Sulphur 386 Jan-Feb 2020
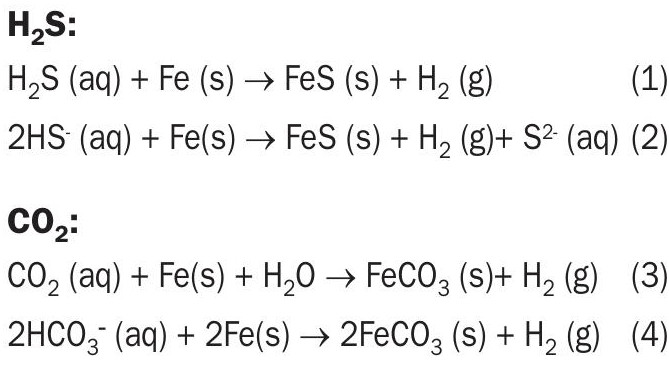
31 January 2020
Improve asset integrity by predicting corrosion
REFINERY GAS TREATING AND SOUR WATER SYSTEMS
Improve asset integrity by predicting corrosion
Using case studies of a refinery amine unit and a sour water stripper (SWS), U. M. Sridhar of Three Ten Initiative Technologies LLP, N. A. Hatcher and R. H. Weiland of Optimized Gas Treating Inc. demonstrate the capabilities of a mechanistic, chemistry-based, truly predictive model for calculating corrosion rates for various amines and for sour water. At a time when asset integrity is much sought after, the utility of this fully predictive model is to prevent failures before they occur, rationally select materials of construction, enhance plant safety, and mitigate risk.
Corrosion is a major concern in gas treating and refinery sour water systems, caused mainly by the acid gases CO 2 and H 2 S. One of the major factors influencing corrosion rates is the chemical activity of the dissolved acid gases themselves and the ions they form when they dissolve into the solvent. Activities depend on temperature, the amine type, its concentration, and acid gas loadings. Flow velocity, pipe roughness, heat stable salts, metallurgy, and suspended solids also affect corrosion rates.
This article highlights a mechanistic, chemistry-based, truly predictive model built using both proprietary and public corrosion rate data that can be reliably interpolated and extrapolated within and outside the measured ranges of the data. The model uses detailed speciation of dissolved acid gases, sheer stress at the pipe wall, metallurgy, and temperature to calculate corrosion rates for various amines (primary, secondary, tertiary) and for sour water over a range of temperatures, flow velocities, and loadings.
Acid gases and corrosion
The basic corrosion reactions of iron with H 2 S and CO 2 dissolved in water are:
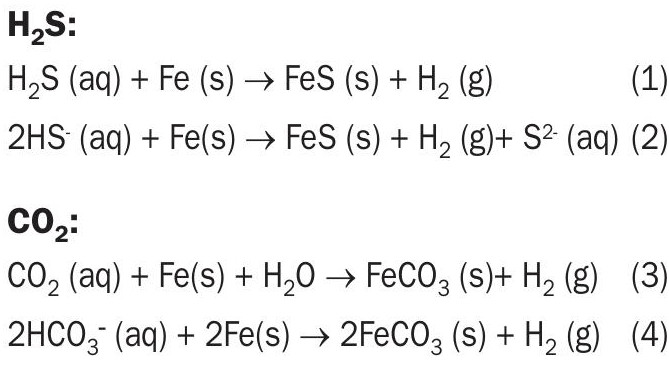
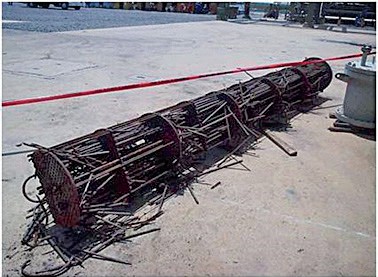
Bisulphide ion (HS – ), free physically-dissolved H 2 S, bicarbonate ion (HCO 3), and free physically-dissolved CO 2 are protonic acids. They are oxidising agents because during the oxidation of iron, they can either give up or produce a hydrogen ion. However, the final ionic reaction products, sulphide (S 2) and carbonate (CO 3 ) cannot produce the hydrogen ion necessary for oxidation. At the modest temperatures in most solvent treating and sour water systems, molecular H 2 S and CO 2 react with iron at appreciable rates only in the presence of water. “Speciation” refers to the equilibrium ionic and molecular species concentrations found by solving the chemical reaction equilibrium equations, atom balances, and a charge balance. Speciation of the solution is necessary in order to make sense of corrosion rates. However, the corrosion products themselves (i.e., solids) do not alter the speciation.
The concentration of free molecular H 2 S and CO 2 are pH dependent. In turn, pH is a function of total dissolved acid gases, amine type and strength, and temperature.
In general, free H 2 S and CO 2 oxidise iron faster than bisulphide and bicarbonate ions; however, their concentrations are usually very much lower.
Oxidation occurs in four steps (see Cummings et al 1 ):
- transportation of dissolved free acid gases and their ions (collectively acid gases) from the bulk solution to the metal surface;
- adsorption of the acid gases onto the metal surface;
- reaction of the acid gases with the metal;
- transportation of the reaction products back into the bulk solution.
