, a tube may rupture, forcing the plant to shut down immediately, and resulting in costly hardware replacement and production loss.
ReforSafE™ technology
To prevent these adverse scenarios, Clariant offers an advanced and reliable service package for evaluating and optimising the steam reformer. Clariant’s reformer survey allows plant operators to identify bottlenecks, increase tube lifetimes, maximise catalyst potential, and reduce energy consumption to boost plant productivity while minimising the consumption of energy and resources.
Clariant’s reformer survey examines and optimises key indicators of steam reformer performance:
- tube wall temperature (TWT);
- steam-to-carbon ratio;
- excess combustion air;
- furnace draught;
- operating temperatures;
- burner performance;
- heat distribution;
- catalyst activity;
- methane slip.
Clariant’s syngas partners can choose between three levels of reformer analysis, which differ in their degree of details concerning collection and examination of data.
ReforSafE™ Standard
The first level is a quick TWT check, performed with a Land Cyclops 100L pyrometer. Clariant experts will visit the plant to carry out visual inspection of the furnace, and to collect relevant information such as steam-to-carbon ratio, and methane slip to evaluate the performance of the catalyst. The service includes TWT evaluation through peepholes and temperature correction.
Scope of the first service level:
- ensuring safe reformer operation;
- evaluation of TWT;
- evaluation of catalyst performance.
ReforSafE™ Premium
Both burners and TWT are evaluated through the peepholes with a high resolution thermal imaging borescope that measures temperatures from 600 to 1,800°C at a short, accuracy-enhancing wavelength, and provides a thermal image of 656 x 494 px (+320,000 temperature points). This real-time, 3-D heat profile includes all tube rows of the furnace and can be easily examined for areas of inhomogeneous heat distribution and hot spots on the tubes. The equipment also provides live thermal images of reformer tubes, burners and refractory. The comprehensive temperature survey also includes recommendations for burner adjustments.
Scope of the thermal imaging service:
- same as pyrometer measurement, plus;
- evaluating the performance of burners and fuel headers pressure;
- providing recommendations for burner adjustment and optimisation.
ReforSafE™ Premium Plus
Readings are taken with a LAND gold cup reflector, which provides superior accuracy, as its near-perfect reflectance blocks background radiation, thus creating a black body for measurements. Using direct tube contact, the gold cup creates a seal between the tube surface and the instrument, shielding the thermocouple from additional radiation around the tube.
This is necessary since the tubes are typically cooler than their environment, especially the reactor wall. When measuring a cooler target in the hot surrounding, the radiation of the hotter surrounding reflects at the cooler target and causes a higher temperature measurement than the actual temperature of the target. The larger the temperature difference or the closer the tube is to the wall, the larger the error.
The gold cup measurements provide extremely accurate temperature data, as they are not affected by tube emissivity, hot background reflections, or side path emissions. The data gathered is used to calculate a formula for temperature corrections of future pyrometer measurements.
Scope of the thermal imaging plus gold cup service:
- same as thermal imaging service, plus;
- determination of thermal efficiency of the furnace;
- determination of tube emissivity;
- providing a correction formula for measuring true TWT;
- operator training on how to measure and correct true TWT.
Troubleshooting for reliable operation
The reformer survey indicates any problems with the burners and the refractory to ensure that all tubes are operated within their design temperature. This not only extends the lifetime of tubes but also that of the catalyst as thermal stress is minimised. Furthermore, catalyst performance can be evaluated based on the reformer data. Substandard performance, due to poisoning, coking or aging can be monitored, and corrective actions, such as catalyst steaming to remove coke formation, can be initiated to recover catalyst activity.
Optimising overall reformer performance
By fine-tuning different furnace parameters, plant operators can achieve higher methane conversion, and reduce their energy consumption. If pressure drop in the steam reformer is a limiting factor, upgrading to a catalyst with extremely low pressure drop, such as Clariant’s ReforMax® LDP Plus series, can help remove this bottleneck, and increase the plant’s production capacity.
NAVIGANCE
Data-driven chemical process optimisation
Navigance is a tech startup recently created by Clariant: a cloud-based solution providing real-time recommendations to optimise control parameters in chemical processes. Its innovative approach, combining hybrid plant models and machine learning with process and catalyst expertise, can deliver continuous benefits within weeks, without tying up inhouse resources.
Fine tuning a chemical process such as methanol production for optimum efficiency and reliability is an art. Keeping plant systems not only running, but running at their best, takes years of frontline experience and expertise to master. And, despite the many digital advances now within plants’ reach, it still takes a considerable amount of educated guesswork in many cases, too.
One of the key challenges for chemical facilities seeking optimisation is their sheer complexity. Their many interlinked and overlaid processes and variables make it difficult to build realistic first principle plant models that describe real-time process behaviour. The control systems commonly in use are also insufficient to enable dynamic, nonlinear process optimisation.
Unlocking hidden potential
The power to optimise effectively lies in the data many chemical plants already routinely gather. Successfully tapped, it can help improve operations in both the immediate and longer term.
However, the investment of time and resources needed to study, interpret and extract meaningful insights from immense data sets often precludes any real practical use beyond KPI development and dayto-day troubleshooting.
As a result, most process optimisation decisions are still taken using informed intuition and deep rooted experience of process behaviour. Even for the most experienced operators, making choices based on an incomplete picture of plant data can leave uncertainty as to whether the right decisions are made every time.
Machine learning, a subset of artificial intelligence (AI), offers a way to reduce these uncertainties. Deployed correctly and based on the right foundation, it can pinpoint and distil the data that matters most and identify the changes which need to be applied to process control variables to achieve operational goals.
