Nitrogen+Syngas 387 Jan-Feb 2024
31 January 2024
Process optimisation with AI – hype or reality?
PROCESS OPTIMISATION
Process optimisation with AI – hype or reality?
Lisa Krumpholz, Managing Director of Navigance, delves into the world of artificial intelligence (AI) and discusses its potential to enhance process optimisation in syngas plants.
Syngas plants are vital for the synthesis of crucial industrial products like ammonia, methanol and hydrogen. Engineers working in these plants need to take decisions and have to provide answers to many different kinds of questions in their day-to-day operations: How can the plant meet its production target while optimising the energy usage within the plant to reduce costs and environmental impact? Does the heat distribution in the primary reformer need adjustments to ensure a low methane slip and good reforming efficiency? Is the methanol synloop operated optimally to maximise production? Is there any room to improve the CO2 removal capacity? Is the syngas compressor operating well or are there any signs of anomalies? And engineers need to answer questions for taking a decision about the timing of the turnaround window and catalyst replacement: How will the performance of the low temperature shift catalyst develop until the next turnaround? Is it possible to delay the turnaround by a few months and what is the economic impact?
To make the right judgements, experienced engineers today already rely on analysis of their plant’s operating data using specific models and tools. Will and can artificial intelligence (AI) be a game changer?
The rise of generative artificial intelligence (GenAI)
It’s a little more than a year ago that a technology based on large-language-models (LLMs) created a massive hype about the opportunities of generative AI in our working lives. On 30th November 2022, OpenAI launched their chatbot ChatGPT. It is a tool that enables every user to steer a humanlike chat conversation with a very simple user interface, while the underlying model responds to questions and can create many types of written content, including explanations, articles, emails or software code, based on the user’s input.
Since then, numerous GenAI models and tools have been developed and launched by various companies. No matter whether it is LLM-based GenAI or Textto-Picture AI or others, they all have in common that their foundation are powerful machine learning models that are running in the background. These models are enabled by two essential factors: Firstly, they are based on a vast amount of data from many sources. That includes everything that is generally available on the internet, but also specifically developed databases for research like ImageNet, a database of labelled and sorted images. Secondly, what may seem to the users now as magiclike output, required countless iterations of model training and model refinement.
The GPT in ChatGPT stands for ‘Generative Pre-trained Transformer’ – describing the architecture of OpenAI’s groundbreaking chatbot and it includes ‘training’ as an essential element it its name. A fundamental component of GPT, the transformer architecture was published in a paper in June 2017, while OpenAI released GPT-1 in June 2018. This initial model contained 117 million parameters. In only two years, OpenAI released the third iteration of its model, GPT-3, further improving its performance with a thousandfold increase of parameters to 175 billion. This reflects the tremendous investments in its development and the speed at which it advances.
Challenges ahead for (Gen) AI applications in chemical processes
This leads to the question which role will GenAI play in the operation and optimisation of chemicals plants? When and how will GenAI help operators for example to fine tune process parameters to run an ammonia plant in the most energy efficient state for a desired production rate?
First some good news: There is lots of literature and information available on the internet related to chemical engineering and for syngas plants more specifically. For well-known problems GenAI tools like ChatGPT may therefore provide useful, generic answers, which help the user to get a better understanding of their problem. It does not necessarily tell how to solve it exactly (Fig. 1) and the user needs to verify the correctness of the information.
Yet, when it comes to analysing and making use of operating data from syngas plants – or any chemical plant for that matter – in an easy-to-use interface for an operator, there are several challenges to be solved that go far beyond the processing of language-based information from the public domain. Here are three significant areas that are a requirement to develop GenAI applications:
Data availability: One may think the chemical sector is in a good position to utilise its data for decision making. The use of control systems has been widespread since the 1960s, and a typical ammonia, methanol or hydrogen plant now has hundreds to thousands of sensors collecting huge volumes of process and performance data. Today, this data is used to help control the plant, generate reporting and – increasingly common in recent years – may also be stored long term to support troubleshooting and analytics.
