Nitrogen+Syngas 371 May-Jun 2021
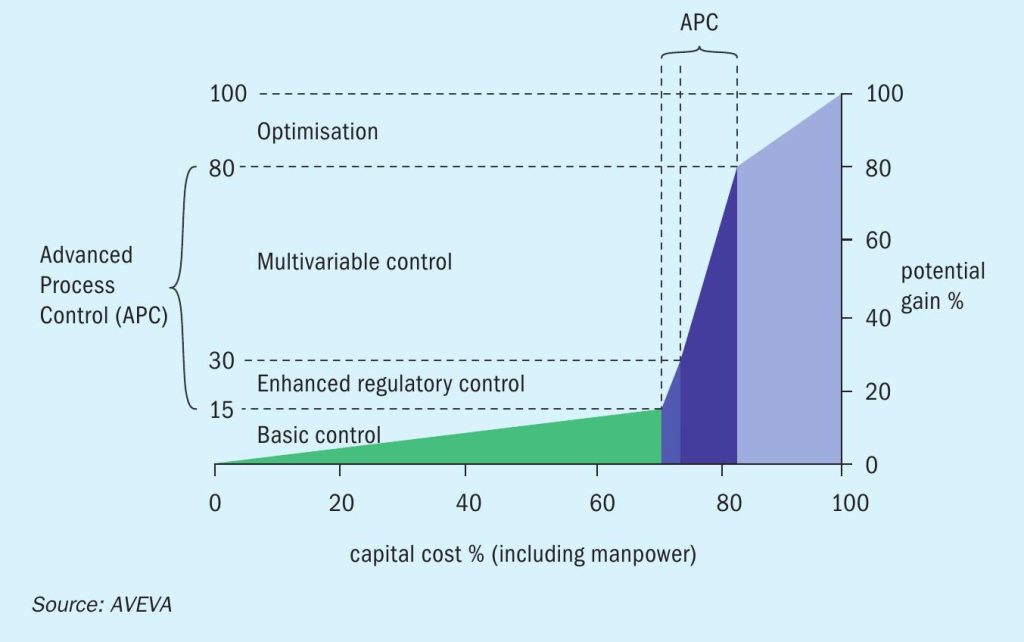
31 May 2021
Improving the process economics of ammonia plant operations
ADVANCED PROCESS CONTROL
Improving the process economics of ammonia plant operations
The ammonia industry has always dealt with fluctuations in supply and demand as well as volatile feedstock and energy costs. The unexpected global pandemic that started in 2020 has injected a higher degree of uncertainty for ammonia manufacturers’ operating costs and product demand for fertilizer. W. Poe of AVEVA discusses how advanced process control systems can help ammonia producers turn economic uncertainty into a competitive advantage.
In 2019, nearly 235 million tonnes of ammonia were produced worldwide with the fertilizer industry creating the greatest demand. The Covid-19 pandemic disrupted manufacturing activities and brought down the demand for ammonia. However, as economies are opening and the production of food and chemicals is rising, the demand for ammonia is expected to increase in the coming years2 . Coupled with that, 107 new ammonia plants have been announced to start up through 2030 to increase global production capacity to nearly 290 million tonnes by 20301 . As a result, ammonia producers will continue to face an uncertain and highly competitive market environment for the years to come. Older plants in particular will be challenged to approach the efficiency of the new builds.
The widely adopted Haber-Bosch process for steam reforming relies heavily on natural gas as the source of hydrogen. Ammonia synthesis for the fertilizer industry is a process that demands significant energy to react the chemical species that form NH3 . Since nitrogen gas is strongly held together by triple bonded molecules, a large amount of energy is consumed to operate the system at the high temperatures and pressure required. The synthesis of nitrogen and hydrogen into ammonia is an energy intensive step in the ammonia production process that only realises a 10 to 20% conversion of gas per cycle, thereby requiring considerable recycle of synthesis gas. Therefore, an ammonia plant generates the highest profitability when the hydrogen content of natural gas is converted most efficiently. As a result, the biggest impact on ammonia plant profitability is maximising yield at the lowest possible energy consumption per tonne of ammonia produced.