A fresh approach
Such capabilities require fresh thinking and a broad range of expertise, such as sufficient domain knowledge in the chemical process to develop models robust enough for AI-based optimisation and the mathematical and statistical know-how to develop and implement machine learning effectively.
Clariant took up the challenge, investing in Navigance: a new venture and innovative service for chemical producers that harnesses its rich catalyst and process expertise and decades of industry experience.
“What started as an internal initiative within Clariant Catalysts has developed into an entirely independent business and company using digital technologies to offer process optimisation services,” says Stefan Heuser, Senior Vice President & General Manager at Clariant Catalysts. “It’s a journey that underlines our commitment to delivering and generating value for different players in the chemical industry.”
Acting with intelligence
In a purely technological sense, Navigance is a service that enables producers to continuously optimise their chemical process using their own plant data. It provides this powerful support through a cloud-based platform.
Machine learning studies and identifies complex, non-linear patterns in historical and real-time plant data, predicts future performance and spots improvement potential to a high degree of accuracy. These insights are presented as recommendations in an online dashboard.
Operators can act on this advice immediately, adjusting control variable settings to continuously optimise and achieve their defined goals. These may be efficiency goals, such as yield, production output and other operational targets. Equally, the intelligent algorithms used can also identify any deviations and so help to maintain operational reliability too.
Partnering for success
Navigance is more than just a technology solution. Its team works closely with producers through their entire journey towards data-driven decision making – then beyond.
The approach of Navigance is not only to provide AI and data analytics tools to gather data and present findings, but also to deliver prescriptive recommendations and, crucially, provides ongoing expert support.
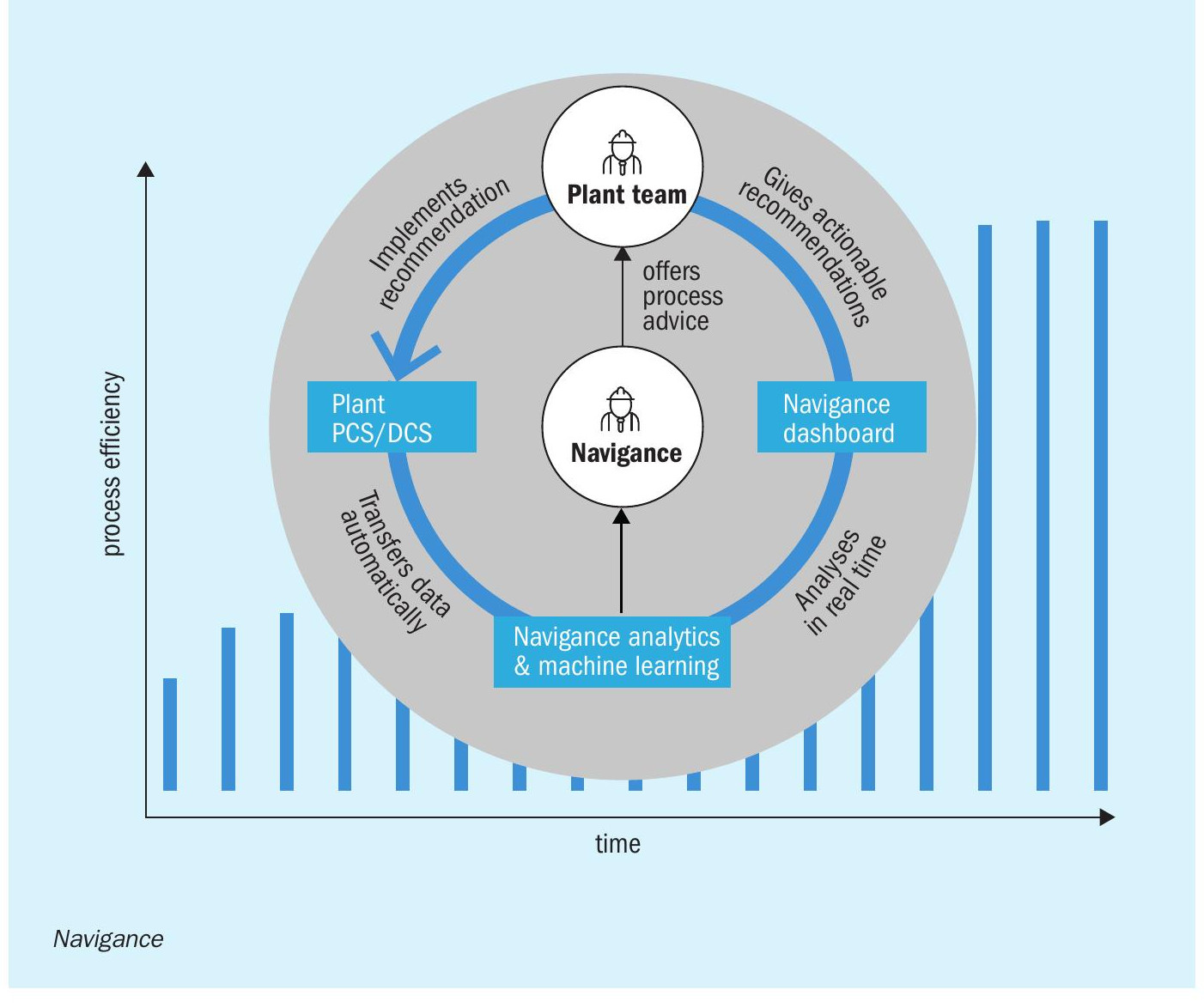
This starts with planning and deployment. Navigance process and data science experts take the pressure off chemical producers to find in-house resources to introduce real-time optimisation successfully themselves. This expertise remains available once the platform is up and running, providing insights and advice drawn from the data over time that help optimise further, even as conditions change.