However, compared to information needed to develop and train ChatGPT to its current capabilities, the availability of chemical process data is sparse, virtually nothing is generally available on the internet. The data is in the hands of the chemical producers, while even the largest companies in the industry operate only a few ammonia, methanol or hydrogen plants when looking at it in the context of real big data needed for the development of GenAI.
Modelling technology: The underlying models of GenAI are very complex and need to be developed for syngas applications. The complexity and high number of influencing process parameters in any ammonia, methanol or hydrogen plant requires highly specialised models.
For use in dynamic, real-time optimisation of chemical plants, hybrid process models are known to overcome limitations and disadvantages of both individual model types: a) traditional first-principles models and b) purely data-driven black-box models. As the name suggests, they are a middle ground between the other two modelling technologies. Conceptually, a data-driven model, such as a neural network, is used as a basis and extended with expert knowledge of physical/chemical principles through e.g. ‘feature engineering’. In essence, hybrid models combine flexibility and adaptability of data-driven models with the process specific fundamentals.
With sufficient data, hybrid process models can accurately describe the plant operation. Compared to first-principles models, they do not require that all the physical principles in measured data are ideally fulfilled but learn to describe the actual behaviour of the real plant, which may encounter heat loss in a pipe, an unevenly fired reformer or heterogenous catalyst activity in a reactor bed, to name a few examples. And this is a major point: each plant is different and has its own specifics. From the underlying process technology to details of the equipment and available sensor data – no two plants are the same. It means that any AI-based application for syngas plants needs to understand and capture these specifics to provide detailed information and guidance to the operator in any particular situation.
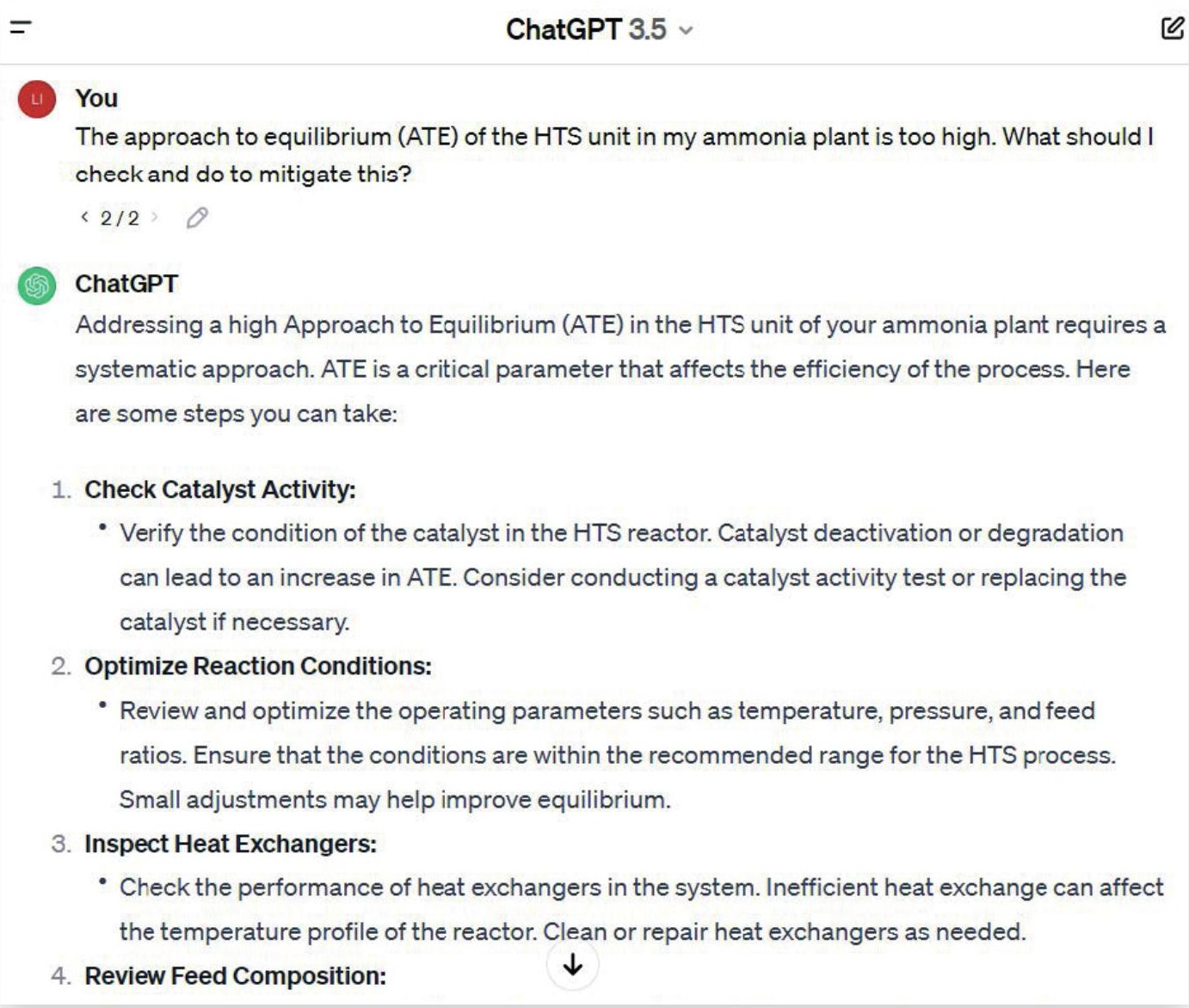