Advanced process control
A study conducted by the Automation Research Council found that only about 15% of available automation benefits for manufacturing plants are captured with basic control systems despite the basic control system accounting for about 75% of the cost of automation. There is typically an additional 85% of ultimate automation benefits available with high return on investment for advanced process control and optimisation applications (see Fig. 1). A multivariable model predictive control also known as MPC presents the best cost-benefit relationship among all the automation layers.
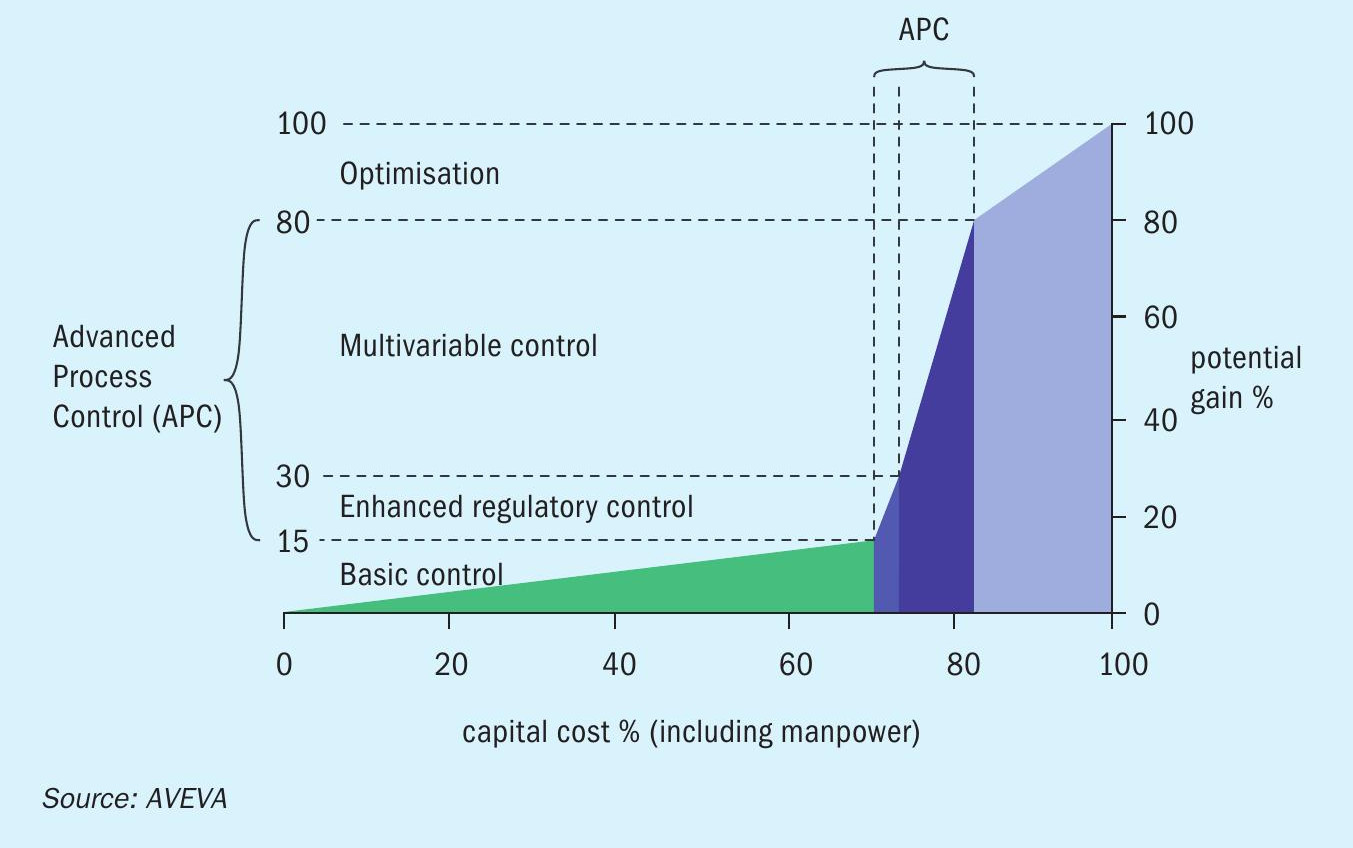
Multivariable model predictive control achieves benefits by reducing variability in the outputs of an operation such as quality, production volumes and operating costs by identifying the sources of variability and responding to these disturbances (Fig. 2). This technology predicts the movement of the process and considers the operating targets and constraints as well as equipment limits that must be honoured for safe and reliable operation. With these predictions the technology can continually push the plant towards optimal targets with reduced variability and higher reliability.