The power to act on the recommendations always remains firmly in the operators’ hands. Enabling them to optimise based on a clearer picture of plant performance, while freeing them to focus on the many other pressing aspects of running their operation.
Right from the start
While some AI projects fail before they really get started, Navigance helps plant teams identify upfront where digitalisation will deliver great value and the right usage cases. This starts with a check of their digital readiness and potential key areas for improvement.
A feasibility study conducted by Navigance helps to understand the plant, its process, logical connections, key variables and constraints. It also determines if there is sufficient quality and quantity of data to build a realistic digital process model, and what is needed to complete this picture if not.
Navigance builds hybrid plant models that enhance established first principle techniques with machine learning. By carefully integrating unknowns using arbitrary functions, these models can ensure a good fit for all observed data and that all relevant effects are captured, for recommendations of a high predictive quality.
This approach enables hybrid models to respond quickly and easily to real-time data, changing conditions and factors that could affect processes, such as varying load scenarios and deactivating catalysts.
Fig. 1 shows the process optimisation cycle with Navigance.
Ready to optimise with intelligence?
Navigance works with all technology licenses and catalyst types and can suit both older and newer plants, whatever their current level of automation.
Base models, tailored to a specific process, enable producers to optimise quickly to reach their individual goals, with little demand on their in-house teams.
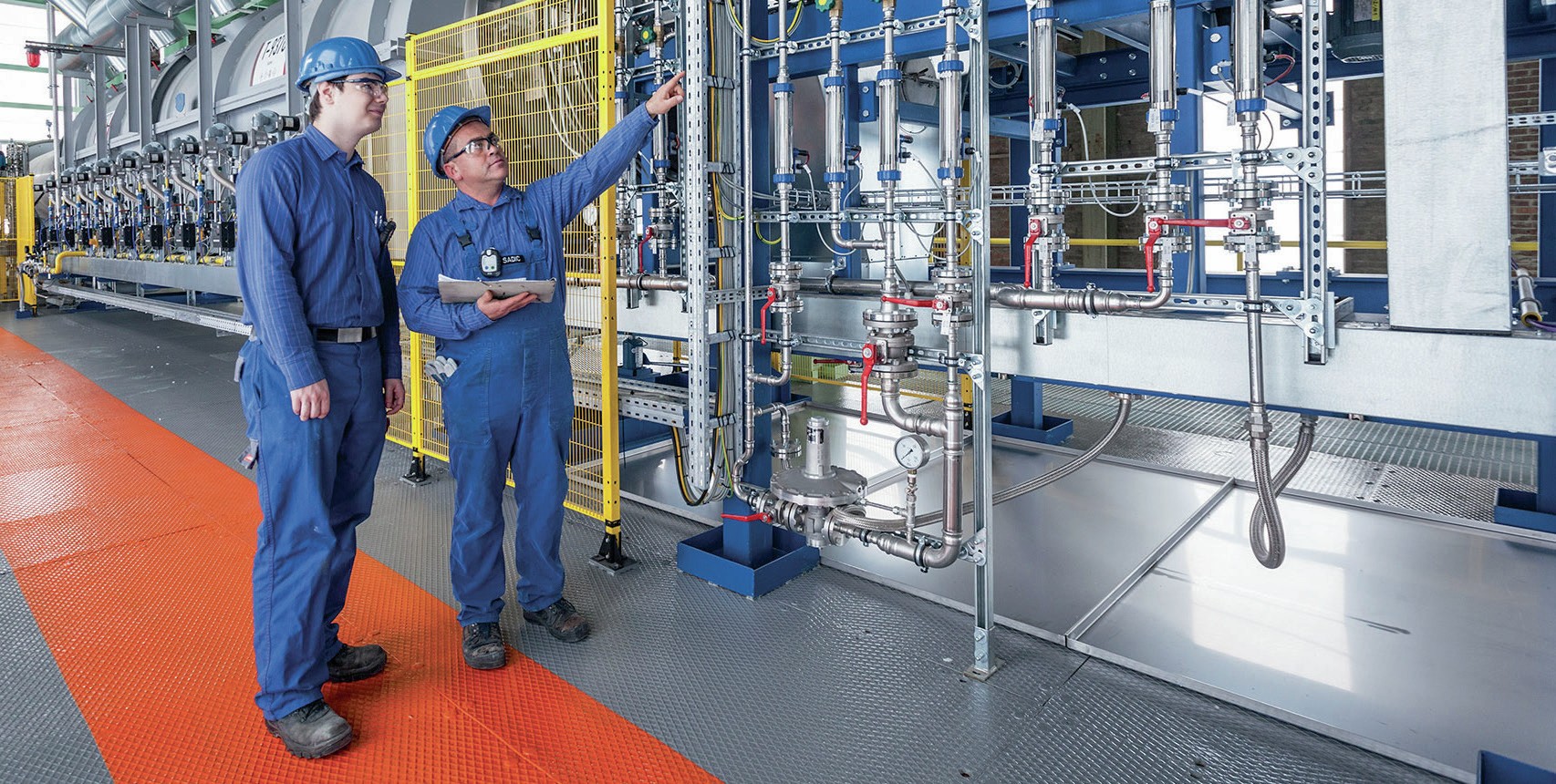
JOHNSON MATTHEY
Enhancing technical services with digital technologies
Johnson Matthey (JM) provides an extensive range of on-site and remote technical services, based on deep process and catalyst knowledge and the expertise of its engineers across a range of chemical processes. The introduction of new digital technologies is enabling JM to enhance its existing services, from on-site surveys, reformer balancing and troubleshooting, to remote catalyst evaluations, sample analysis and benchmarking. It is also providing new ways for customers to interact with JM.