Corrosion is ongoing because the reaction products FeS and FeCO 3 are always removed as solids, which drives the corrosion reactions forward. The results can be extreme (Fig. 1). The amount of unreacted iron and the concentrations of the reacting species (H 2 S, CO 2 , bisulphide and bicarbonate) are limiting reagents for the corrosion reaction. Metallic iron itself can be a limiting reagent because FeS and FeCO 3 deposited on the surface of the metal can shield it from contact with the oxidising agents. In practice, solution loading is generally limited to 0.4-0.5 mole per mole of amine in mild steel metallurgies because higher values cause more extreme corrosion rates.
In a newly built amine unit or one that has undergone a turnaround and been completely cleaned, initial corrosion rates will be very high because all metal surfaces are fully exposed to the corrosive solution. Over time corrosion levels off, then starts to drop as an iron sulphide layer occludes more and more of the surface, preventing contact between iron and the corrosive components. Once the iron sulphide layer is completely formed, the corrosion settles down to a nominal, hopefully manageable, rate, determined by the rate at which FeS is removed from the surfaces chemically and via scouring by suspended particulates. The solution changes colour through the different stages of corrosion from pale amber, through light and dark green, to nearly black. This is different from corrosion by CO 2 because CO 2 forms a more fragile iron carbonate layer that does not adhere well to the metal surface and is more easily sloughed off. Fluid velocity also affects corrosion rates by creating shear stress that tears away the sulphide or carbonate layers, exposing fresh iron surface to further corrosion. In practice, the rich amine velocity is limited to below 3–5 ft/s and lean amine velocity to below about 7 ft/s.
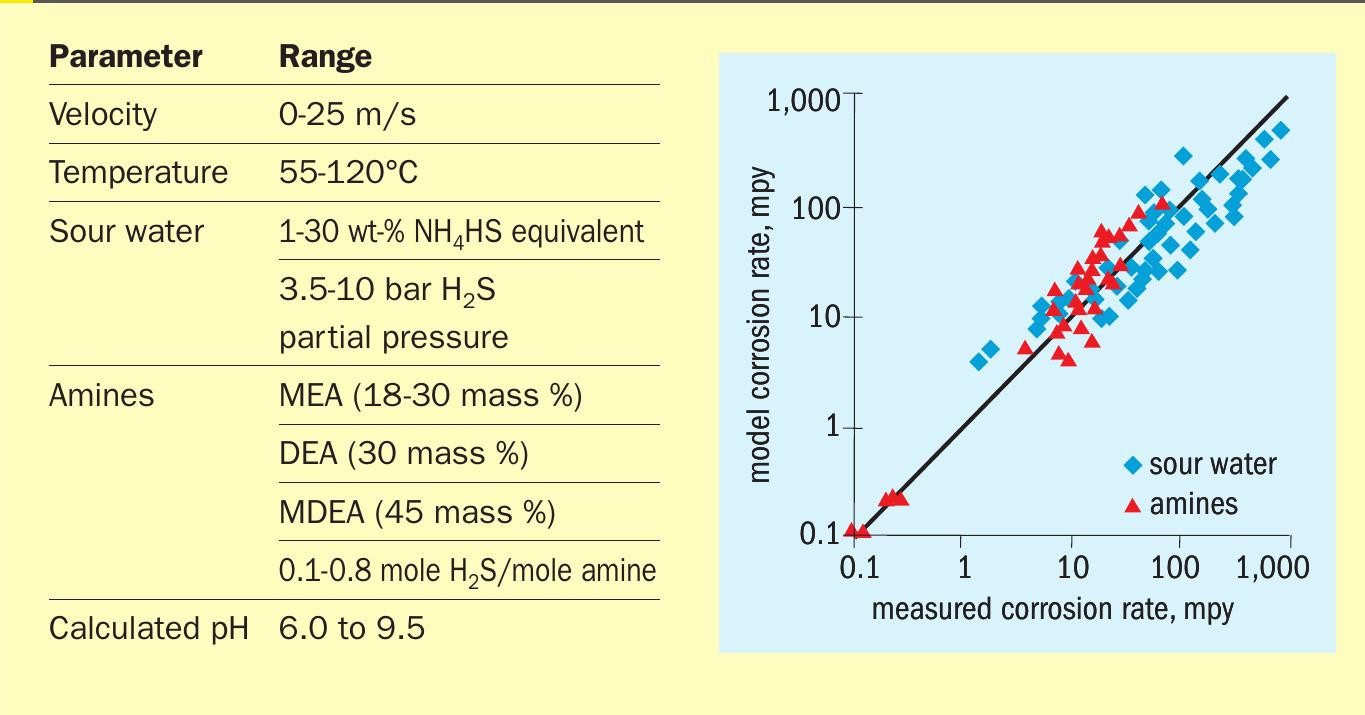
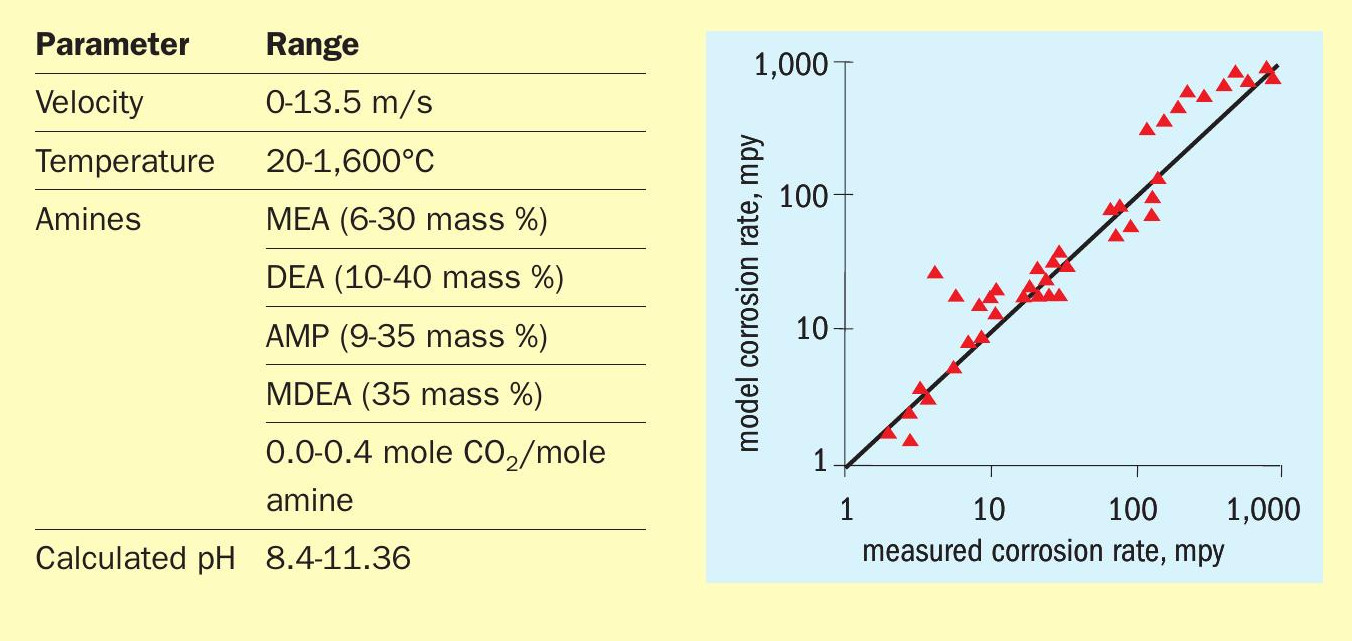
Basis for a predictive corrosion model
Fundamental or first-principles models are based on physical and chemical principles and obey nature’s laws of chemistry, physics, and thermodynamics. While harder to develop than empirical models, they are rooted in science and so tend to apply over much broader ranges. Fundamental models are also much more predictive.