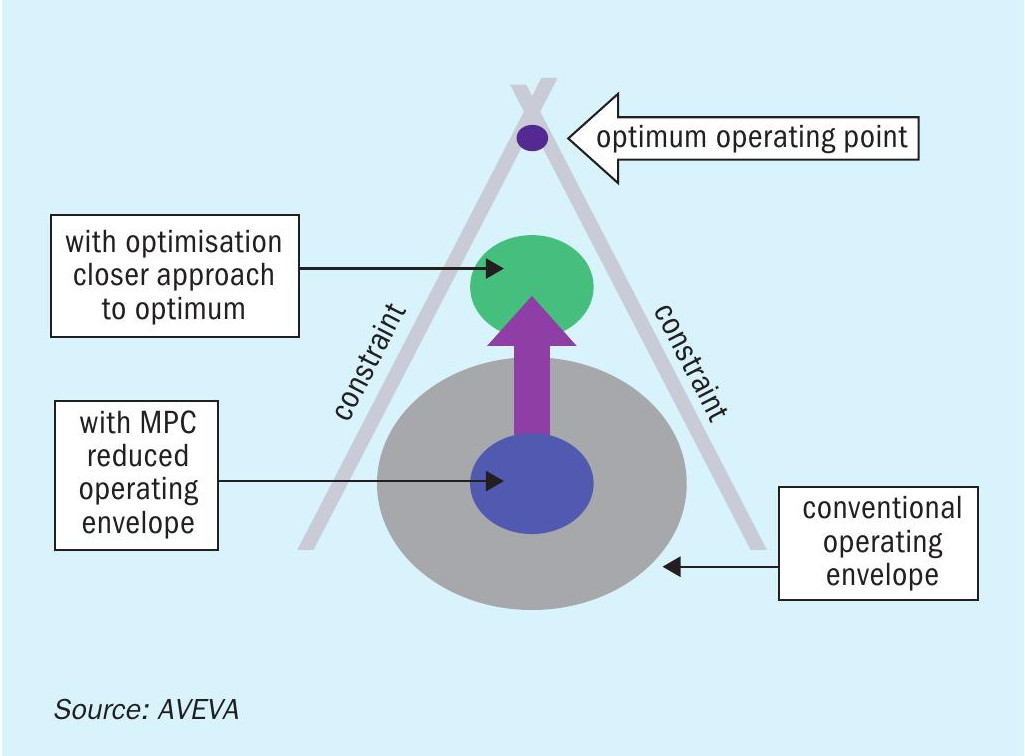
When operators try to push the plant towards constraints, they may encounter instability or exceed the constraint. To avoid that, they will back off to a comfortable operating point away from that constraint and typically keep the process there for the remainder of their shift. The algorithms of MPC allow more elegant handling of constraints. When the predictive models anticipate that a constraint will be encountered, it will take action to move right up to the constraint without exceeding it. The advanced controller will back off the constraint as conditions change, but only as much as necessary, and for as long as necessary. Then it will move right back up against the constraint.
By recognising and understanding the effect of different disturbances and set-points on constraints, the widest range of stable process operations can be accessed, quality is improved and operating profit is increased.
Benefits of multivariable model predictive control can be summarised as follows:
- allows operation to be closer to limits by reducing variations;
- moves the process closer to multiple constraints (limits) simultaneously;
- provides access to a wide range of process operations;
- improves quality;
- increases operating profit by improving key metrics such as production rates and specific energy consumption.
Another way to look at the impact of model predictive control is in relation to multiple constraints. Optimum operating conditions are typically at the intersection of constraints. With the process variability experienced with basic proportional-integral-derivative controllers, operators must select setpoints that keep the plant within the boundaries of multiple constraints and therefore a wide operating envelope. With the reduced variability of model predictive control, the operating envelope is reduced and the process can be pushed closer to the optimal operating point (Fig. 3).