JM is in the process of developing and launching a range of new and improved technical service offers in three areas:
Specialist measurement and analytics
JM offers services in enhanced measurement and monitoring. An example is its ReformCMS offer, in which advanced steam reformer modelling capability, developed over many years as a catalyst supplier and designer/licensor of steam reformers, is used in conjunction with advanced measurement techniques to provide a much more accurate picture of reformer performance than is possible when using such tools independently. A remote monitoring interface allows JM to generate focussed recommendations for improving reformer performance as often as is necessary, thus allowing customers to increase throughput, reduce energy consumption, increase tube life and reduce NOx emissions.
Remote monitoring and dashboards
JM provides direct connections to plant historians and the potential for reports to be accessed remotely via a portal or dashboard. JM is able to deploy a range of secure connection options, suitable to each customer’s particular environment and standards, including direct links or periodic file transfers. As well as allowing automated visualisation and generation of recommendations for plant and catalyst optimisation, the benefit of these solutions is to facilitate a richer exchange of information between the customer and JM. This means that JM engineers have easy access to a customer’s latest data and can provide support and advice with a faster turnaround as less time is required for data collection and exchange.
Improved service for our customers
Finally, JM is working to introduce a range of new applications and tools to enable its engineers to have secure remote access to required customer information, historic knowledge and reports, links for reporting, as well as tools to aid safety on-site. These tools such as mobile applications, connectivity and communication tools are aimed at enabling engineers to provide better services on-site and help achieve JM’s commitment to the safety of all its staff. JM is also launching tools to ensure more effective risk and safety assessments, simplified data reporting and to streamline communications between staff and with customers. Furthermore, JM has a range of webinar capabilities to ensure that training services can be provided from anywhere in the world.
The pace of digital innovation means that JM is continuing development across all the markets that it serves – new solutions are in development with customers and partners that aim to improve the experience of customers, reduce effort and time, and improve connectivity between JM and its customers. JM is actively engaging in partnerships with customers to develop customised solutions that leverage the best of JM’s experience and technical expertise to meet specific customer challenges.
KBR TECHNOLOGY SOLUTIONS
Technical services and digitalisation solutions increase operational reliability in ammonia plants
You license a unit, commission it and start it up. The air is full of hope and expectations of performance and value delivered by the unit. Production targets are established and suddenly the startup turns into daily management and routine.
As the routine sets in, things often appear to work smoothly and can quickly lead to a position of comfort where most results are delivered, but also where we normalise small issues and stop challenging our companies, our teams and even ourselves to perform better. This can open the value breach between the maximum value a team and its assets can generate vs the actual value realised. The worst part is that this gap becomes invisible and, over time, it grows.
Adopting technical services and digitalisation solutions can make a dramatic difference. By effectively planning and managing the lifetime of the unit and recognising the various events that take place, value adding services and solutions, especially those provided by the licensor, can help plant owners and operators to quickly benchmark their performance in operation and maintenance of their unit, and to define the optimum way for achieving top performance and the way to close the value breach, in order to maximise returns.
KBR envisions the following four general areas where technical services and digitalisation solutions can add value:
- Fit for operation
- Operational reliability
- Profitable operation
- End of lifetime services
Fit for operation solutions and services help the unit become ready to be operated at top performance. They may include commissioning and start-up services, but also training services, operator training simulator (OTS) solutions, operation readiness reviews, safety, health and environment services, maintenance team readiness and even procedure reviews.
Operational reliability solutions and services make sure the unit performs at design conditions consistently and at target operating and maintenance costs. They include asset integrity and reliability reviews, asset management, reliability management solutions, maintenance management systems, equipment inspections and repairs, maintenance execution and turnaround planning and execution.
Profitable operation solutions and services help maximise the yields that the unit can deliver. Many units can operate at higher-than-design capacities. Hence, these services include studies to define how to do so in a safe and reliable way, studies to lower operation/maintenance and energy costs, increase quality, yields, production outputs, but also technologies that lead to reduced costs, such as remote process monitoring, preventive operating issue resolution, preventive maintenance, advanced process control and advanced simulations.
End of lifetime services help extend the lifetime of the asset, for instance revamps and retrofits and eventually services related to the decommissioning of the asset.
Discover, diagnose, design and deliver
KBR uses a unique methodology that is proven to deliver measurable valued solutions, which are designed to address complex problems related to plant operational issues.
The methodology has four major sections: discover, diagnose, design and deliver.
In the first step, KBR works together with clients to understand their business and operations strategy, profitability targets and possible improvement scenarios. After agreeing on the priority of the identified improvement scenarios, KBR selects the one to work with and move into the second step.
In the second step, KBR collectively elaborates on how the selected scenario will address the desired improvements and generate the potential value.
In the third step, KBR evaluates all possible solutions to the problem and provides guidance to identify the parameters of the solution. The third step concludes with the selection of the solution, which will deliver the expected value.
The fourth and final step is the implementation, ensuring the promised value is delivered, is adaptable and can be sustained by operations. This includes solution adoption, adjustment to the corresponding business processes and the required training and change management programs, which will enable the organisation to work according to the implemented scenario.
Digitalisation solutions for operational reliability
Operational reliability is one of the key areas which impact the plant’s productivity and is directly linked to the plant’s uptime. This, in turn, is determined by the health of both the equipment and the process.
Operational reliability is the crucial factor in maintaining high profitability. High level of operational reliability implies reduced maintenance costs and less capital tied up as high spare parts inventory, both of which directly affect the plant’s bottom line.
In order to achieve high level of reliability in terms of asset and process performance, and to ensure that the plant operates safely and efficiently, the maintenance practices must be driven by sound reliability-based business processes and accompanying solutions.