This phenomenological approach has been used to create a best-in-class predictive corrosion model. Experimental corrosion rate data collected in a Joint Industry Program (JIP) were studied to quantify dependence on a variety of parameters, then integrated into a comprehensive corrosion module and married with the abilities of the ProTreat ® simulator, itself a fundamental process simulation tool. The result is a corrosion module that allows corrosion coupons to be placed at multiple locations within an acid gas removal (AGR) or sour water stripping (SWS) simulation to predict the effects of operational and design decisions on corrosion virtually anywhere in a unit. Linking empirical data to a fundamental model and embedding it into the ProTreat ® gas treating and SulphurPro ® sulphur recovery simulators gives the industry a powerful new tool that is reliable and extremely easy to use.
The corrosion rate model has been implemented within ProTreat via a Virtual Corrosion Coupon, which can be inserted anywhere in a ProTreat simulation flowsheet. Recognising the four-step oxidation process, model parameters were regressed to a large amount of H 2 S and CO 2 corrosion data collected in JIPs for both rich amine and sour water systems in a variety of metallurgies. Corrosion rate is a function of the activities (ai) of free molecular H 2 S and CO 2 , bicarbonate and bisulphide ions, temperature (T), and fluid velocity (v).
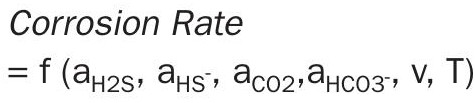
Tables 1 and 2 show the data ranges used for H 2 Sand CO 2 -only systems, respectively, together with parity plots showing predicted vs. measured corrosion rates over nearly four orders of magnitude. (Note: mpy means mils per year and 1 mil = 0.001 inch.)
Component activities rather than concentrations produced a better fit to the data. Note that although the amine type, strength and concentration are parameters that do not directly affect corrosion rates 3 (hence, they do not appear explicitly in the correlations) they certainly affect the activity of the acid gas species. In that sense, their effect is indirect. The model calculates corrosion for a straight pipe as well as for a variety of fittings and, being mechanistic, it can be reliably extrapolated to conditions outside measured ranges. The model is within a factor of two or so of the measured data. This may seem like a wide uncertainty; however, practitioners will recognise just how difficult it is to achieve great reproducibility of corrosion rate measurements, especially in commercial settings.