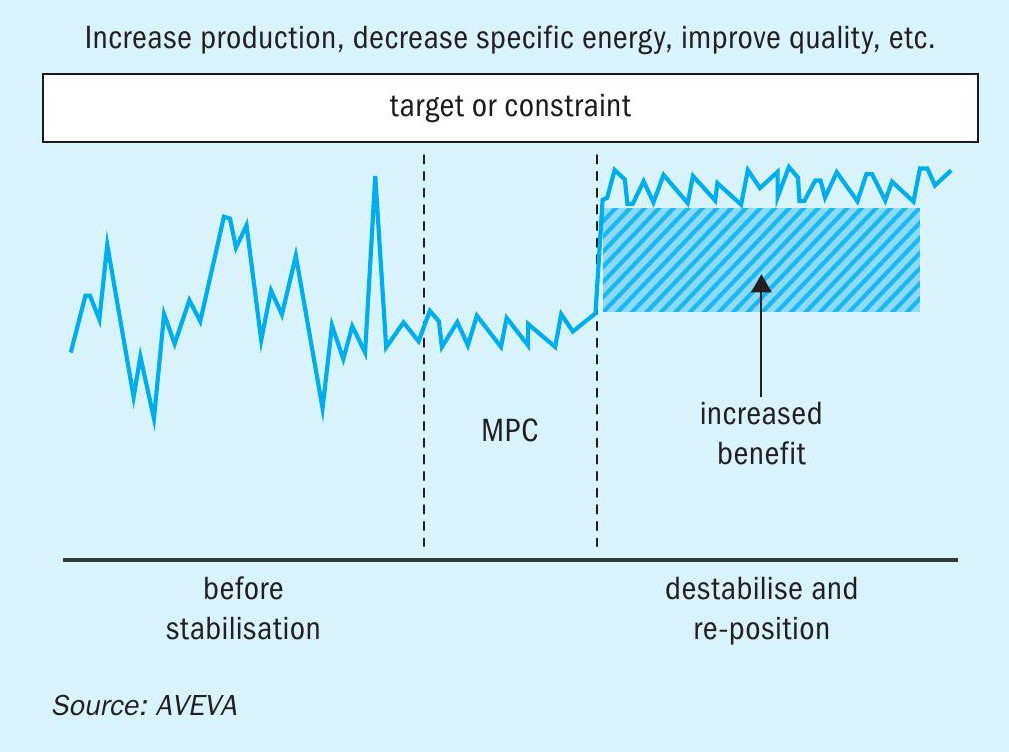
The model predictive controller contains dynamic models that describe the process responses, including time delays, interactions between inputs and outputs, and complex responses (inverse, integrating, etc.). Statistical methods are used to develop the predictive model relating input variables (disturbances and PID setpoints) and output variables (targets and constraints). As a result, the model can predict the future trajectory of the process outputs for any variation in the process inputs (Fig. 4).