The advances of technology offer the opportunity to plant owners and operators to apply different approaches to the management of the plant, the process and the operations, which can have significant benefits in terms of safety, efficiency, plant uptime and eventually increased profits.
Digitalisation is the adoption of new business practices and technologies and their incorporation into the operations and plant decision making, with the purpose of increasing the value generated by the assets, by pushing the operating points to new areas and maintaining them safely there.
The digitalisation solutions for operations monitoring and management are currently in a mature state due to:
- advances in data acquisition and data processing;
- availability of advanced modelling and simulation techniques;
- availability of complex and robust process models;
- commercial software using artificial intelligence (AI) and machine learning (ML) techniques;
- availability of cloud-based solutions and the industrial internet of things (IIoT);
- mobile devices;
- 3D visualisation tools;
- virtual reality equipment and software.
A comprehensive digitalisation solution uses business and process data to learn from the past process and equipment behaviour, to analyse the current indicators and understand why the plant and process behave as they do, to prognose what will happen in the future and to support the operations and business management teams to make the best decision proactively (Fig. 1).
It should be noted that the digitalisation solutions offer additional value when they combine seamlessly process knowledge with cutting edge technology and sound accompanying business processes, adapted to the business and plant environments.
Configuration of a software alone does not guarantee value.
KBR discusses below two digitalisation solutions, which ensure increased operational reliability and help clients reap maximum value from their existing assets. These are the reliability-based maintenance (RBM) solution and the performance monitoring and advisory solution, KBR InSiteSM , which together form the basis for condition-based practices.
By embedding an artificial intelligence/ machine learning (AI/ML) module the condition-based practices can be progressed to more predictive and prescriptive operational strategies.
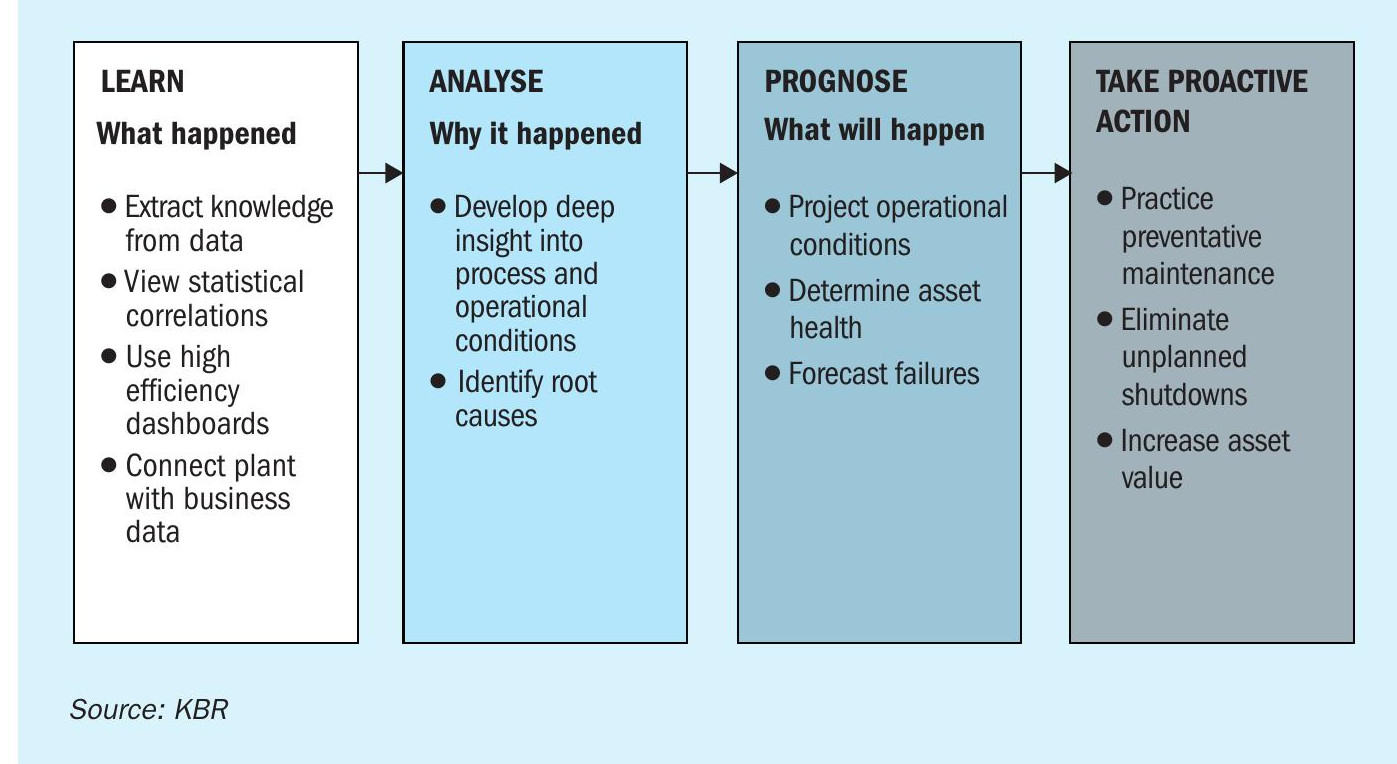
Among the digitalisation solutions available in the market today, reliability-based maintenance solutions, such as the one KBR has designed and developed specifically for ammonia plants, accompanied by best in class relevant business processes, offer great value to customers and have high return on investment, because they address the core issues of operational reliability and help clients increase the value generated by their assets.
Reliability-based maintenance minimises and even eventually eliminates reactive maintenance, resulting in significantly lower maintenance costs and lower inventories of spare parts. When the plant experiences less reactive maintenance, both unplanned maintenance-related downtime and labour costs decline.