Case Study 1:
Treating refinery fuel gas in an amine unit
The case centres on an amine-based refinery fuel gas treater in India. The refiner wanted to reduce energy consumption and increase unit capacity by replacing DEA with MDEA. With the recent changes to BS-VI standards mandating significantly lower sulphur specifications in fuels, there was a keen desire to avoid higher H 2 S slip under increased sulphur load contributing to total sulphur emissions. The hope was that MDEA would achieve better H 2 S selectivity at lower circulation. However, there was concern that corrosion might increase so the question was whether some carbon steel piping might need to be replaced with stainless-steel or other exotic metallurgy.
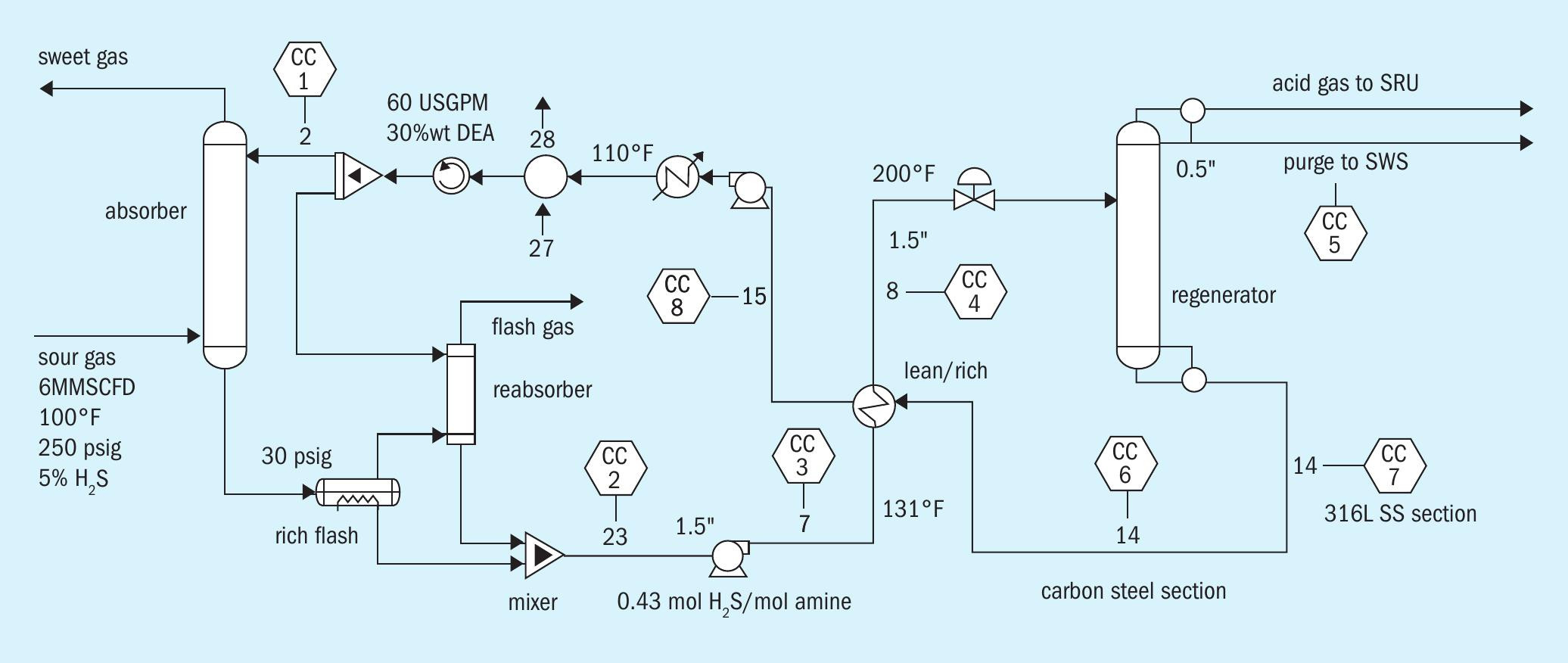
The case study was built in ProTreat ® .
Fig. 2 is a flow diagram of the refinery fuel gas treating system. Corrosion coupons are shown by the hexagonal symbols labeled CC attached to various streams. Fig. 3 shows typical corrosion coupon information that is fed into the flowsheet (left-hand side) and the corrosion rate information (right-hand side) generated as part of the general process flowsheet simulation.