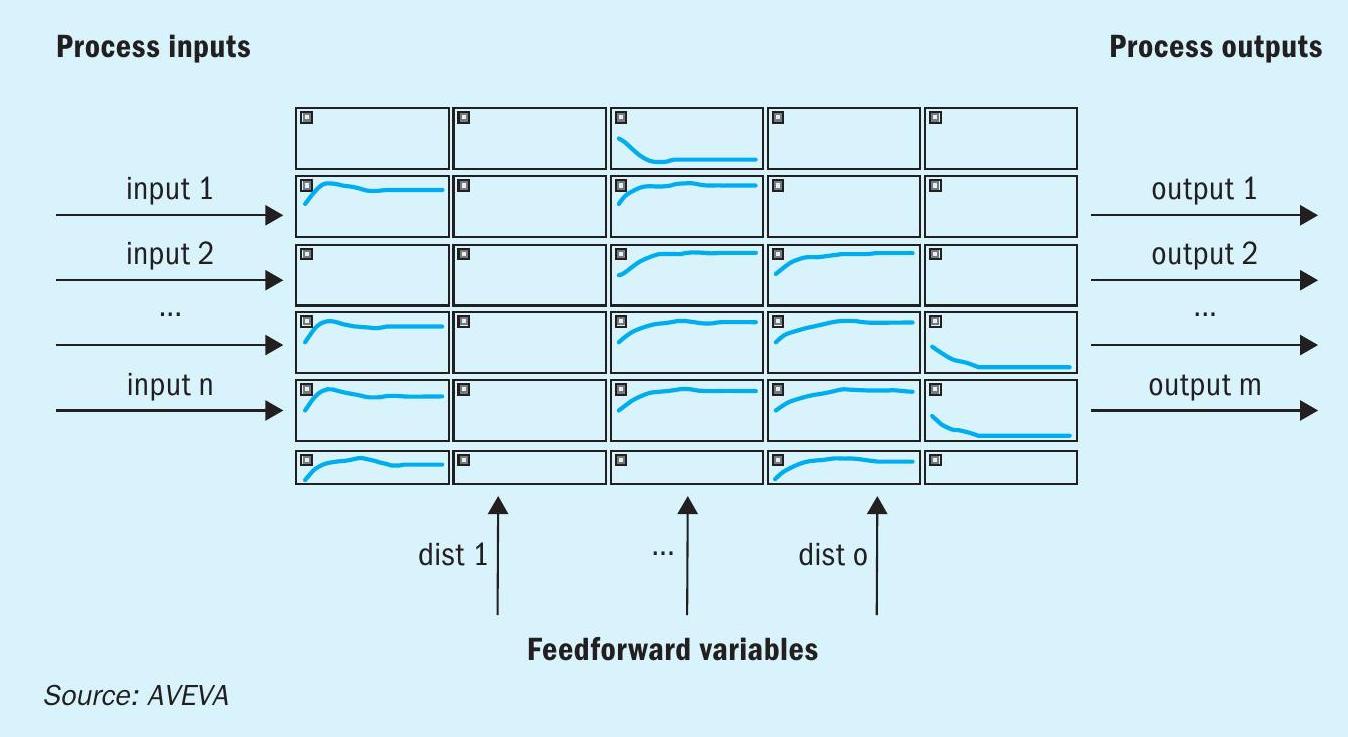
By predicting the future variations, these models can continuously determine the setpoint adjustments required now to maintain targets and honour constraints. The underlying mathematics are quite complex and even more complex if nonlinear relationships are included with neural networks. Predictive models are either determined from plant tests or from data captured by the plant historian.
A powerful aspect of these models is their ability to understand the impact that a change in set point of any controller will have on other outputs not under its direct control. Also, as constraints change the controller reacts instantly to the situation.
MPC applied to ammonia plants
The ammonia synthesis process lends itself nicely to model predictive control. The process has multiple reaction steps, high energy usage, interactions – especially in the synthesis loop with pressure, inerts, purge rate, large recycle and complex dynamics such as introduction of hydrogen at the primary reformer and nitrogen at the secondary reformer to control ratio at the synthesis converter inlet. A percent increase in production or decrease in specific energy consumption can substantially improve the profitability of the operation. Typically, MPC applications for ammonia plants will result in 1% to 2% improvement in specific energy, and 1% to 4% production improvements.
Model predictive control can be implemented for several ammonia applications, as follows. The primary and secondary reformers, synthesis gas loop and ammonia synthesis reactor typically have the greatest value. Depending on individual plant operation and bottlenecks, the other units may have a good return on investment as well.
- primary and secondary reformers;
- carbon dioxide removal facilities;
- hydrogen (purge gas) recovery facilities;
- methanation system;
- synthesis gas loop;
- ammonia synthesis reactor;
- shift converters;
- steam system.
Many of the typical advanced process control or model predictive control objectives for ammonia plants are listed below:
- key performance indicators (KPIs);
- maximise unit throughput;
- minimise specific fuel gas and steam consumption;
- drivers for KPIs;
- control methane slip and balance primary reformer outlet temperatures;
- control converter inlet H/N ratio and total inerts concentrations within pressure constraints and hydrogen (purge) recovery constraints;
- control synthesis converter temperatures;
- control refrigeration capacity;
- qualitative benefits;
- enforce all specified operating and safety constraints;
- stabilise the unit operation in the presence of unmeasured disturbances;
- reduce operator workload.