The KBR RBM solution has modular functionality and optimised business processes that enable best practices. It helps clients improve the performance of their reliability and maintenance practices and maximise their asset’s return on investment (ROI), by transforming the maintenance operations from reactive to proactive.
KBR’s RBM solution is fully integrated with the plant distributed control system (DCS) and the client’s business system and provides scalability for growth in terms of users, facilities, and assets.
KBR’s RBM solution is offered with the following modules:
- Maintenance management
- Document management
- Asset performance management
- 3D asset visualisation
- Field data collection
- Real time historian
Fig. 2 shows the functional block diagram of KBR’s RBM solution.
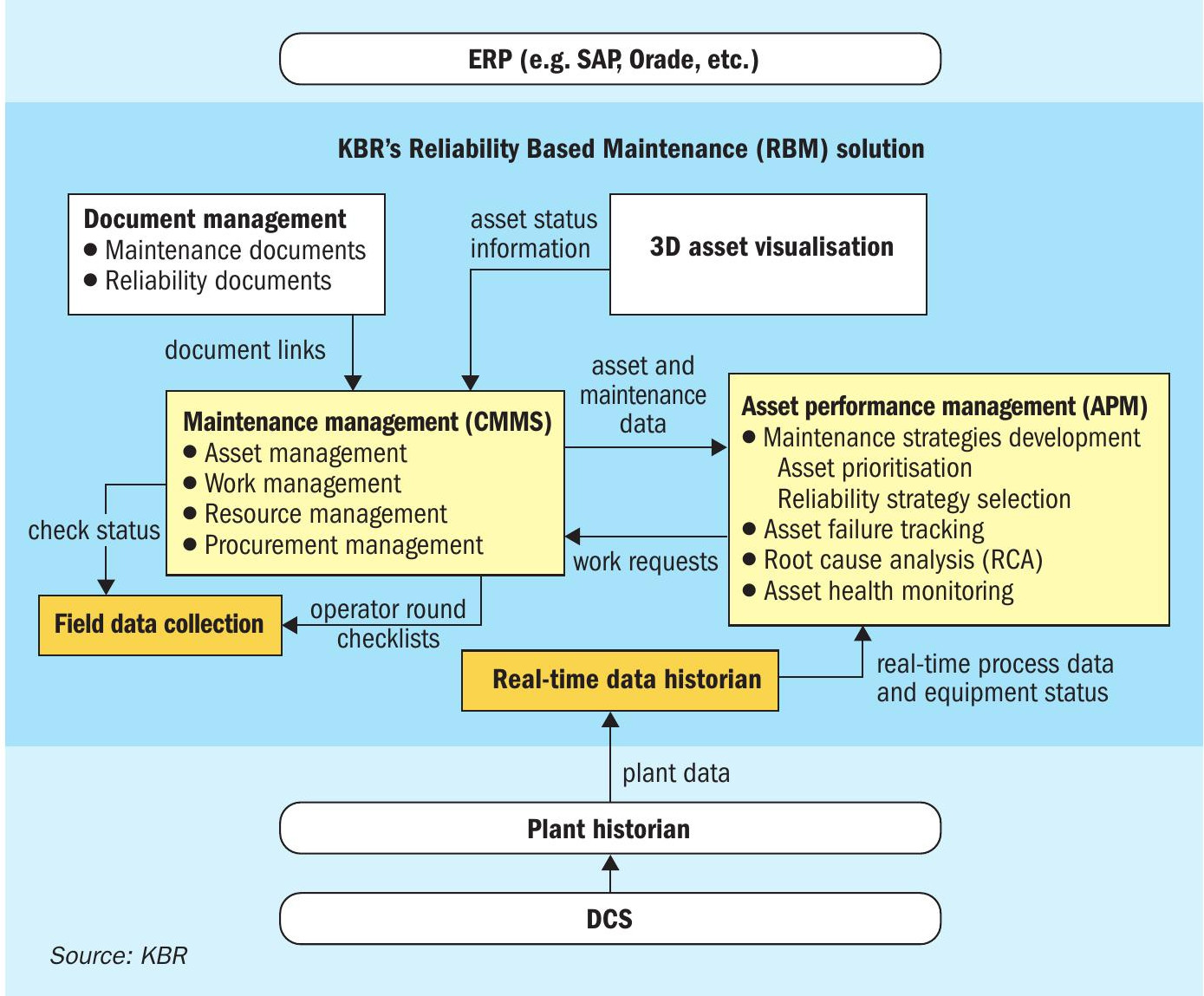
The RBM solution enables proactive monitoring of assets and automatic notification of performance issues, so that the plant’s operations and maintenance management teams can take corrective actions. Benefits are generated due to the direct reduction of the overall plant maintenance requirements and associated costs, reduction of unplanned shutdowns, increase of plant uptime, reduction of overall production costs and improvement in energy consumption. Continuous performance management will ultimately yield improved safety, higher product quality, increased reliability and availability of the company’s assets, which have a direct effect on profitability, while at the same time will help manage risks and compliance to regulatory requirements.
KBR InSiteSM
KBR InSiteSM is a cloud-based technology licensor service for remotely monitoring plant process performance and the health of associated critical equipment. KBR InSiteSM uses a combination of state-of-theart web-enabled visualisation dashboards and advanced mathematical algorithms to observe, track, and analyse operational parameters and key performance indicators (KPIs) that impact the overall efficiency and productivity of the plant.
At the core of this service is the availability of a pool of KBR process and operations experts who leverage their in-depth technical know-how of the process to provide timely guidance to plant personnel to support improvements in plant operations and equipment reliability. The process experts use the KBR InSiteSM dashboards as an enabling platform to visualise and diagnose operational problems, determine probable root cause, and recommend corrective actions that can either prevent abnormal operations or enable quick resolution of current problems. Drill-down features allow the rapid visual identification and exploration of potential problem sources.