Accuracy and reliability: Hallucinations of large language model based GenAI – meaning responses presenting false or misleading information as facts – are a known problem, which developing companies are still tackling. The safety standards and zero-accident goal of the entire chemical industry, require high accuracy and reliability of information, especially when it comes to information that is used to conduct changes in operating conditions for optimising a plant or monitoring its performance. Therefore, there is no allowance for such ‘AI hallucinations’ in the context of steering an ammonia, methanol or hydrogen plant.
Real-time optimisation or monitoring of ongoing operations, in which current data is continuously fed to a model, calls for robust dynamic models that must cope reliably with changing operational reality. It requires that models are running ‘operationally’ and quickly ‘learn’ with the changing conditions. Therefore, any model for continuous monitoring and optimisation of an ammonia, methanol or hydrogen plant must be monitored for performance and quality, which is part of a process called machine learning operations (MLOps). It’s a discipline where the chemical industry can build on the progress and experience that has already been established over years in other industries.
In summary:
- Data availability is a challenge and making data available relies on the chemical producers operating ammonia, methanol and hydrogen plants. The first companies that will succeed in delivering a syngas process GenAI, will be companies that have access to sufficient process data from many different plants of a similar type.
- A pure data-driven approach to machine learning models for syngas plants is not proven successfully and on a large scale to date, limiting the advancements of any GenAI development. Hybrid models are capable to tackle this challenge and they will also become part of GenAI-like tools for process optimisation in the future.
- The reliability of models is essential. And well-proven approaches to run them on a large scale can be adopted from other industries.
Paving the way for AI and eventually GenAI in the syngas industry
A few years ago, Navigance started to invest in developing a capable and scalable technology to build and operate plant specific process models for ammonia, methanol and hydrogen plants. Navigance’s powerful, cloud-based platform today is operational for 100+ chemical plants. And it is suited to process data on a scale that will be sufficient to enable the development of GenAI in the future.