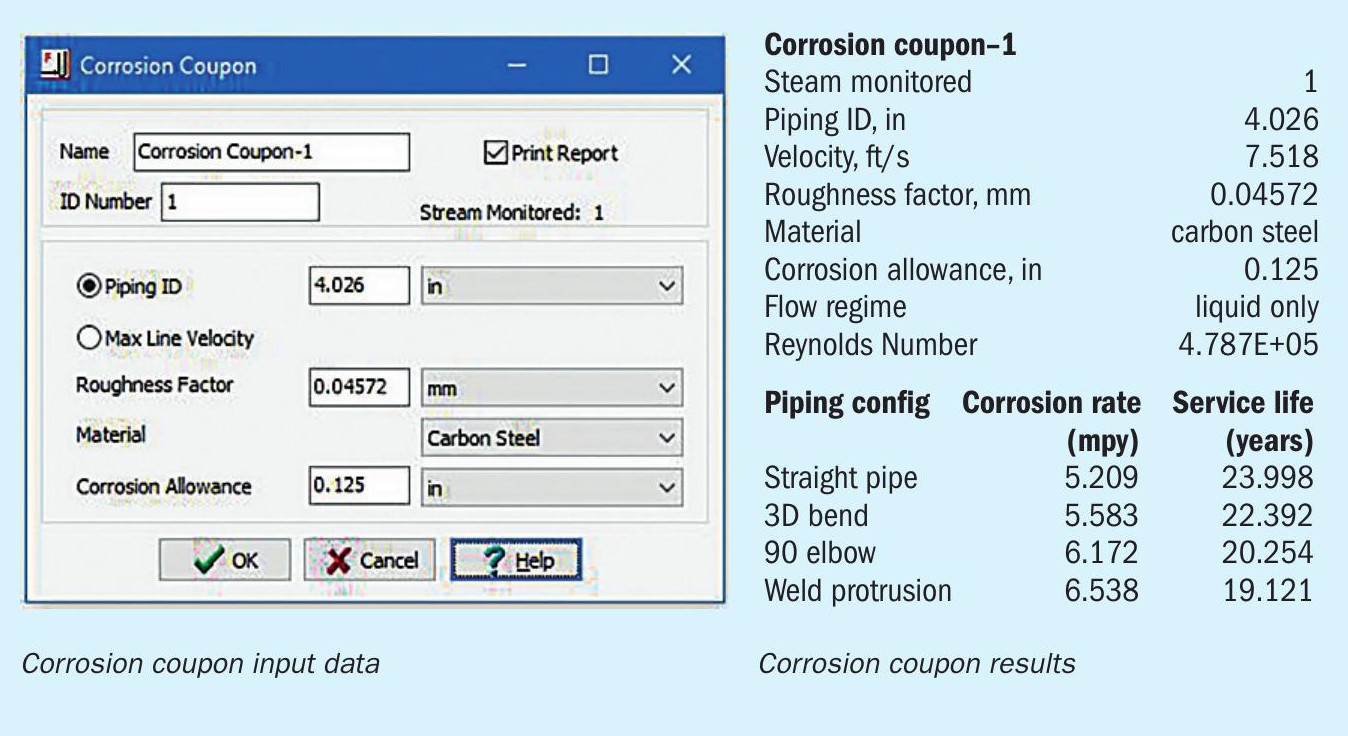
Eight corrosion coupons were inserted in various pipe sections. Three cases were considered:
- 30 mass % DEA and 60 gal/min circulation (base case)
- Case (a) switched to 45 mass % MDEA
- Case (b) with 45 mass % MDEA and 45 gal/min circulation (reboiler duty scaled to the same duty per volume of solvent as base case)
Fig. 4 compares predicted corrosion rates in various parts of the plant for the (a) 30% DEA, (b) 30% MDEA and (c) 45% MDEA cases. Note that the reboiler duty in Cases (b) and (c) was scaled to the same heat load per unit volume of solvent as Case (a), and the circulation rate of 45 US gal/min for Case (c) was designed to produce the same treated gas composition as in Case (a). The high corrosion rates experienced by Coupon 4 show the greatest sensitivity to amine type, strength, and circulation rate. Coupon 4 is in the hot rich amine line entering the regenerator. Increasing corrosion rates for Coupon 4 result from increasing concentrations of both H 2 S and HS – ion, directly caused by different amine alkalinities and strengths. Corrosion rate does not directly track with pH or solvent loading for this case study.
Based on this case study, upgrading the entire metallurgy to stainless steel was obviously unnecessary. However, the corrosion rate of the hot rich line with MDEA at 45 mass % is quite a bit higher than the base case. When the circulation of MDEA at higher strength is reduced, corrosion increases even further to the point where the service life of the piping in that area is only about four years with a corrosion allowance of 1/8th inch. Thus, a more exotic metallurgy was recommended. The piping for the hot rich and hot lean flows are always areas of concern, and the industry has migrated towards the use of austenitic stainless steel in these locations. The model supports these observations.
The corrosion coupon model can be used on sour water stripper systems as well. Similar observations have been drawn from various design and operating sour water stripper scenarios. Pertinently, the ammonia content in sour water stripper systems does not influence the corrosion. However, the ammonia content affects the speciation which in turn affects the corrosion.