Proprietary calculation methods and first principle-based simulation models unlock the value of near real-time plant data to create a visual “digital fingerprint” of the plant and provide the necessary framework to estimate the performance and health of process and rotating equipment. Inherent or derived parameters such as exchanger heat transfer coefficients, compressor efficiencies, specific-energy consumption, approach to equilibrium, etc. are examples of parameters calculated periodically by these first principle models. Moreover, these models can be used to periodically reconcile the overall heat and material balance of the plant so that inaccurate or faulty sensors can be flagged for recalibration or replacement. Offline, what-if analyses can also be conducted using these models, to identify opportunities for production increase or energy reduction by driving the plant operations towards the upper limits of thermodynamic efficiency (subject to process or equipment constraints).
Smart, proactive, escalating alerts to flag deviations of KPIs from as-designed or targeted performance are setup to trigger advisory service and the proactive engagement between plant personnel and KBR’s experts. These alerts act as early warning notifications of impending abnormal or sub-optimal process deviations, they can be visual (displayed on the dashboards) and can be propagated as text messages via e-mail or to a mobile device. Any data analysis related to these alerts as well the recommended follow-up actions are summarised in Event Reports that are sent to the customer.
This mode of alert propagation and expert analysis based on early event detection clearly reflects a paradigm shift from a conventional, reactive form of service to a more proactive form. This prevents incipient issues from progressing into serious operational problems that could negatively impact plant uptime.
In essence, the KBR InSiteSM service embeds the key functionality required to estimate the current health of an asset, to detect early events that can impact this health, and the expert guidance to prevent or mitigate abnormal events from leading to unplanned plant shutdowns.
Integrating KBR InSiteSM and RBM
KBR’s experience with process plants is that the type of root cause of unplanned shutdowns varies depending on the age of these plants. For instance, in brownfield plants most unplanned shutdowns are directly caused by equipment failure, usually as a consequence of gradual wear and tear associated with the operation of such equipment. On the other hand, at grass root plants many of the unplanned shutdowns are attributed to uncontrolled process upsets, failed sensors, abnormal weather conditions, errors in trip-logic, and in some situations due to operational oversight of inexperienced operators. To address both these diverse situations, KBR has designed the integrated InSiteSM and RBM solutions, as part of our integrated operations management and performance monitoring offering.
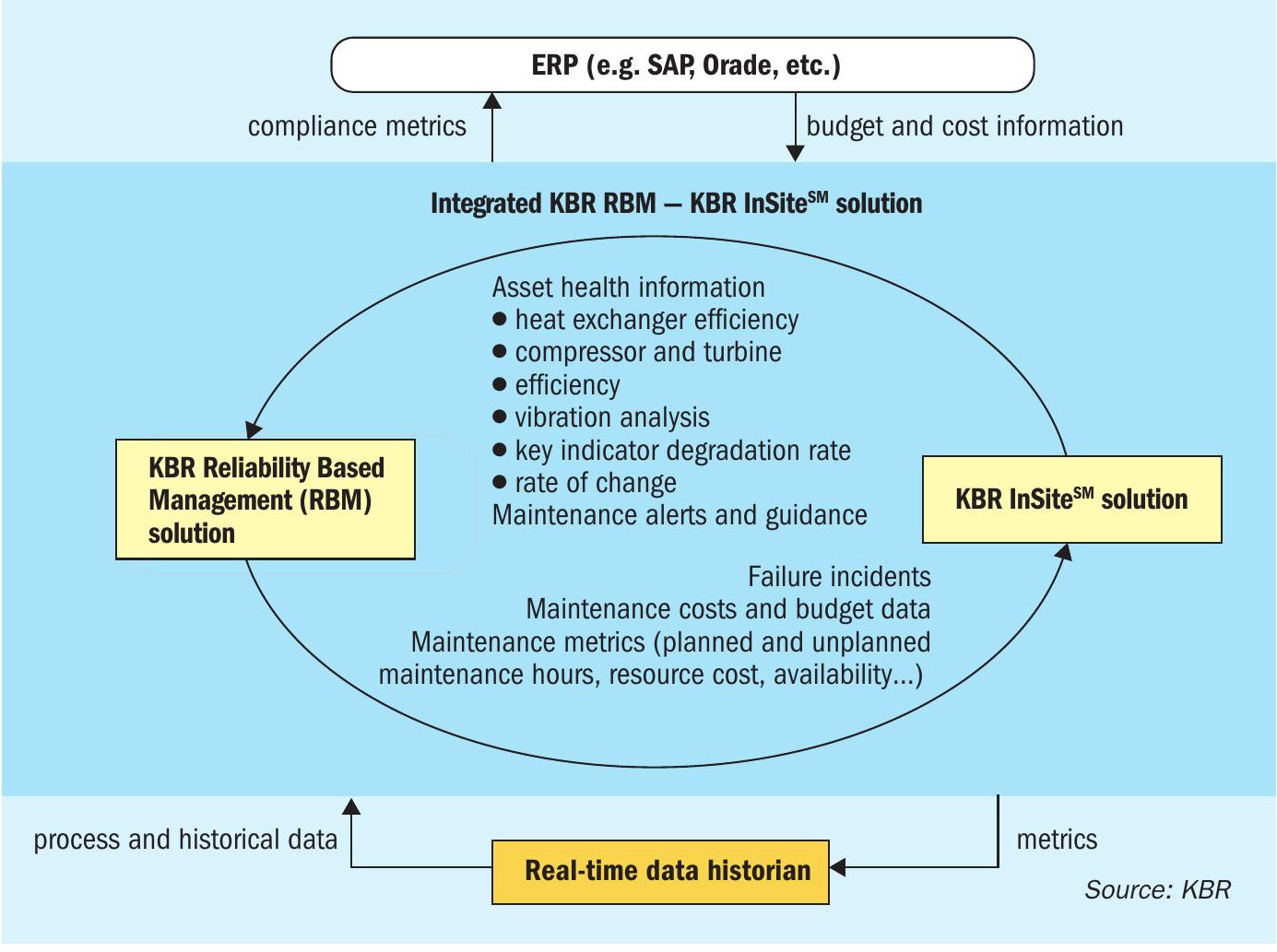
A schematic of the functional integration of the two solutions is shown in Fig. 3.