The other area of development is tailoring machine learning technologies to solve or support specific use cases and problems found in syngas plants. All solutions are built on hybrid base models which are specific to the ammonia, methanol or hydrogen process and then tailored with expert domain knowledge to the details of each plant and the desired optimisation goal or monitoring requirement. Here are some examples of currently implemented AI-based solutions in syngas plants:
Reformer optimisation
A common challenge for plant operators is to fine tune the reformer operation to minimise the methane (CH4 ) slip from the primary and secondary reformer, while keeping the hydrogen to nitrogen ratio at the reformer outlet at the desired ratio of the ammonia synthesis loop. These key performance indicators (KPIs) of the reformer section are influenced by multiple process variables such as the primary reformer outlet temperature, steam to carbon ratio, process gas, steam and air flows as well as temperature distribution in the reformer. Usually, the operator is guided to keep these process variables within a defined operating window. However, further finetuning of the setpoints for the variables within this operating window is left to the experience of the individual operator.
Navigance has developed an AI-based Interactive Reformer Optimisation tool for the reformer section, which is provided as software-as-a-service (SaaS) in a web-based interface. This tool comprises a hybrid process model tailored to the primary and secondary reformer of the specific plant. It utilises plant specific, machine-learning based data analytics for predicting the impact of changes to defined process variables on selected KPIs of the reformer section.
The Interactive Reformer Optimisation tool visualises the current operating conditions in context to the defined operating limits together with the KPIs of the reformer section. The operator can simulate “what-if” operational scenarios to determine the impact of changes to the process variables on selected KPIs of the primary and secondary reformer compared to the current operation of the process. Thereby the interactive tool enables the operators to define and evaluate options to improve process performance with a reliable prediction of the impact of operational changes to the reformer section KPIs. The underlying machine-learning based, hybrid process models are continuously processing the plant data and allow the plant team to learn from the plant’s behavior and to take informed decisions how to fine tune the reformer operation.
Catalyst performance prediction
A plant process engineer is often tasked to evaluate options and scenarios for determining the timing of economically attractive turnaround windows and take decisions on which catalysts to replace. Or when unforeseen issues such as catalyst poisoning or other incidents occur, to determine the impact and risk on meeting the next scheduled turnaround window for the catalyst replacement. This requires not only analysis of the current operating data but also a deep knowledge of the catalysts as well as specialised modelling tools to carry out projections. Mostly the engineer depends on the support of technical experts from the company providing the current catalyst in use.
Navigance has designed a solution with cutting-edge machine-learning based models for engineers to project the lifetime performance of specific catalyst types for different operational scenarios. Models for high-temperature or low-temperature shift catalysts are integrated into a web-based tool. It enables self-service catalyst performance projections of the catalyst in a hydrogen or ammonia plant based on current and historical operational performance of this plant. The development of these highly specialised hybrid models was made possible by using many data sets from more than 20 different plants and numerous catalyst loads totalling decades of operating data. Once tailored and trained with data from a specific plant, it allows the engineer to create multiple operational scenarios for projection of the HTS or LTS catalyst performance for time horizons from weeks, months to years. This supports informed decision-making for catalyst operation to meet planned turnaround windows based on economic considerations.
Outlook for the use of GenAI
At some point in time the currently already used hybrid models and the generated data and results will feed into Gen-AIsupporting models (based on Generative Adversial Networks (GANs)). It will still take a long time until GenAI models will be able to answer complex optimisation tasks, e.g. total energy consumption optimisation in an ammonia plant or even a CO slip optimisation.
On the other hand, the currently available AI-tools based on hybrid models can already perform these tasks when tailored to a specific plant. And they are also capable of reliably predicting or simulating the process behaviour in different operating scenarios.
The hype around GenAI tools, such as ChatGPT, lies in their ability to facilitate user interactions with underlying complex models through human-like communication. This has significantly contributed to a user-friendly experience, eliminating the need for specialised expertise. The first step towards a GenAI-like feeling for currently available AI-tools in the syngas industry will therefore be switching to an interaction with hybrid models in a chat-bot style rather than providing a user interface that demands lengthy data input and multiple clicks. Simplifying the interaction process can help to positively contribute to a wider adoption and acceptance of available AI tools in daily operation of ammonia, methanol and hydrogen plants and pave the way for even more powerful GenAI tools in the future. n
References