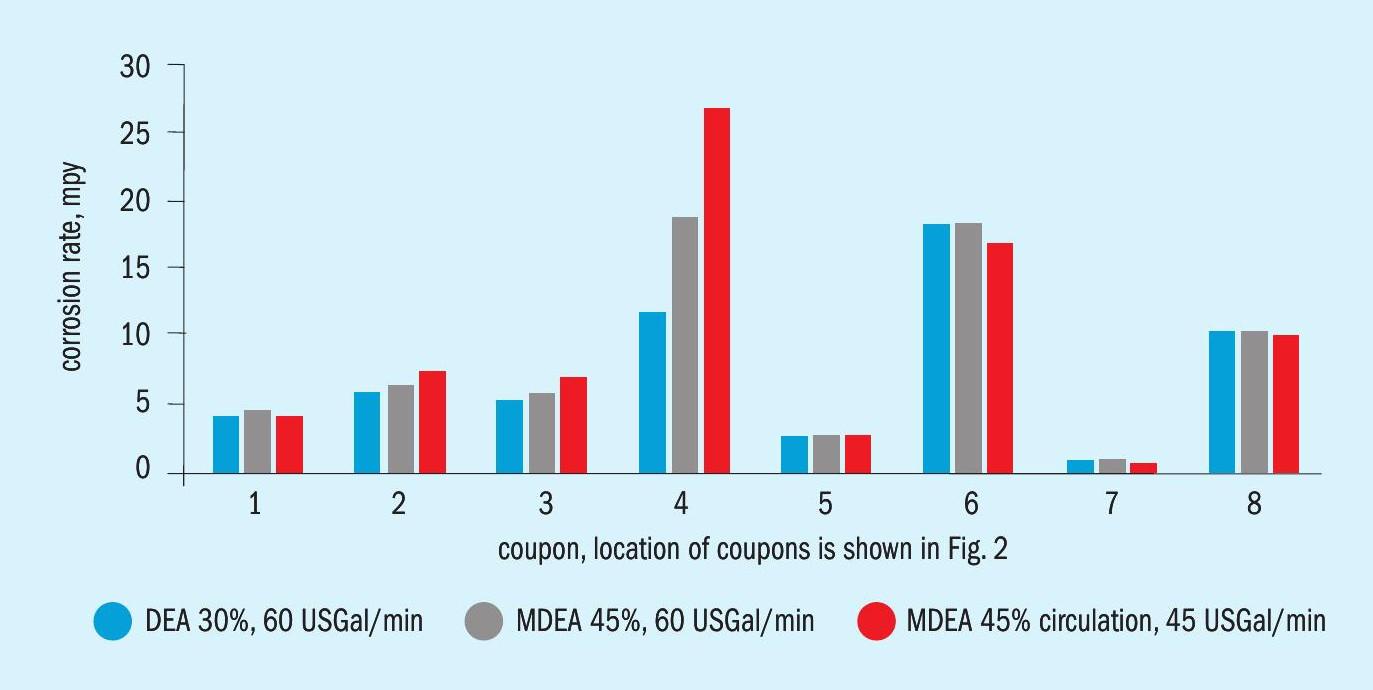
Case study 2:
SWS corrosion
Two cases were considered: a conventional overhead condenser system (Fig. 5), and a pumparound condenser configuration (Fig. 6). It is not uncommon to hear in the industry that pumparound type systems are less corrosive, so this type of system was of interest to contrast and challenge the statement. For this case study, the feed sour water contained 4 wt-% equivalent NH 4 HS. Table 3 summarises predicted corrosion rates.
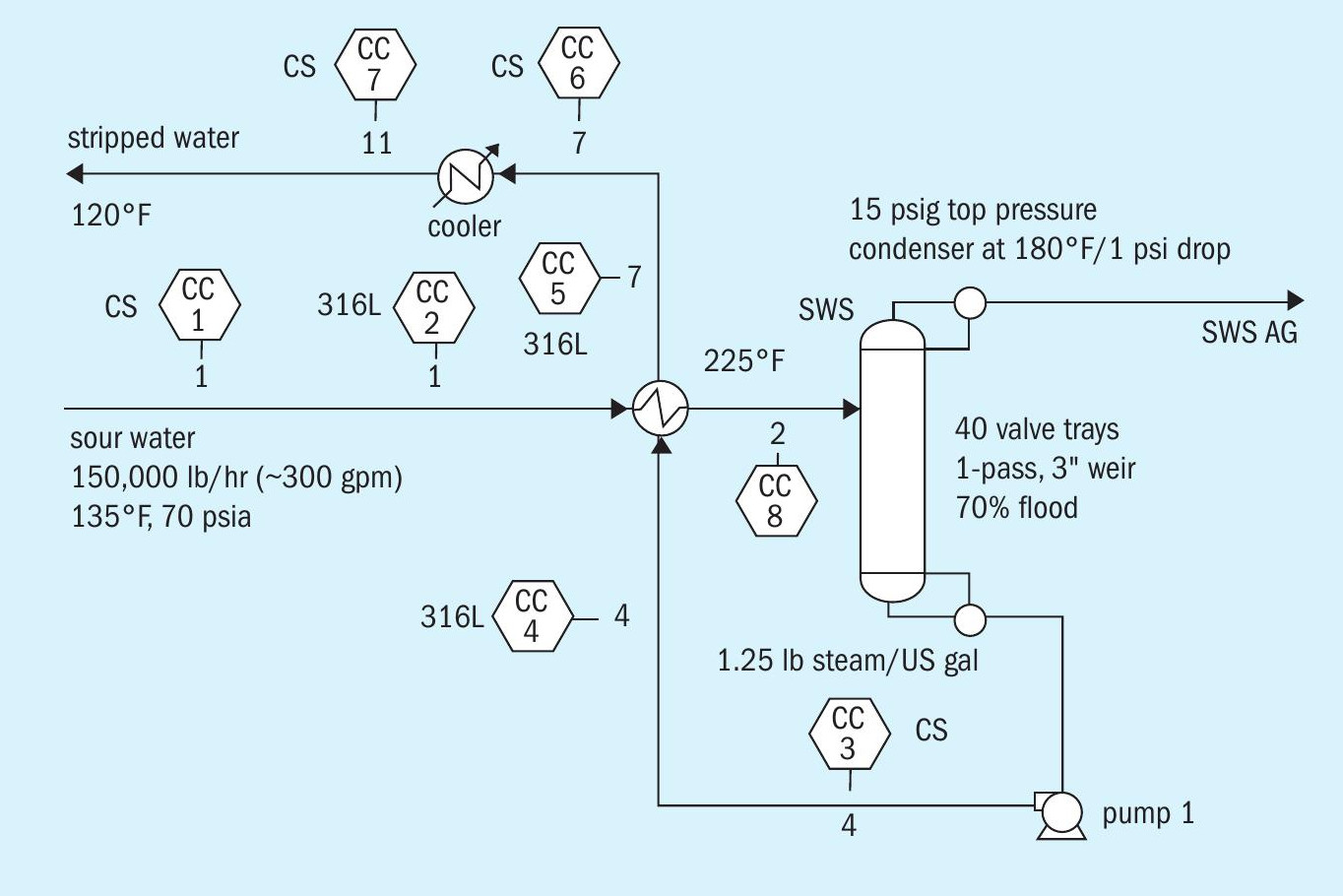
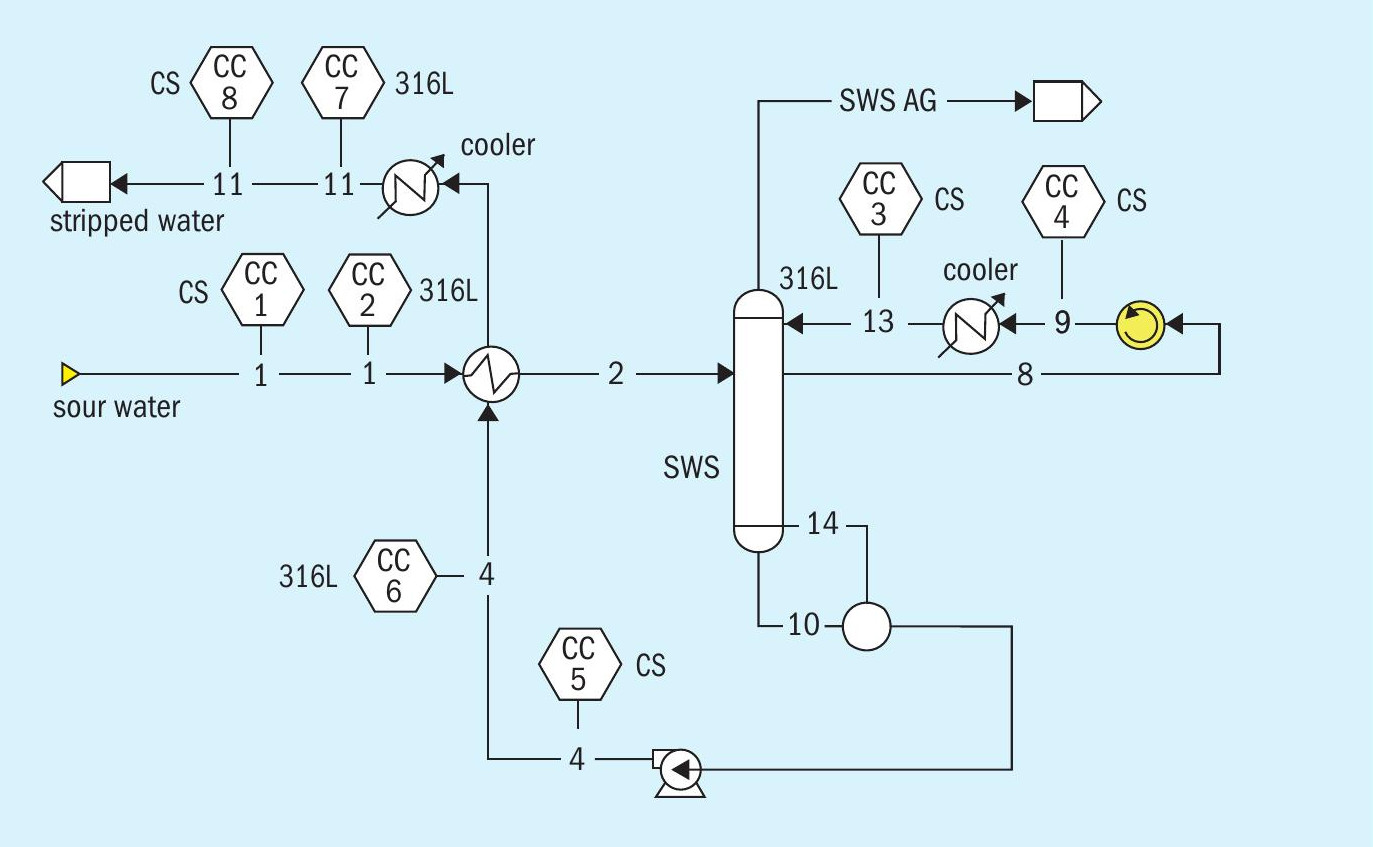
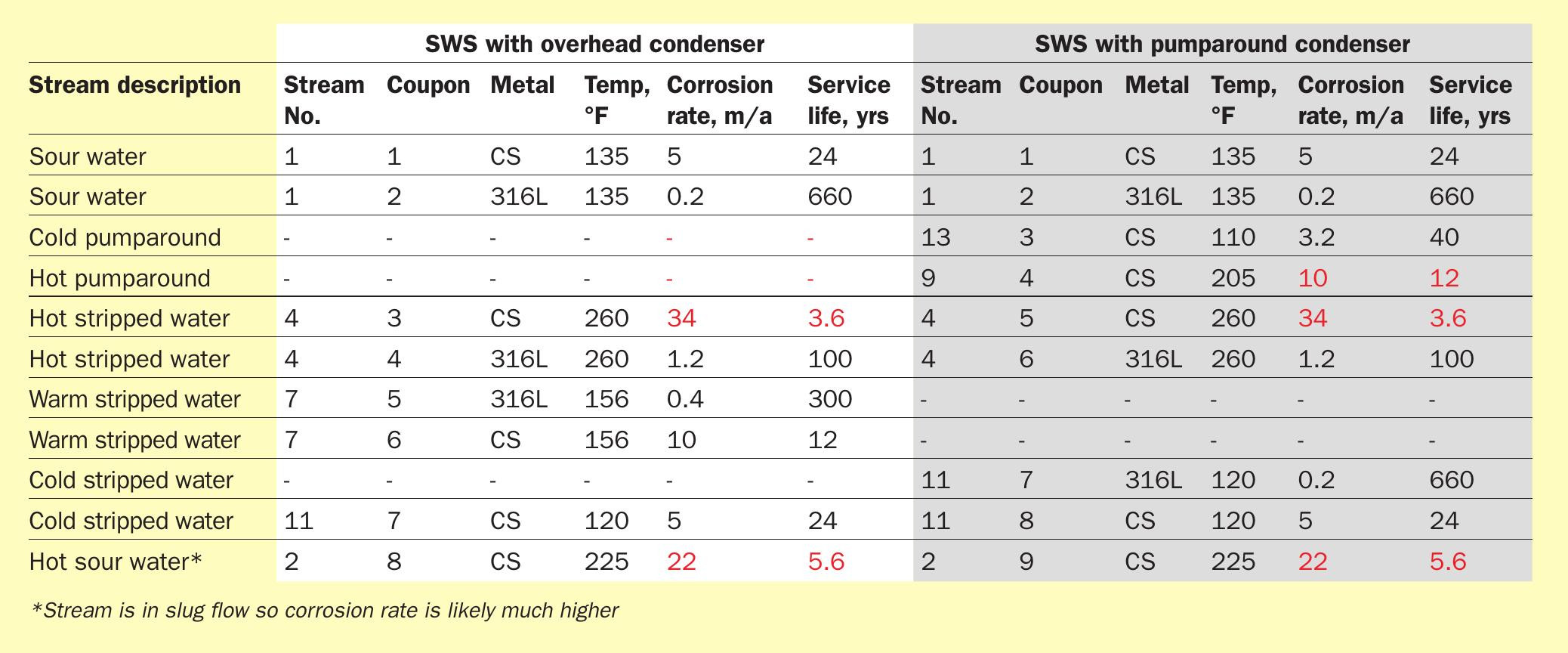
The hot sour water (Stream 2) shows a high corrosion rate as one might expect. The high temperature combined with the fact that the tower feed is partially vaporised leading to quite high velocity of a stream in