The first two are overall objectives of the plant that are key metrics for the value that is generated with advanced process control. The next few are some of the key drivers to improve the overall key metrics. The final three are more qualitative outcomes. Note that any application should obey the process equipment operating constraints and certainly not compromise safety.
Stabilising the unit in the presence of unmeasured or seldom measured disturbances such as ambient temperature and pressure, feedstock changes and others help the controllability of the entire complex. It has also been proven to assist in faster unit start-up. Plant operators have many assigned tasks, including continually tweaking basic control setpoints values. This type of activity adds tension to the operators’ daily work as many of the required adjustments are counter intuitive or need action much faster than the human brain can understand and execute. This type of solution helps the operators to better understand process behaviours, improving their effectiveness, and ultimately leading to higher job satisfaction.
Case studies
Case study 1
Case study 1 is based on an application deployed in an ammonia plant operated by a major producer in North America. This plant started the journey on using APC with successful pilot projects on a urea desorber plant and a sister plant for the entire ammonia plant. After conducting a study to determine the potential benefits and cost, a project was initiated focusing on the front end of the plant for feed maximisation and energy savings. The project yielded savings of about $750,000 per year due to ammonia production improvements and specific energy reduction. Payout was less than six months. The application has been in operation since 2005 and is being expanded to the synthesis loop and the urea plant.
The challenge
Improve utilisation and sustainability of urea desorber and ammonia units that produce 535,000 t/a of ammonia and 680,000 t/a of urea.
The solution
Use model predictive control for:
- steam to gas ratio control;
- excess oxygen control;
- feed maximisation strategy.
The benefits
- ~$750,000 per year in energy savings at industry average gas prices and energy consumption;
- 0.75% improvement in energy on a million Btu/t basis.
Case study 2
Case study 2 is based on an application deployed in an ammonia plant from another major producer in North America. It pertains to a site that desired to maximise ammonia production while enforcing operating and safety constraints. There were secondary objectives to reduce operator workload and specific energy consumption. A study was conducted at the site that included the cost and benefits of upgrade to a distributed control system and the identification of advanced process control benefits to justify the distributed control system installation. This study led to the installation of a distributed control system along with an extensive ammonia plant application that yielded 3-4% increase in ammonia production. The application was shortly thereafter expanded to all areas of the urea plant. In 2015 the plant capacity was expanded with a Kellogg Reforming Exchange System and the ammonia plant application modified accordingly. Advanced process control for the nitric acid plant is underway and they are also exploring opportunities for first principles-based optimisation.
The challenge
- maximise ammonia plant throughput and enforce specified operating/safety constraints;
- reduce operator workload and specific fuel gas and steam consumption.
The solution
Use model predictive control for:
- hydrogen to nitrogen ratio
- syngas suction pressure
- air compressor speed
- ammonia synthesis reactor temperature
- steam to carbon ratio
- feed flow control
Benefits
- 3-4% increase in ammonia production (in operation since 2006);
- expanded to urea plant, modified with KRES addition and now being installed on the nitric acid plant.
Improving benefits beyond the MPC
As mentioned before, the MPC is the main contributor to benefits related to plant automation. The MPC is also an important foundation to further optimise the ammonia plant. Once the process is stable through MPC, complementary models can be used to further optimise operations.
While the predictive models used for advanced process control are empirical dynamic models, first principles-based models can be added to the automation architecture. The most common first principles-based models used for online applications are process performance monitoring, including adaptive reactor models and real-time optimisation models. They are continuously tuned to close the mass, energy and equilibrium balance, optimising operations according to the desired objective function, while keeping variables within the physical, operating and economic constraints. Typical objective functions for real time optimisation are minimising energy consumption or directly maximising plant profitability.
Another modelling approach that can be used is predictive analytics for equipment reliability with machine learning. In this case, the model is built based on historical data and can include process variables as mechanical variables.