The combined solution enriches RBM with predictions and alerts regarding the health of the process equipment based on derived parameters (i.e. not available directly from sensor values in the control system), such as heat exchanger fouling, compressor efficiencies, and catalyst activity all of which can impact plant uptime. Moreover, it provides the flexibility to consolidate all the reliability management and maintenance management KPIs into the interactive dashboards of KBR InSiteSM , thereby offering KBR clients a centralised mechanism to track and review overall health of the asset.
Furthermore, the integration of RBM with the maintenance management module of RBM provides the ability to use the current health of the process equipment to support condition-based maintenance practices, enhancing further the ability of the combined solution to improve plant uptime.
Together, the integration of KBR InSiteSM with RBM and the maintenance system forms the basic building block for the deployment of a wider digitalisation program, which includes the use of AI/ML modules (described below) as well as the development, alignment, and optimisation of business processes and organisational capabilities needed to effectively implement and utilise the various technologies that comprise the overall digital solution.
Artificial intelligence and machine learning
An emerging global mega-trend in the process industry today is the use of artificial intelligence and machine learning for analysing plant operations data and predicting process anomalies and equipment failure. These applications typically use data from disparate sources (such as process historians, and laboratory and maintenance systems) to provide early detection and identification of incipient issues that can potentially lead to undesirable process excursions or equipment failure in an operating plant.
Moreover, the ability of such data-driven models to predict ahead of time when these process excursions or equipment failures will occur, allows a plant operator to take proactive remedial action to prevent an uncontrolled process excursion that can result in an unplanned plant shutdown. Similarly, the prediction and analysis of time-to-failure of equipment can help the operations and maintenance teams to plan and schedule maintenance activities ahead of time as well as initiate actions for on-time delivery of spare parts to reduce production downtime.
Therefore, for KBR the embedding of AI/ML functionality within the InSiteSM and RBM applications represented a perfect opportunity to create a high-value solution that helps customers adopt prediction-based preventive maintenance practices that can reduce unplanned shutdowns and improve the uptime of operating plants. To meet these requirements, KBR designed an AI/ML module that would as a minimum provide the ability to:
- detect anomalies or events that indicate that a process or an equipment is behaving abnormally;
- quantify the contribution of different variables or parameters to these anomalies;
- predict ahead of time the value of a critical process variable that if uncontrolled can lead to a plant upset;
- forecast the time to failure of an equipment;
- provide prescriptive analysis or recommendations to resolve a process anomaly or to prevent an equipment failure.
From an end-user perspective it is critical that the diagnostics provided by the AI/ ML module in response to an anomaly are very visual and facilitate easy review of the main contribution factors. To further extend the functionality, KBR is developing a smart rule-based system that combines the AI/ML diagnostics with the domain knowledge of their experts to help identify not only the root cause of the anomaly but also the remedial actions required to prevent these operational anomalies from progressing into unplanned plant shutdowns.
Digitalisation and expertise
Reducing unplanned downtime and increasing asset utilisation represent the largest opportunities for reducing operational expenditure (opex) of a plant. Hence the ability to quickly diagnose and remedy operational issues which impact equipment performance is a prerequisite for achieving operational excellence in most plants. Based on KBR’s experience, the ability to combine digitalisation solution with domain knowledge of their process experts has provided them the opportunity to assume the role of a “Trusted Advisor” to plant personnel and achieve significant benefits for them in the various stages of plant life:
During commissioning and start-up: As a detailed engineering contractor, KBR has provided commissioning and start-up teams for onsite service while being supported by technology advisors from the home office. To a large extent, the deployment of the KBR InSiteSM service has increasingly contributed to the safe and successful start-up of these plants. Specifically, the ability to remotely monitor plant operations in near real-time through the visualisation dashboards has allowed KBR experts located at different engineering offices to collaboratively shadow commissioning and start-up activities and alert the onsite team of any deviations from best practice guidelines. Moreover, it allows the validation of any operating guidelines that are recommended to plant personnel from the home office through the use of dynamic simulation programs which are developed to closely mimic transient conditions normally observed during start-up of the various process units.
Normal steady state plant operations: Continuously improving normal steady state operations of a plant through timely expert advice based on proactive monitoring and data analysis is a primary benefit derived from the KBR InSiteSM service. The use of proprietary mathematical and optimisation tools combined with subject matter expertise helps identify how the plant can transition from a current steady state operation to a more optimal one.
Unscheduled plant shutdowns: The use of AI/ML tools provides early detection and identification of anomalies and helps prevent incipient issues from progressing to process excursions or equipment failure. These tools can also be used against historical data to identify the root cause of plant shutdowns that occurred in the past. However, a well-tuned dynamic simulator could also be used to mimic the sequence of events that precede an unplanned shutdown, allowing the root cause of the trip to be analysed, controllers to be retuned, and the interlock logic to be modified and thoroughly tested to account for relevant process dynamics as well as operator action. Without the KBR InSiteSM service, the plant operators would have had to learn from each event after the fact and plan proper anticipatory response to address similar events in the future.