slug flow will no doubt cause the corrosion rate in this line to be even higher than predicted by the model. Therefore, stainless steel (304 or 316) metallurgy would normally be recommended in this service.
Perhaps the greatest surprise is the corrosiveness of the hot stripped water. A service life of just over three years is unacceptable. The stripped water is predicted to contain 26 ppmw ammonia with 0.33 ppmw H 2 S and 0.1 ppmw CO 2 . There is not enough H 2 S to passivate the carbon steel pipe, but there is enough for unimpeded corrosion of the metal surface. At a flow velocity of 7.5 ft/s in the pipe, the very high temperature (260°F) almost guarantees maximum corrosion rate.
In the pumparound condenser configuration, the pumparound flow itself contains 7.3 wt-% ammonia with 4 wt-% H 2 S and 0.7 wt-% CO 2 . This is enough H 2 S to provide good passivation; however, high temperature causes a rather low service life of only ten years, suggesting higher metallurgy would be advantageous.
Conclusions
Neither amine type, nor amine strength, nor ammonia content have a direct effect on corrosion rate but they do have an indirect effect because of their role in the speciation of the acid gases that cause corrosion. This mechanistic, chemistry-based model built using both proprietary JIP and public corrosion rate data predicts corrosion rates. It has the added advantage of being useable directly within a process flowsheet simulation. And the model’s scientific foundation permits it to be extrapolated outside the known data ranges. Corrosion can now be assessed reliably and conveniently as an integral part of every simulation.
References