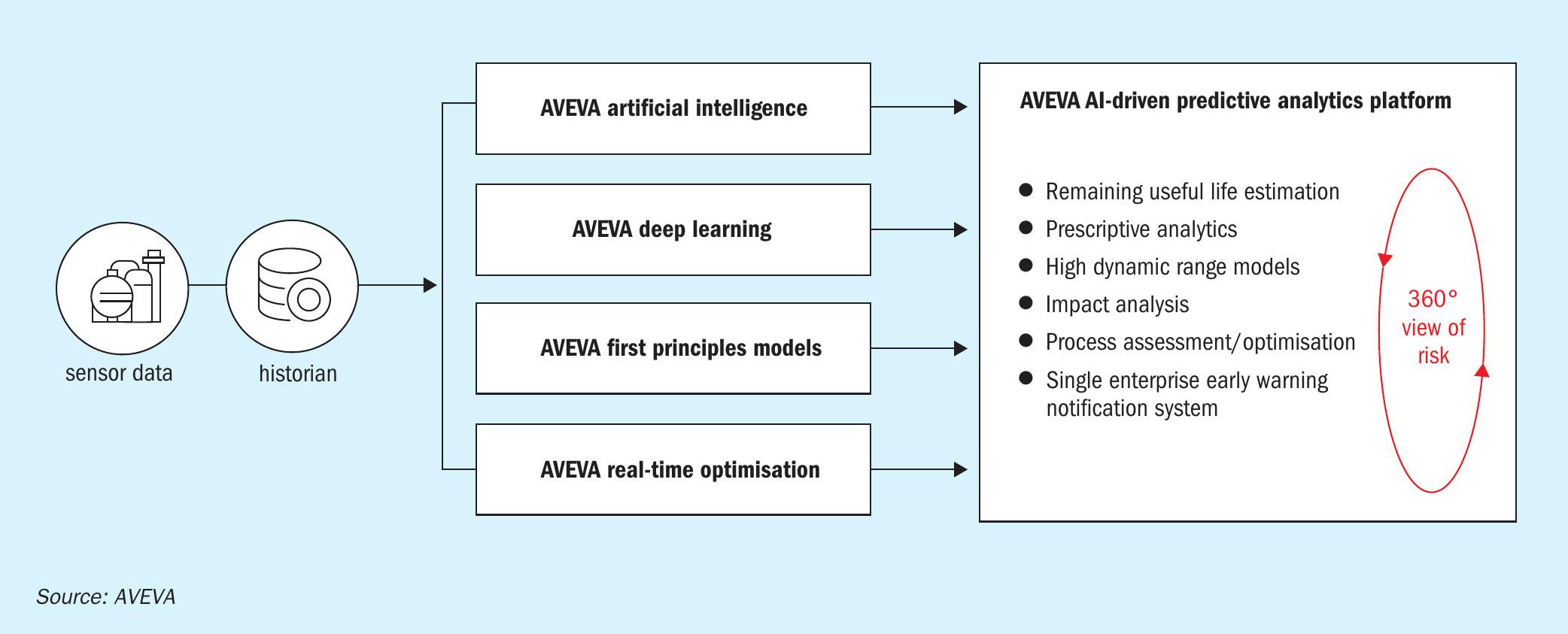
The latest advancement in models for online applications is artificial intelligence (AI) driven predictive analytics, that combines AI such as machine learning, deep learning with artificial neural networks and rigorous first-principles models, and real-time optimisation (Fig. 5). AI-driven predictive analytics is infused with results from the rigorous process simulation and optimisation algorithms, creating a sophisticated model capable of providing advice about the most cost-effective choices for operations and maintenance based on a 360° view of risk.
Summary
Basic control systems capture about 15% of the potential automation benefits at about 75% of the total automation costs. Advanced process control captures the most automation benefits with a high return on investment, and MPC is the main contributor to automation benefits.
There are many cases of the proven, sustainable benefits for ammonia plant operations. The main measurable benefits are production improvements of 1% to 4%, and 1% to 2% reduction in specific energy.
Other automation opportunities for nitrogen fertilizer plants include:
- first principles-based model for process performance monitoring;
- first principles-based model for real time optimisation;
- predictive analytics for equipment reliability with machine learning;
- AI-driven predictive analytics, combining AI, deep learning, rigorous first-principles models, and real-time optimisation.
References